Imitated Detectors
Proceedings of the 30th ACM International Conference on Multimedia(2022)
摘要
Deep neural networks have shown great potential in many practical applications, yet their knowledge is at the risk of being stolen via exposed services (\eg APIs). In contrast to the commonly-studied classification model extraction, there exist no studies on the more challenging object detection task due to the sufficiency and efficiency of problem domain data collection. In this paper, we for the first time reveal that black-box victim object detectors can be easily replicated without knowing the model structure and training data. In particular, we treat it as black-box knowledge distillation and propose a teacher-student framework named Imitated Detector to transfer the knowledge of the victim model to the imitated model. To accelerate the problem domain data construction, we extend the problem domain dataset by generating synthetic images, where we apply the text-image generation process and provide short text inputs consisting of object categories and natural scenes; to promote the feedback information, we aim to fully mine the latent knowledge of the victim model by introducing an iterative adversarial attack strategy, where we feed victim models with transferable adversarial examples making victim provide diversified predictions with more information. Extensive experiments on multiple datasets in different settings demonstrate that our approach achieves the highest model extraction accuracy and outperforms other model stealing methods by large margins in the problem domain dataset. Our codes can be found at \urlhttps://github.com/LiangSiyuan21/Imitated-Detectors.
更多查看译文
AI 理解论文
溯源树
样例
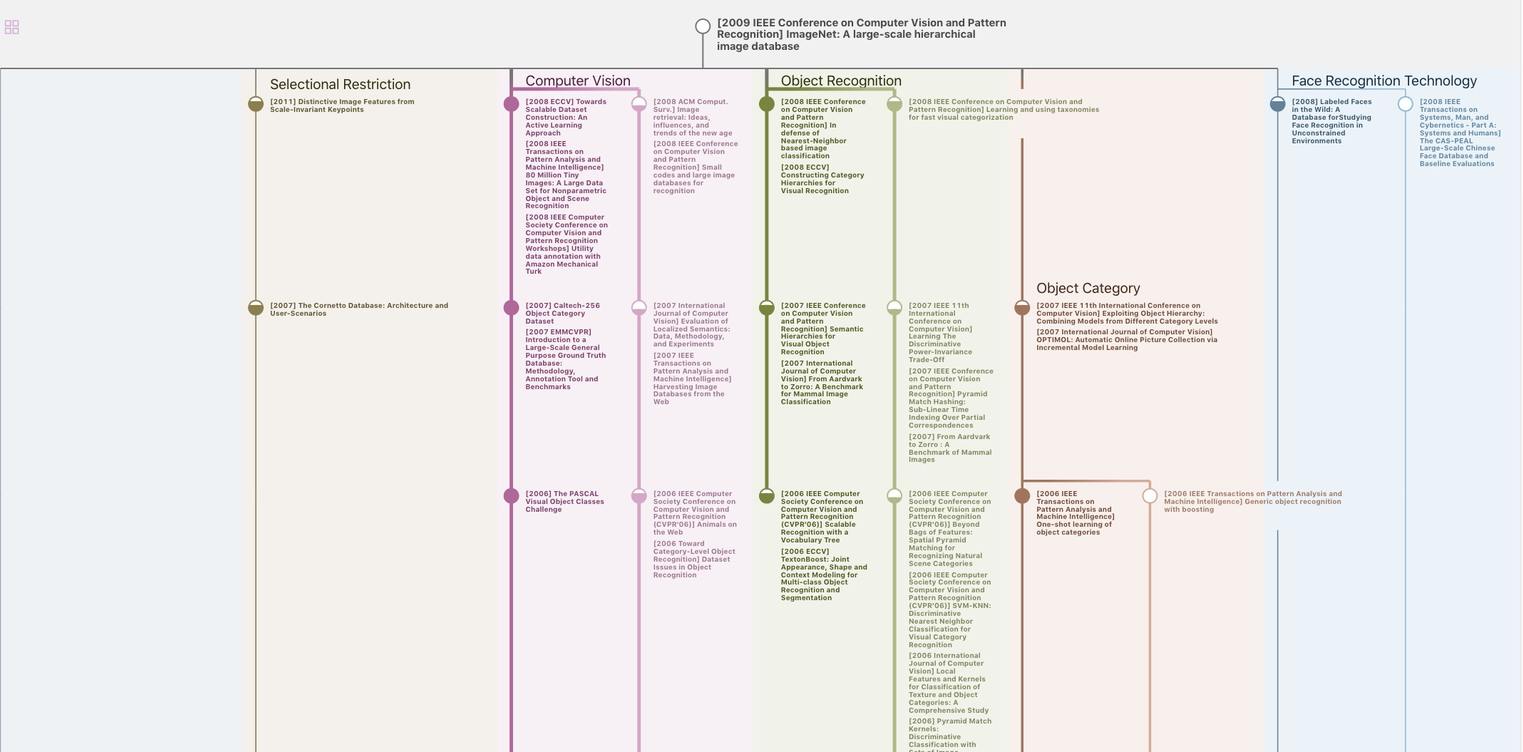
生成溯源树,研究论文发展脉络
Chat Paper
正在生成论文摘要