Deep scene-scale material estimation from multi-view indoor captures.
Comput. Graph.(2022)
摘要
The movie and video game industries have adopted photogrammetry as a way to create digital 3D assets from multiple photographs of a real-world scene. But photogrammetry algorithms typically output an RGB texture atlas of the scene that only serves as visual guidance for skilled artists to create material maps suitable for physically-based rendering. We present a learning-based approach that automatically produces digital assets ready for physically-based rendering, by estimating approximate material maps from multi-view captures of indoor scenes that are used with retopologized geometry. We base our approach on a material estimation Convolutional Neural Network (CNN) that we execute on each input image. We leverage the view-dependent visual cues provided by the multiple observations of the scene by gathering, for each pixel of a given image, the color of the corresponding point in other images. This image-space CNN provides us with an ensemble of predictions, which we merge in texture space as the last step of our approach. Our results demonstrate that the recovered assets can be directly used for physically-based rendering and editing of real indoor scenes from any viewpoint and novel lighting. Our method generates approximate material maps in a fraction of time compared to the closest previous solutions.(c) 2022 Elsevier Ltd. All rights reserved.
更多查看译文
关键词
Material estimation,Deep learning,Indoor scenes,Photogrammetry,Synthetic dataset,Digital 3D assets
AI 理解论文
溯源树
样例
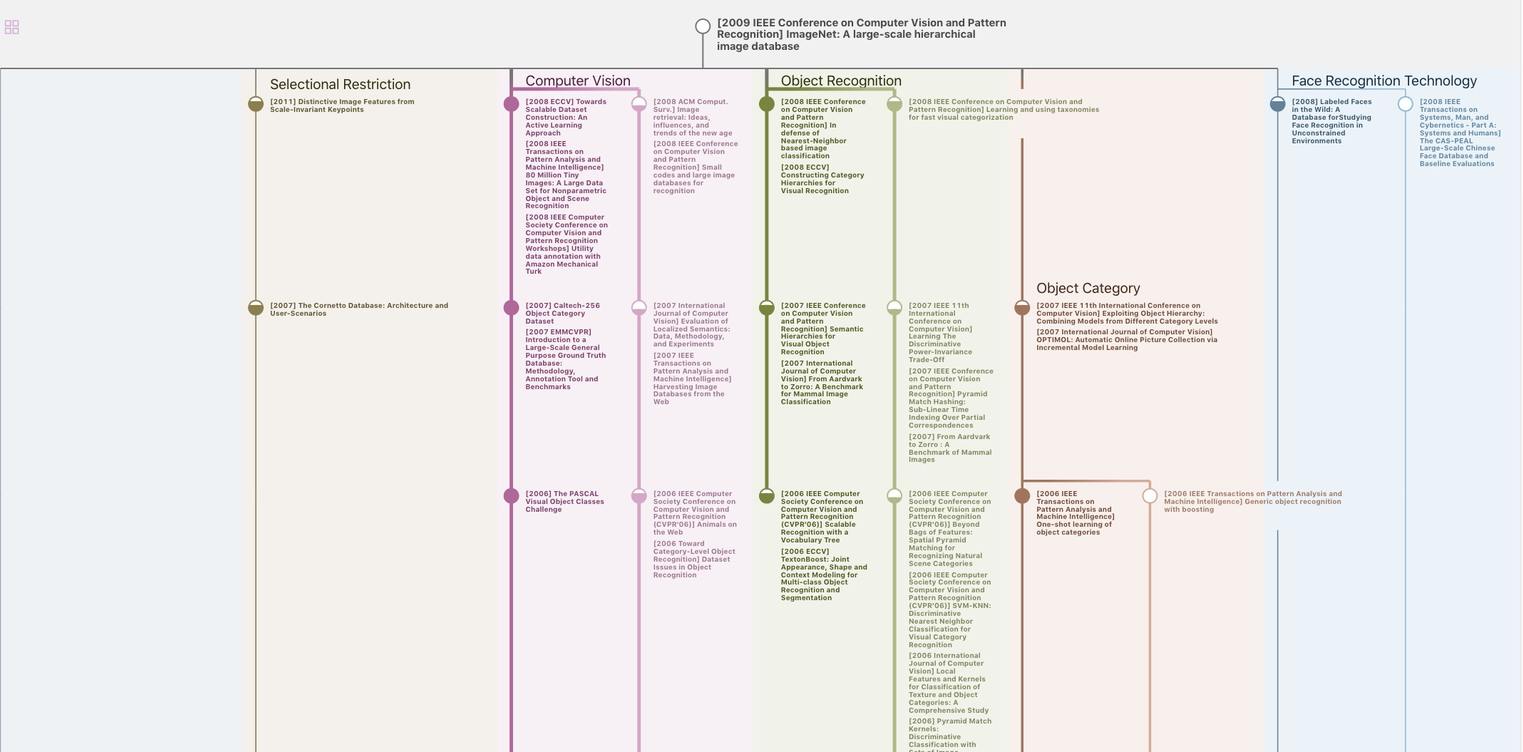
生成溯源树,研究论文发展脉络
Chat Paper
正在生成论文摘要