Accelerated and Deep Expectation Maximization for One-Bit MIMO-OFDM Detection
IEEE TRANSACTIONS ON SIGNAL PROCESSING(2024)
摘要
In this paper we study the expectation maximization (EM) technique for one-bit MIMO-OFDM detection (OMOD). Arising from the recent interest in massive MIMO with one-bit analog-to-digital converters, OMOD is a massive-scale problem. EM is an iterative method that can exploit the OFDM structure to process the problem in a per-iteration efficient fashion. In this study we analyze the convergence rate of EM for a class of approximate maximum-likelihood OMOD formulations, or, in a broader sense, a class of problems involving regression from quantized data. We show how the SNR and channel conditions can have an impact on the convergence rate. We do so by making a connection between the EM and the proximal gradient methods in the context of OMOD. This connection also gives us insight to build new accelerated and/or inexact EM schemes. The accelerated scheme has faster convergence in theory, and the inexact scheme provides us with the flexibility to implement EM more efficiently, with convergence guarantee. Furthermore we develop a deep EM algorithm, wherein we take the structure of our inexact EM algorithm and apply deep unfolding to train an efficient structured deep net. Simulation results show that our accelerated exact/inexact EM algorithms run much faster than their standard EM counterparts, and that the deep EM algorithm gives promising detection and runtime performances.
更多查看译文
关键词
One-bit MIMO-OFDM detection,expectation maximization,convergence analysis,deep unfolding
AI 理解论文
溯源树
样例
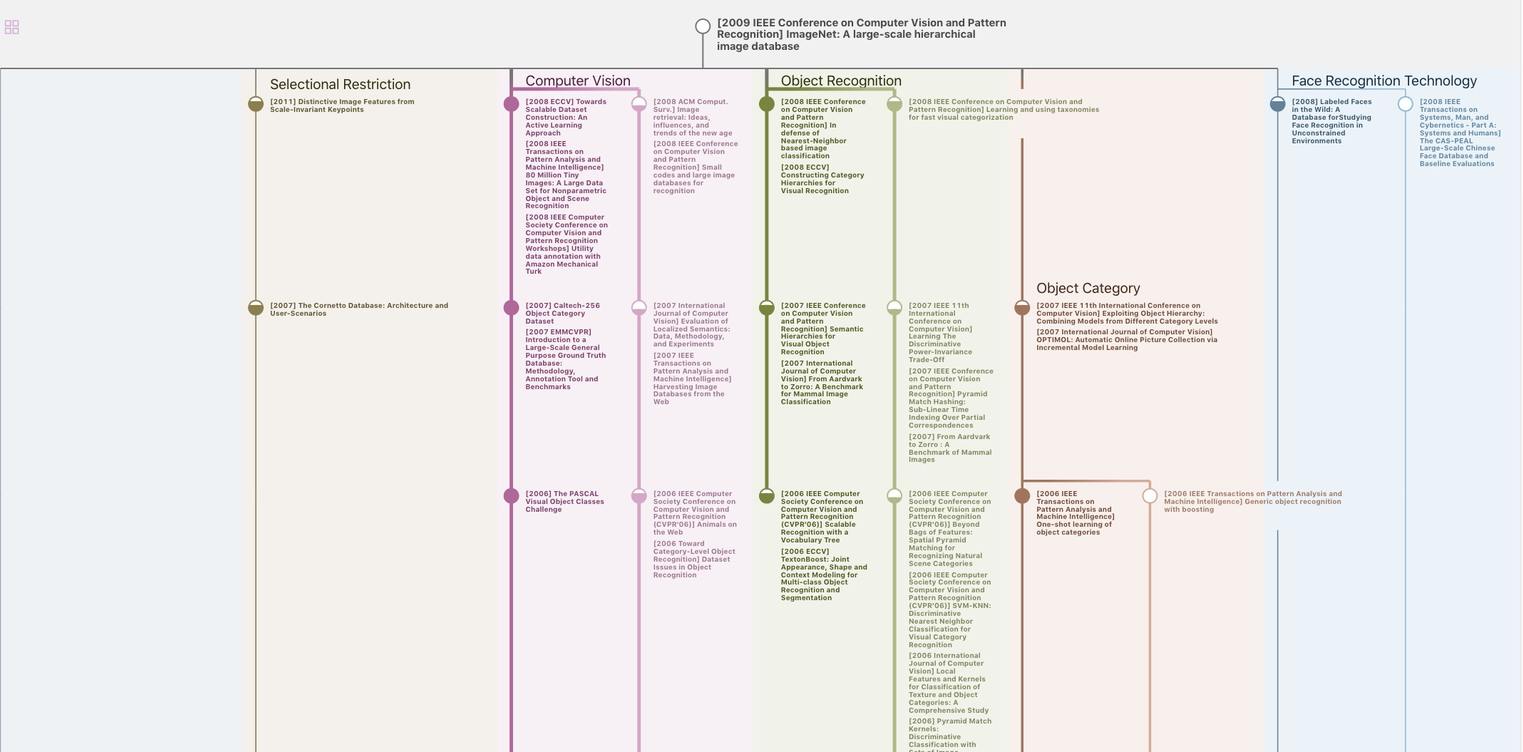
生成溯源树,研究论文发展脉络
Chat Paper
正在生成论文摘要