A multi-scale multi-model deep neural network via ensemble strategy on high-throughput microscopy image for protein subcellular localization
Expert Systems with Applications(2023)
摘要
Protein subcellular locations are closely related to the function of proteins. By detecting the abnormalities in subcellular locations, we can infer the occurrence of some diseases and mine new drug targets. A large number of single-cell high-throughput microscopy images provide us with relevant resources for studying protein distribution patterns. However, in the existing image-based protein subcellular localization methods, the traditional techniques are lack of efficiency and accuracy, and the potential of deep learning methods has not been fully tapped. So in this study, we propose a multi-scale multi-model deep neural network via ensemble strategy for protein subcellular localization on single-cell high-throughput images. First of all, we employ a deep convolutional neural network as multi-scale feature extractor and use global average pooling to map extracted features at different stages into feature vectors, then concatenate these multi-scale features to form a multi-model structure for image classification. In addition, we add Squeeze-and-Excitation Blocks to the network to emphasize more informative features. What is more, we use an ensemble method to fuse the classification results from the multi-model structure to obtain the final subcellular location of each single-cell image. Experiments show the validity and effectiveness of our method on yeast cell images, it can significantly improve the accuracy of high-throughput microscopy image-based protein subcellular localization, and we achieve the classification accuracy of 0.9098 on the high-throughput microscopy images of yeast cells. In the work of protein subcellular localization, our method provides a framework for processing and classifying microscope images, and further lays the foundation for the study of protein and gene functions.
更多查看译文
关键词
00-01,99-00
AI 理解论文
溯源树
样例
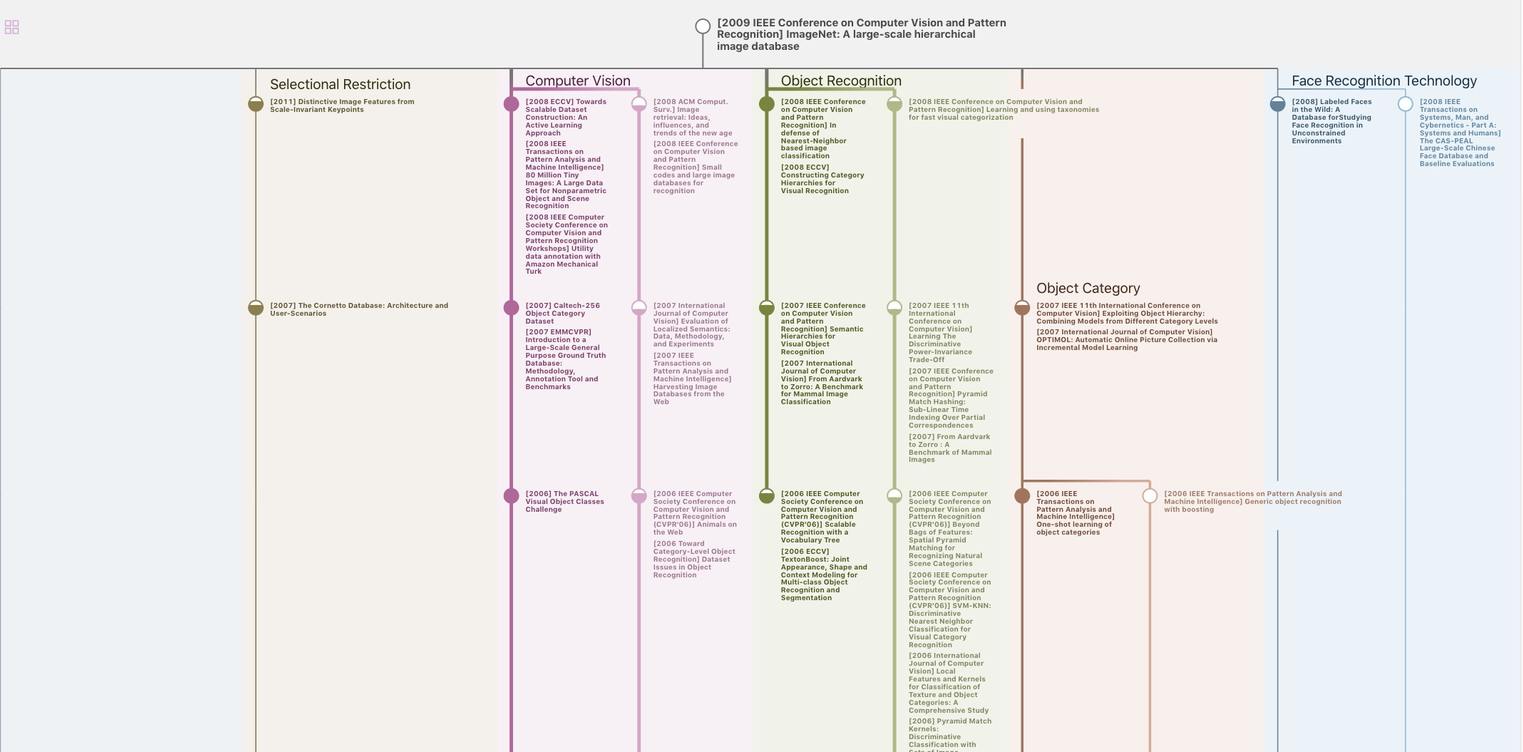
生成溯源树,研究论文发展脉络
Chat Paper
正在生成论文摘要