A compression strategy to accelerate LSTM meta-learning on FPGA
ICT Express(2022)
摘要
Driven by edge computing, how to efficiently deploy the meta-learner LSTM in the resource constrained FPGA terminal equipment has become a big problem. This paper proposes a compression strategy based on LSTM meta-learning model, which combined the structured pruning of the weight matrix and the mixed precision quantization. The weight matrix was pruned into a sparse matrix, then the weight was quantified to reduce resource consumption. Finally, a LSTM meta-learning accelerator was designed based on the idea of hardware–software cooperation. Experiments show that compared with mainstream hardware platforms, the proposed accelerator achieves at least 50.14 times increase in energy efficiency.
更多查看译文
关键词
Edge calculation,FPGA,LSTM Meta-Learning Accelerator,Structural pruning,Mixed precision quantization
AI 理解论文
溯源树
样例
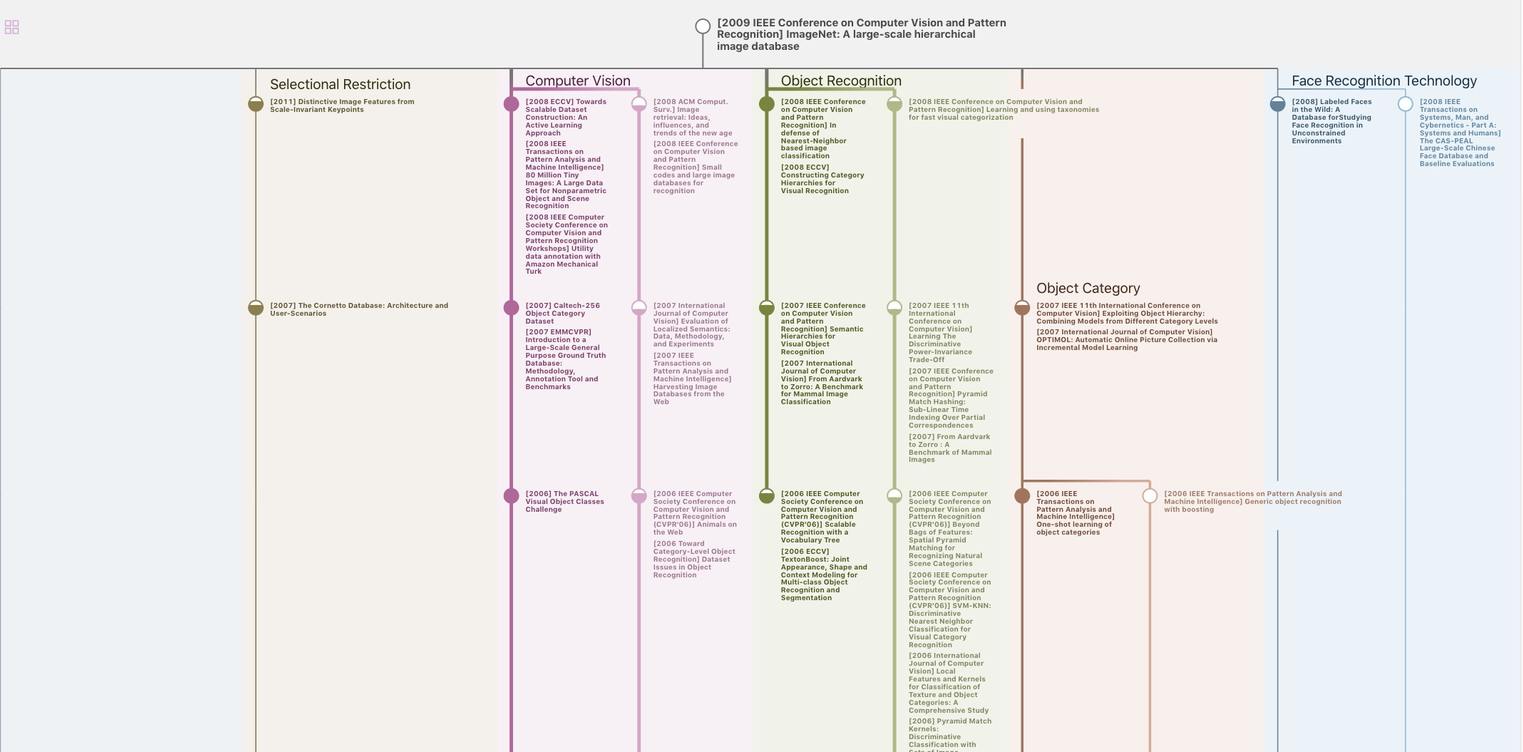
生成溯源树,研究论文发展脉络
Chat Paper
正在生成论文摘要