Feeling Without Sharing
Proceedings of the 30th ACM International Conference on Multimedia(2022)
摘要
The explosion of video data brings new opportunities and challenges for emotion recognition. Video emotion applications have great commercial value, but the potential to involve illegal snooping on personal feelings has led to controversy over privacy protection. The federated learning (FL) paradigm can substantially address the growing public concerns about data privacy in video emotion recognition. However, conventional FL methods perform poorly due to the uniqueness of the task: the data are heterogeneous across clients induced by emotional label skew and cross-culture expression differences. To mitigate the heterogeneous data, we propose EmoFed, a practical framework of federated learning video-based emotion recognition via multi-group clustering and privacy-agnostic hybrid aggregation. It yields a generically applicable and improved model while protecting privacy, which trains local models under group-aware personalized aggregation. To further encourage communicating comprehensive and privacy-agnostic information among clients, we upload model parameters of both the global layers and personalization layers to the server. We utilize the homomorphically encrypted method for personalization layers, which incurs no learning accuracy loss since no noise is added to the model updates during the encryption/decryption process. The proposed method works on video-based emotion recognition tasks to predict actors' emotional expressions and induced emotion by viewers. Extensive experiments and ablation studies on four benchmarks have demonstrated the efficacy and practicability of our method.
更多查看译文
关键词
sharing,feeling
AI 理解论文
溯源树
样例
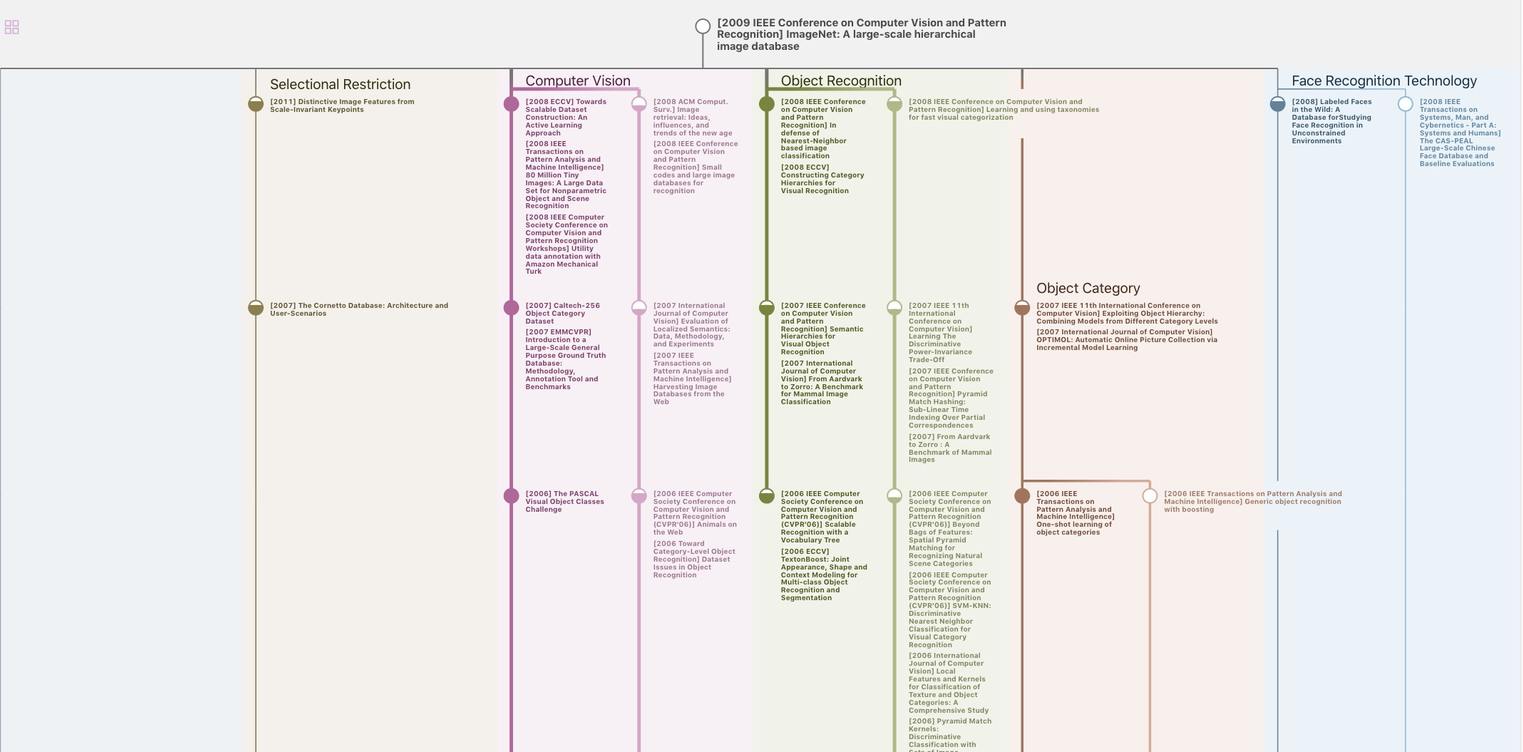
生成溯源树,研究论文发展脉络
Chat Paper
正在生成论文摘要