Self-Aligned Concave Curve: Illumination Enhancement for Unsupervised Adaptation
International Multimedia Conference(2022)
摘要
ABSTRACTLow light conditions not only degrade human visual experience, but also reduce the performance of downstream machine analytics. Although many works have been designed for low-light enhancement or domain adaptive machine analytics, the former considers less on high-level vision, while the latter neglects the potential of image-level signal adjustment. How to restore underexposed images/videos from the perspective of machine vision has long been overlooked. In this paper, we are the first to propose a learnable illumination enhancement model for high-level vision. Inspired by real camera response functions, we assume that the illumination enhancement function should be a concave curve, and propose to satisfy this concavity through discrete integral. With the intention of adapting illumination from the perspective of machine vision without task-specific annotated data, we design an asymmetric cross-domain self-supervised training strategy. Our model architecture and training designs mutually benefit each other, forming a powerful unsupervised normal-to-low light adaptation framework. Comprehensive experiments demonstrate that our method surpasses existing low-light enhancement and adaptation methods and shows superior generalization on various low-light vision tasks, including classification, detection, action recognition, and optical flow estimation. All of our data, code, and results will be available online upon publication of the paper.
更多查看译文
关键词
illumination enhancement,adaptation,concave curve,self-aligned
AI 理解论文
溯源树
样例
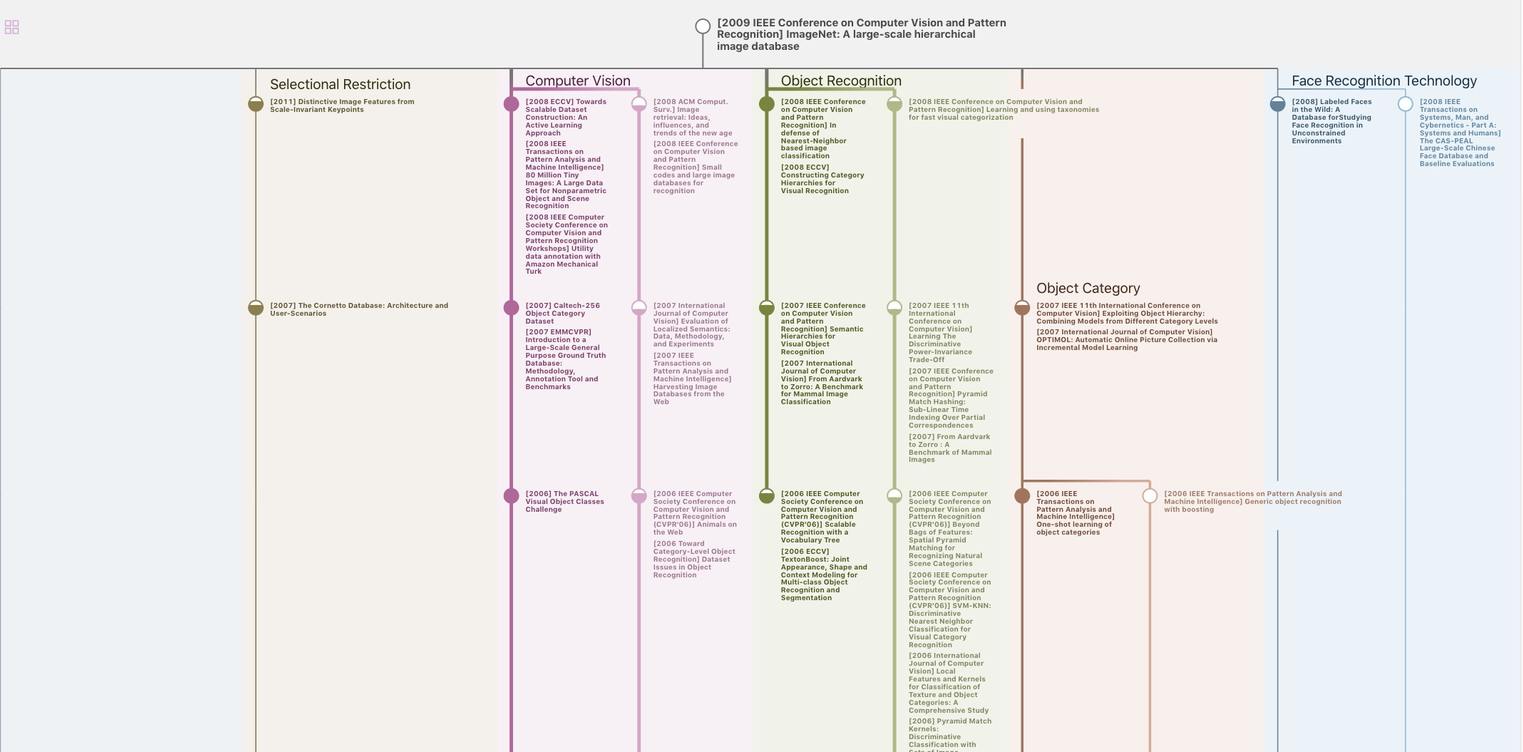
生成溯源树,研究论文发展脉络
Chat Paper
正在生成论文摘要