A Survey on Extreme Multi-label Learning
arxiv(2022)
摘要
Multi-label learning has attracted significant attention from both academic and industry field in recent decades. Although existing multi-label learning algorithms achieved good performance in various tasks, they implicitly assume the size of target label space is not huge, which can be restrictive for real-world scenarios. Moreover, it is infeasible to directly adapt them to extremely large label space because of the compute and memory overhead. Therefore, eXtreme Multi-label Learning (XML) is becoming an important task and many effective approaches are proposed. To fully understand XML, we conduct a survey study in this paper. We first clarify a formal definition for XML from the perspective of supervised learning. Then, based on different model architectures and challenges of the problem, we provide a thorough discussion of the advantages and disadvantages of each category of methods. For the benefit of conducting empirical studies, we collect abundant resources regarding XML, including code implementations, and useful tools. Lastly, we propose possible research directions in XML, such as new evaluation metrics, the tail label problem, and weakly supervised XML.
更多查看译文
关键词
multi-label
AI 理解论文
溯源树
样例
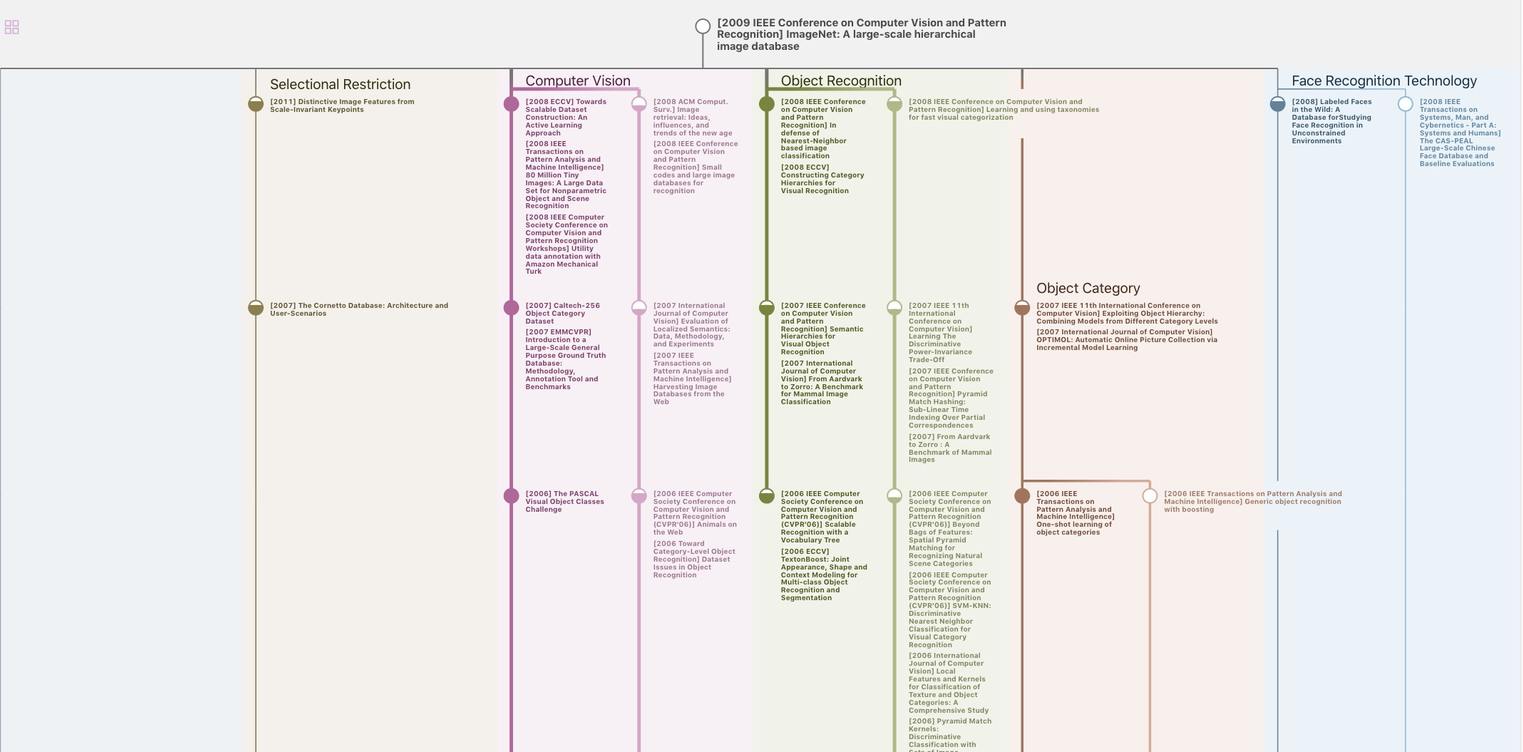
生成溯源树,研究论文发展脉络
Chat Paper
正在生成论文摘要