A Survey of Methods for Addressing Class Imbalance in Deep-Learning Based Natural Language Processing
arxiv(2023)
摘要
Many natural language processing (NLP) tasks are naturally imbalanced, as some target categories occur much more frequently than others in the real world. In such scenarios, current NLP models still tend to perform poorly on less frequent classes. Addressing class imbalance in NLP is an active research topic, yet, finding a good approach for a particular task and imbalance scenario is difficult. With this survey, the first overview on class imbalance in deep-learning based NLP, we provide guidance for NLP researchers and practitioners dealing with imbalanced data. We first discuss various types of controlled and real-world class imbalance. Our survey then covers approaches that have been explicitly proposed for class-imbalanced NLP tasks or, originating in the computer vision community, have been evaluated on them. We organize the methods by whether they are based on sampling, data augmentation, choice of loss function, staged learning, or model design. Finally, we discuss open problems such as dealing with multi-label scenarios, and propose systematic benchmarking and reporting in order to move forward on this problem as a community.
更多查看译文
关键词
addressing class imbalance,deep-learning
AI 理解论文
溯源树
样例
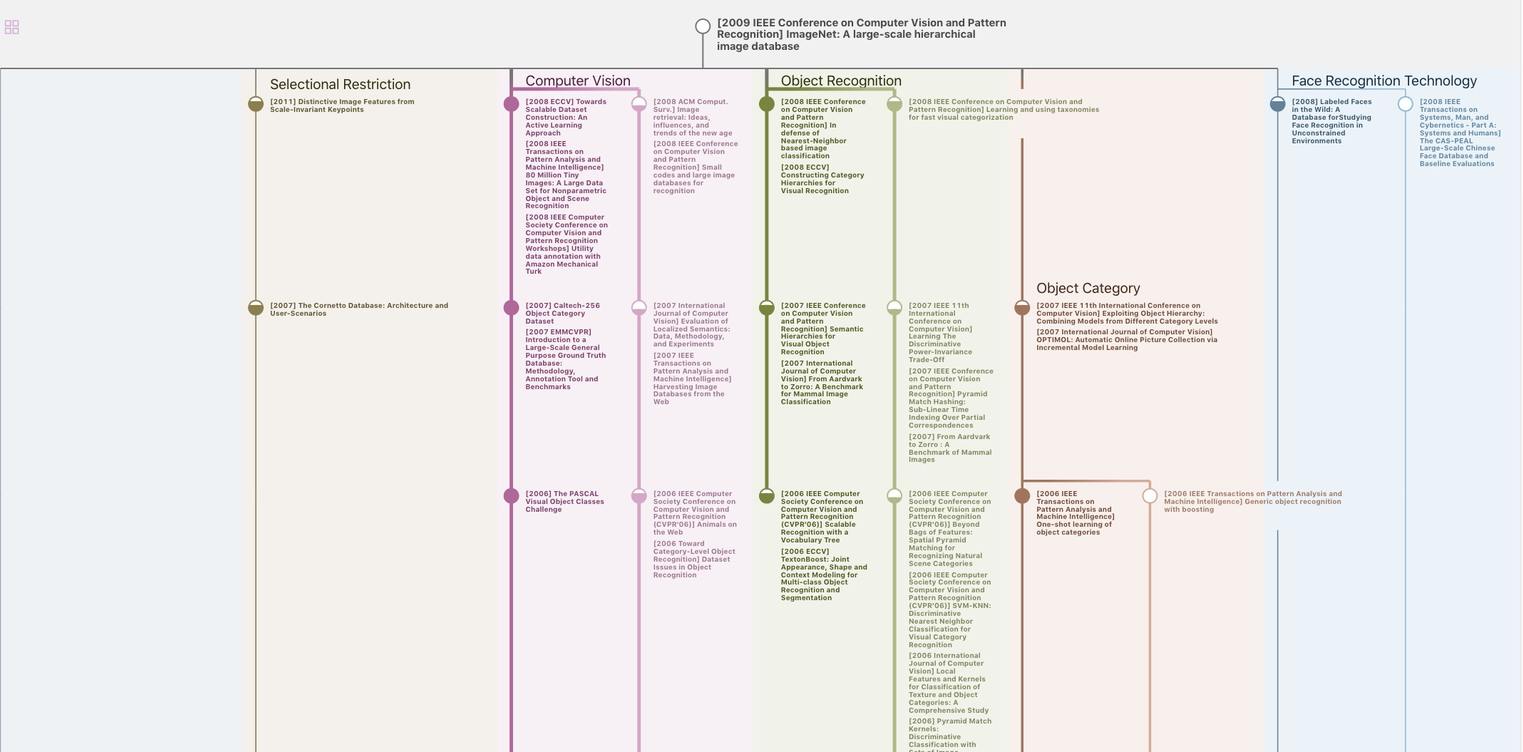
生成溯源树,研究论文发展脉络
Chat Paper
正在生成论文摘要