Bayesian based Re-parameterization for DNN Model Pruning
Proceedings of the 30th ACM International Conference on Multimedia(2022)
摘要
ABSTRACTFilter pruning, as an effective strategy to obtain efficient compact structures from over-parametric deep neural networks(DNN), has attracted a lot of attention. Previous pruning methods select channels for pruning by developing different criteria, yet little attention has been devoted to whether these criteria can represent correlations between channels. Meanwhile, most existing methods generally ignore the parameters being pruned and only perform additional training on the retained network to reduce accuracy loss. In this paper, we present a novel perspective of re-parametric pruning by Bayesian estimation. First, we estimate the probability distribution of different channels based on Bayesian estimation and indicate the importance of the channels by the discrepancy in the distribution before and after channel pruning. Second, to minimize the variation in distribution after pruning, we re-parameterize the pruned network based on the probability distribution to pursue optimal pruning. We evaluate our approach on popular datasets with some typical network architectures, and comprehensive experimental results validate that this method illustrates better performance compared to the state-of-the-art approaches.
更多查看译文
关键词
bayesian,re-parameterization
AI 理解论文
溯源树
样例
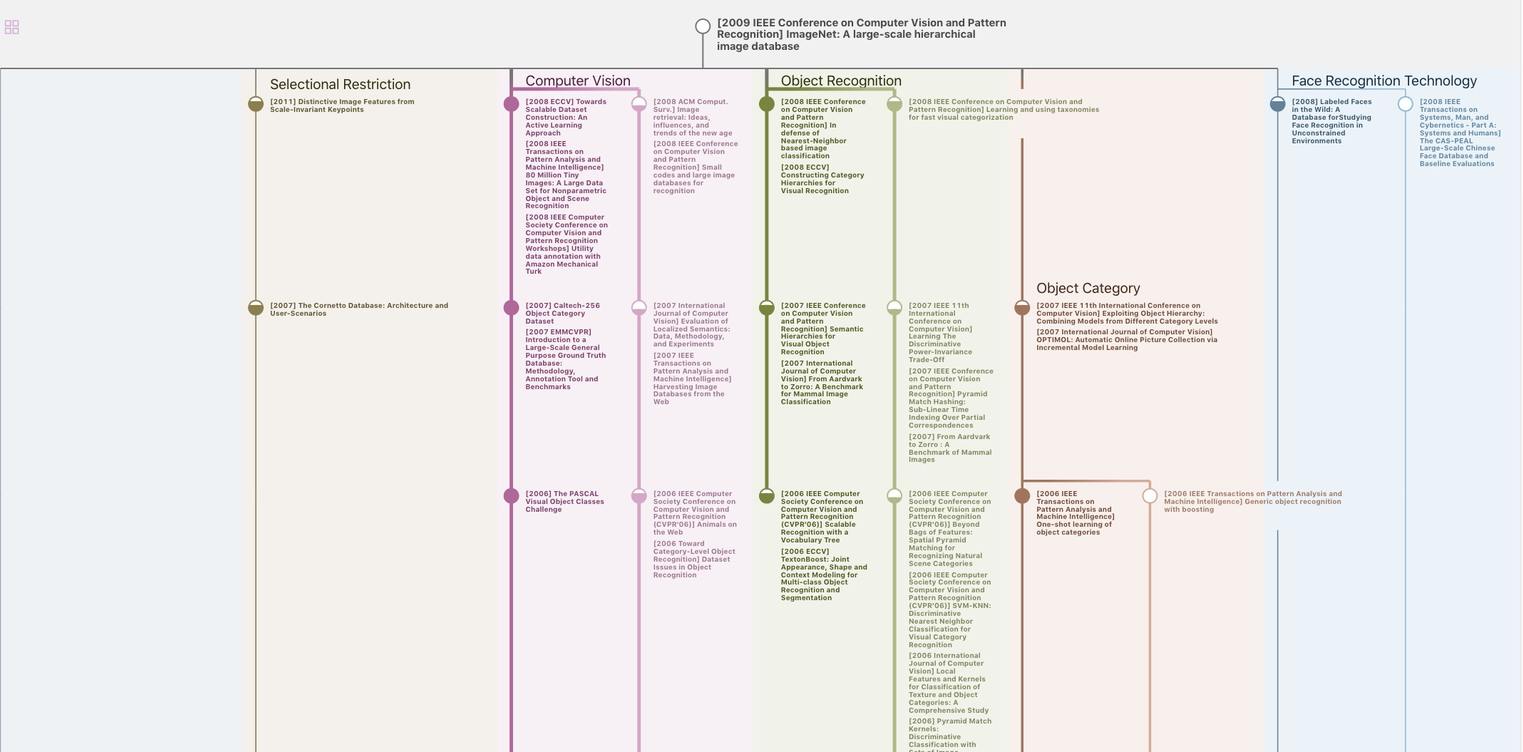
生成溯源树,研究论文发展脉络
Chat Paper
正在生成论文摘要