Contrastive Consensus Graph Learning for Multi-View Clustering
IEEE/CAA Journal of Automatica Sinica(2022)
摘要
Dear Editor, This letter proposes a contrastive consensus graph learning model for multi-view clustering. Graphs are usually built to outline the correlation between multi-model objects in clustering task, and multiview graph clustering aims to learn a consensus graph that integrates the spatial property of each view. Nevertheless, most graph-based models merely consider the overall structure from all views but neglect the local spatial consistency between diverse views, resulting in the lack of global spatial consistency in the learned graph. To overcome this issue, a deep convolutional network is built to explore latent local spatial information from raw affinity graphs. Specifically, we employ a consensus graph constraint to preserve the global consistency between the learned graph and raw graphs. Furthermore, a contrastive reconstruction loss is introduced to achieve the sample-level approximation between reconstructed graphs and raw graphs, which facilitates the network to enhance the consensus graph learning. Experiments on six classical datasets demonstrate that the proposed model outperforms other nine state-of-the-art algorithms.
更多查看译文
AI 理解论文
溯源树
样例
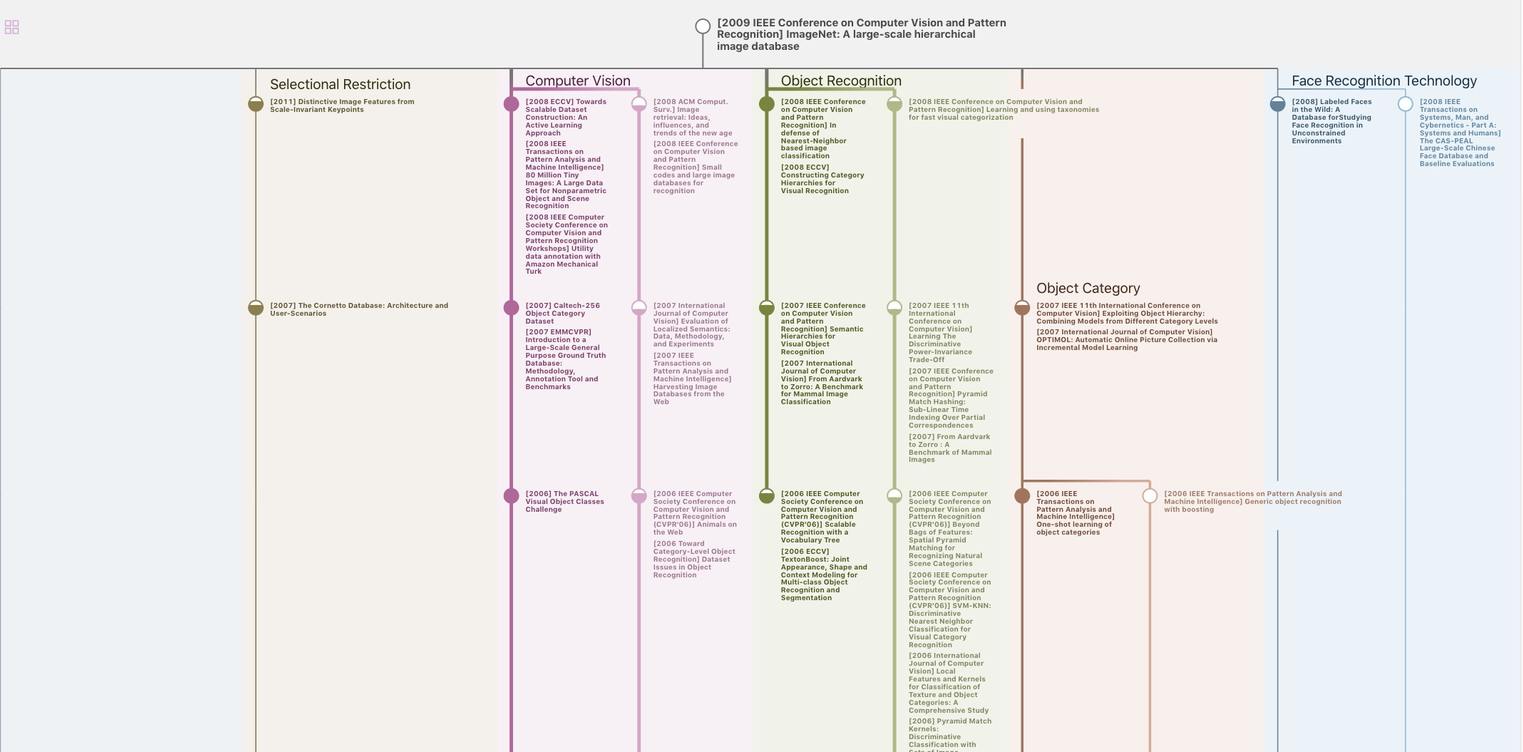
生成溯源树,研究论文发展脉络
Chat Paper
正在生成论文摘要