Machine learning-based mix design tools to minimize carbon footprint and cost of UHPC. Part 1: Efficient data collection and modeling
Cleaner Materials(2022)
Abstract
The emergence of ultra-high-performance concrete (UHPC) as an attractive solution for precast and prestressed applications has coincided with global efforts towards sustainable construction. Tools capable of intuitively demonstrating the tradeoffs between mix proportions and mechanical performance are in increasing need for this industry given the embodied carbon footprint associated with typical UHPC compositions. Meanwhile, the ingredients and their proportions required to produce UHPC synergistically dictate material performance. This makes linear regression ineffective for optimization purposes, while presenting a great opportunity for artificial intelligence (AI) techniques. Yet, the effectiveness of AI models is highly dependent on the size, distribution, and quality of the data. This has led researchers to use datasets from multiple sources to develop their models in lieu of performing extensive experimental runs, which can become exhausting, time-consuming and resource-intensive. This study presents a novel and efficient methodology to optimize mixture design of UHPC while performing reduced experimental runs, allowing concrete producers to quickly generate efficient models using their own data and materials. In this work (Part 1), orthogonal arrays are utilized to collect data needed to enable machine learning (ML) design optimization. ML techniques in random forest and k-nearest neighbors are ensembled to generate Performance Density Diagrams (PDDs), an innovative mix design tool developed to facilitate decision-making by designers, where changes in strength associated with changes in mix proportioning can easily be evaluated by industry personnel. Results indicate that the generated PDDs effectively predicted the trends, magnitude and ranking of most mixtures stored in a test set. Moreover, PDDs enabled the design of an UHPC mixture averaging 155 MPa at age 56 days, while maintaining the fine-aggregate-to-cementitious ratio above unit. This methodology is later extended in “Part 2” of this research, where predicted strengths from the model developed herein are used as inputs to calculate multi-objective comparison indices that allow performance, cost and carbon footprint to be evaluated concurrently..
MoreTranslated text
Key words
UHPC,Artificial Intelligence,Machine Learning,Optimization,Mix Design,Performance Density Diagrams
AI Read Science
Must-Reading Tree
Example
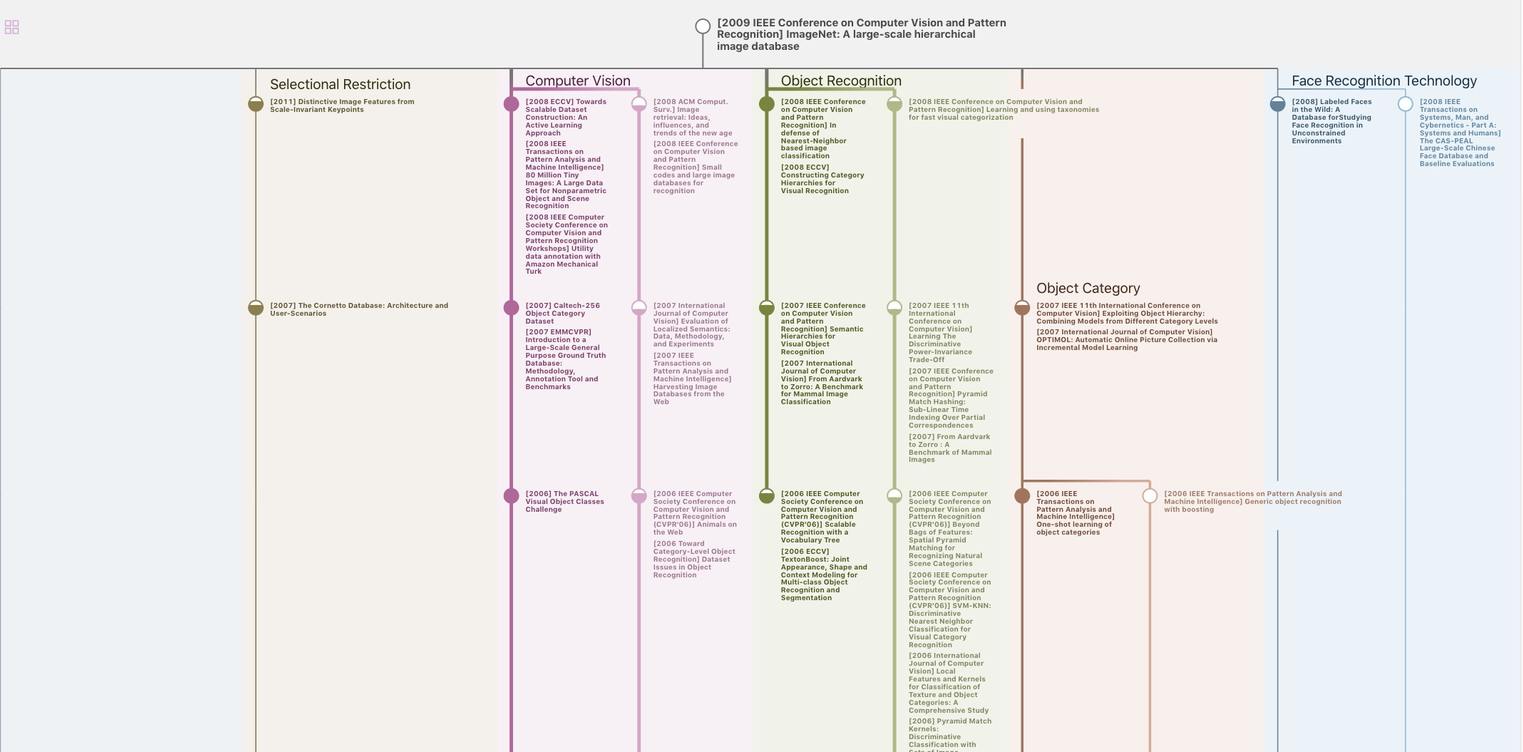
Generate MRT to find the research sequence of this paper
Chat Paper
Summary is being generated by the instructions you defined