Parameter-Efficient Tuning with Special Token Adaptation
17TH CONFERENCE OF THE EUROPEAN CHAPTER OF THE ASSOCIATION FOR COMPUTATIONAL LINGUISTICS, EACL 2023(2023)
Abstract
Parameter-efficient tuning aims at updating only a small subset of parameters when adapting a pretrained model to downstream tasks. In this work, we introduce PASTA, in which we only modify the special token representations (e.g., [SEP] and [CLS] in BERT) before the self-attention module at each layer in Transformer-based models. PASTA achieves comparable performance to full finetuning in natural language understanding tasks including text classification and NER with up to only 0.029% of total parameters trained. Our work not only provides a simple yet effective way of parameter-efficient tuning, which has a wide range of practical applications when deploying finetuned models for multiple tasks, but also demonstrates the pivotal role of special tokens in pretrained language models.
MoreTranslated text
Key words
special token adaptation,tuning,parameter-efficient
AI Read Science
Must-Reading Tree
Example
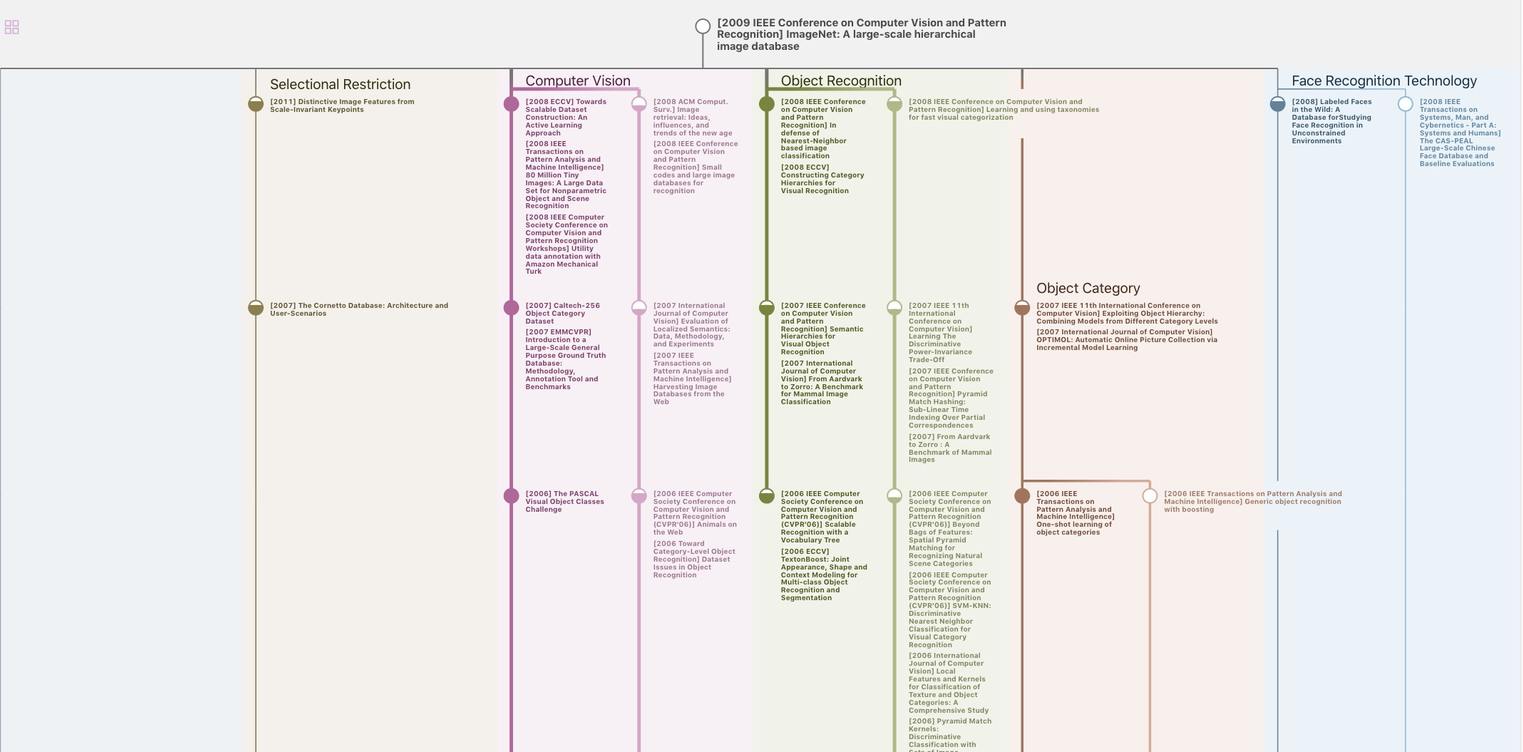
Generate MRT to find the research sequence of this paper
Chat Paper
Summary is being generated by the instructions you defined