Explainable machine learning rapid approach to evaluate coal ash content based on X-ray fluorescence
Fuel(2023)
摘要
As one of the most important indexes of coal quality, accurate and rapid prediction of ash content is urgent and important significance for the coal processing industry. In this work, combined with Shapley Additive exPla-nations (SHAP), a machine learning model has been developed to predict the ash content of coal samples based on composition data of XRF analysis. Among the many supervised regression learning algorithms, Poly, RFR, XGBoost, and DNN are used in this predictive model to overcome the ash content prediction research gap. The input parameters were the elements content and ash contents of the coal samples. To evaluate the proposed method, a dataset of XRF data was constructed, containing 217 sets of element content with different ash content labels. Specifically, the dataset is divided into a training set and test set in the proportion 8:2, and Random-izedSearchCV is used to optimize hyperparameters during model training. Experimental results show that the RFR model produced a superior prediction performance over other models (the RMSE, MAE and R2 were 1.3278, 0.9339 and 0.9916, respectively). The contribution and role of each element to the ash prediction model are explained and investigated. Moreover, as a result of SHAP interpretation, the nine most important elements (Al, S, Si, Fe, Ca, Ti, K, Sr and Zr) has the greatest contribution to model performance. The case of this paper suggests that interpreted machine learning models and XRF data are good alternatives to ash content prediction.
更多查看译文
关键词
Ash content,X-ray fluorescence,Random forest Regression,SHAP,Coal preparation
AI 理解论文
溯源树
样例
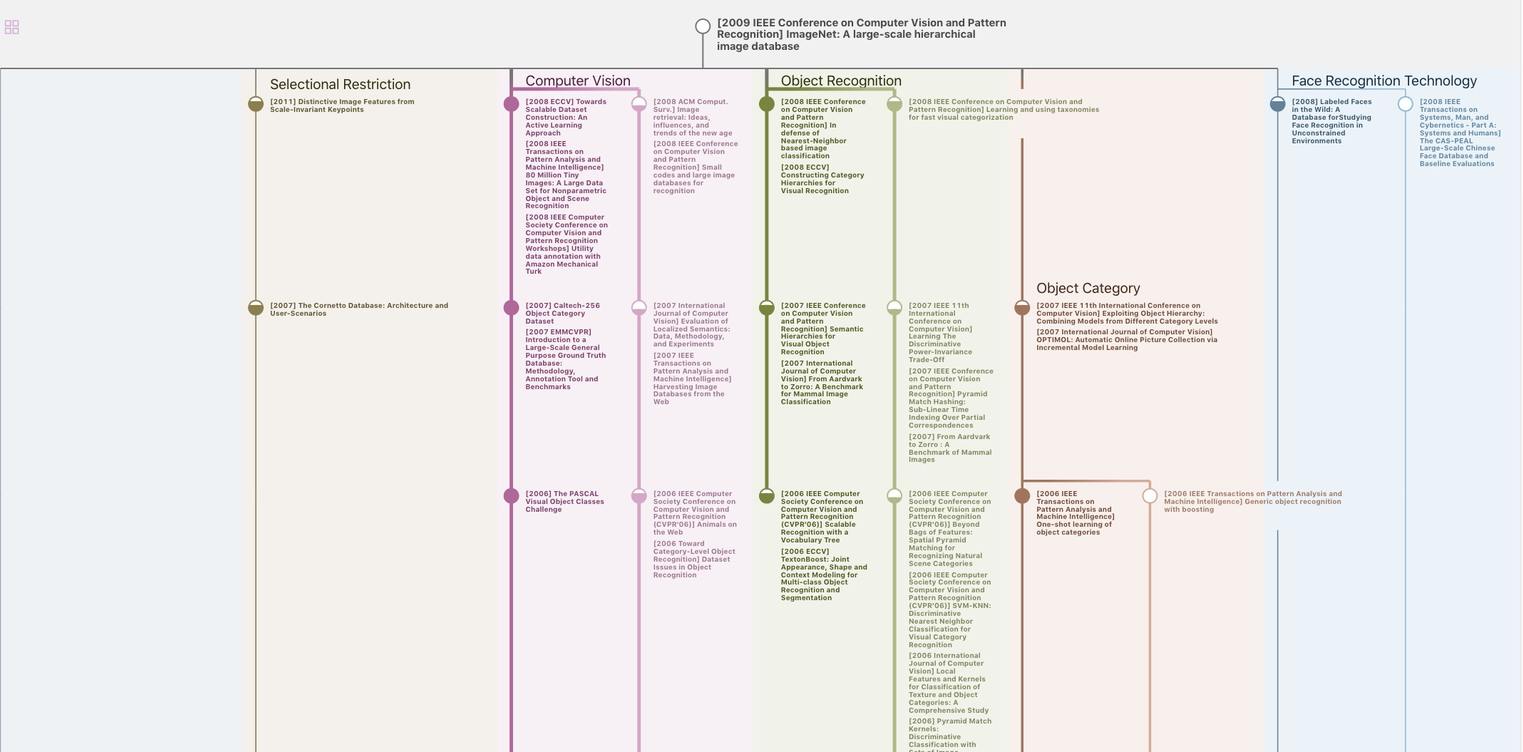
生成溯源树,研究论文发展脉络
Chat Paper
正在生成论文摘要