Neighborhood-Adaptive Multi-cluster Ranking for Deep Metric Learning
IEEE Transactions on Circuits and Systems for Video Technology(2022)
Abstract
Deep metric learning methods generally concentrate on designing distance-based losses to learn sample embeddings, which tacitly presuppose the neighborhood structure around each sample (e.g., hypersphere for Euclidean distance). However, this supposition is overly optimistic: 1) visual data is often located on low-dimensional manifolds curved in high-dimensional space, and all regions of the manifold may hardly share the same local structures in the input space; 2) it is unlikely that the local structure in the output embedding space is as homogeneous as assumed due to the non-linearity of neural networks. Hence, simply characterizing sample embeddings while ignoring the respective neighborhood structures leads to limitations. To address this problem, this paper presents a Neighborhood-Adaptive Multi-cluster Ranking (NAMR) framework by leveraging the heterogeneity of local structures. Specifically, considering that indexing algorithms are usually required in large-scale retrieval, NAMR characterizes an image from two kinds of embeddings (i.e., sample embedding and structure embedding). The sample embedding can be trained using any distance-based loss, while the structure embedding representing the neighborhood structure can be jointly learned with the sample embedding in a self-supervised multi-cluster ranking manner. In this way, existing indexing algorithms can seamlessly support large-scale retrieval employing NAMR embeddings without any modifications. We evaluate the proposed model on five standard benchmarks, consistently and explicitly improving four baselines (especially the simplest triplet loss) and achieving state-of-the-art performance.
MoreTranslated text
Key words
Neighborhood-adaptive,multi-cluster ranking,metric learning,self-supervised learning
AI Read Science
Must-Reading Tree
Example
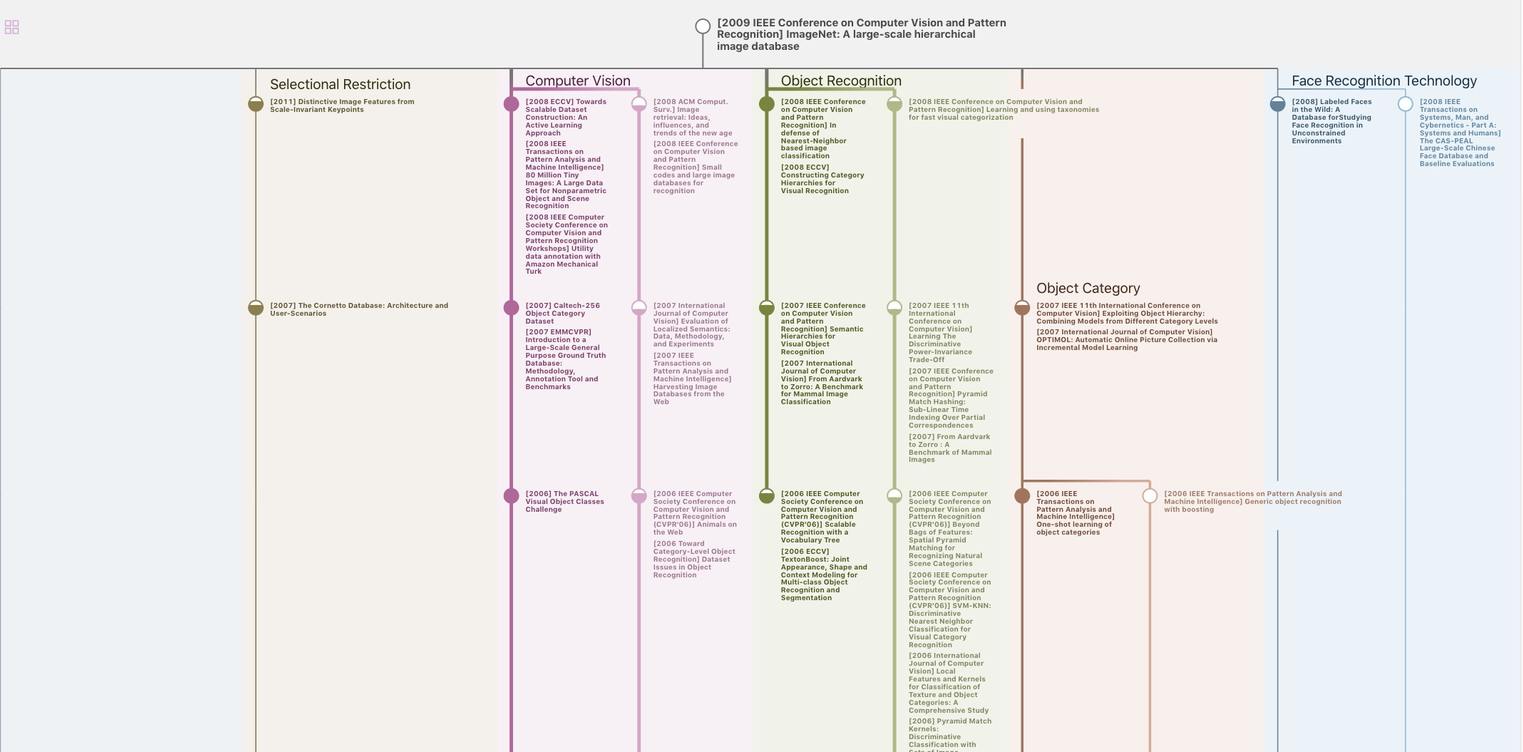
Generate MRT to find the research sequence of this paper
Chat Paper
Summary is being generated by the instructions you defined