Remote sensing retrieval of inland water quality parameters using Sentinel-2 and multiple machine learning algorithms
ENVIRONMENTAL SCIENCE AND POLLUTION RESEARCH(2022)
摘要
Remote sensing has long been an effective method for water quality monitoring because of its advantages such as high coverage and low consumption. For non-optically active parameters, traditional empirical and analytical methods cannot achieve quantitative retrieval. Machine learning has been gradually used for water quality retrieval due to its ability to capture the potential relationship between water quality parameters and satellite images. This study is based on Sentinel-2 images and compared the ability of four machine learning algorithms (eXtreme Gradient Boosting (XGBoost), Support Vector Regression (SVR), Random Forest (RF), and Artificial Neural Network (ANN)) to retrieve chlorophyll-a (Chl-a), dissolved oxygen (DO), and ammonia-nitrogen (NH 3 -N) for inland reservoirs. The results indicated that XGBoost outperformed the other three algorithms. We used XGBoost to reconstruct the spatial-temporal patterns of Chl-a, DO, and NH 3 -N for the period of 2018–2020 and further analyzed the interannual, seasonal, and spatial variation characteristics. This study provides an efficient and practical way for optically and non-optically active parameters monitoring and management at the regional scale.
更多查看译文
关键词
Remote sensing,Water quality,Machine learning,Non-optically active parameters,Sentinel-2,Inland waters
AI 理解论文
溯源树
样例
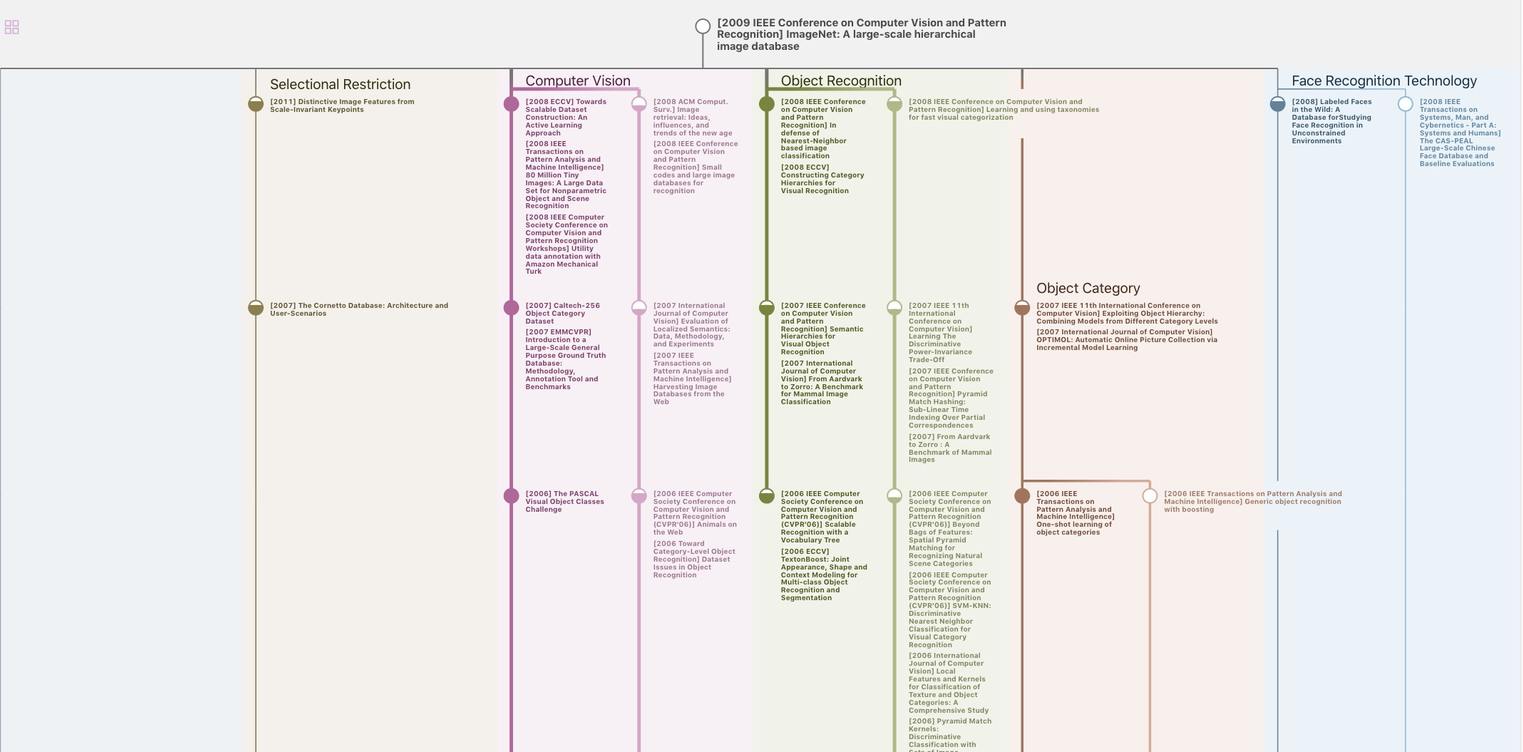
生成溯源树,研究论文发展脉络
Chat Paper
正在生成论文摘要