Instance difficulty-based noise correction for crowdsourcing
Expert Systems with Applications(2023)
摘要
Crowdsourcing offers an efficient way to obtain a multiple noisy label set of each instance from different crowd workers and then label integration algorithms are used to infer its integrated label. In spite of the effectiveness of label integration algorithms, there always exists a certain degree of noise in the integrated labels, and thus noise correction algorithms have been proposed to reduce the effect of noise. However, existing noise correction algorithms seldom consider the effect of instance difficulty on noise correction. In this paper, we argue that the greater the difficulty of an instance, the fewer crowd workers can label it correctly, and the more likely the instance is a noise instance. Based on this premise, we propose a simple but very effective noise correction algorithm called instance difficulty-based noise correction (IDNC). In IDNC, we at first propose two methods to measure the difficulty of each instance. Then, we use the proposed two methods to filter the noise instances to obtain a clean set and a noise set. Finally, we build two different classifiers on the clean set to correct the noise instances in the noise set via the consensus voting. The extensive experiments on both simulated and real-world crowdsourced datasets validated the effectiveness and efficiency of our proposed IDNC.
更多查看译文
关键词
Crowdsourcing learning,Noise correction,Instance difficulty
AI 理解论文
溯源树
样例
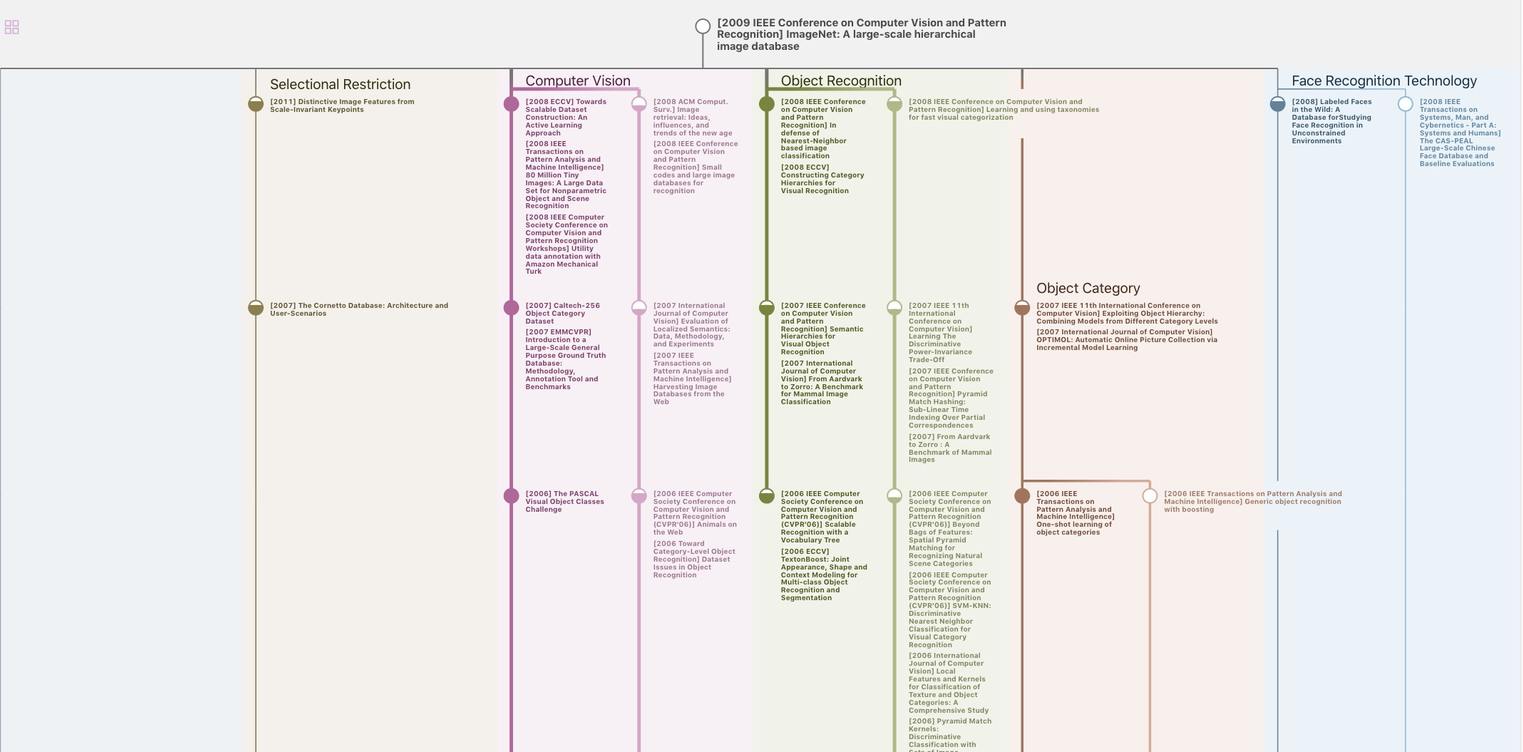
生成溯源树,研究论文发展脉络
Chat Paper
正在生成论文摘要