Adaptive distribution calibration for few-shot learning via optimal transport
Information Sciences(2022)
摘要
Few-shot learning (FSL) is a challenging task in the community of data mining but frequently appears in real-world applications. A popular strategy for FSL is transferring information from the domain with extensive amounts of data to the domain with few data. However, two main issues are still open for transfer learning: what kind of information and how much should be transferred. In this work, an Adaptive Distribution Calibration (ADC) is designed to adaptively transfer distribution informase classes for calibrating the biased distributions of novel classes. More specifically, ADC automatically determines the correlations between base classes and novel classes by considering the optimal transport among them. Then, ADC adaptively calibrates the distribution of each novel class according to its correlated base classes. More novel class data can be sampled from the calibrated distribution to train a robust classifier. Furthermore, we theoretically analyze the generalization error bound of the proposed ADC, which shows that the best hypothesis that considers both support and generated data performs at least as good as the best hypothesis learned on support data alone. This generalization error bound guarantees theoretically the effectiveness of the proposed method. Extensive experiments, including comparing with baselines(0.17% ∼ 2.6% improvement on different datasets compared to the next best), ablation studies and hyper-parameter analysis, have been conducted on three widely used FSL datasets (miniImageNet, tieredImageNet and CUB) to demonstrate the effectiveness of ADC.
更多查看译文
关键词
Few-shot learning,Adaptive distribution calibration,Optimal transport,Data augmentation
AI 理解论文
溯源树
样例
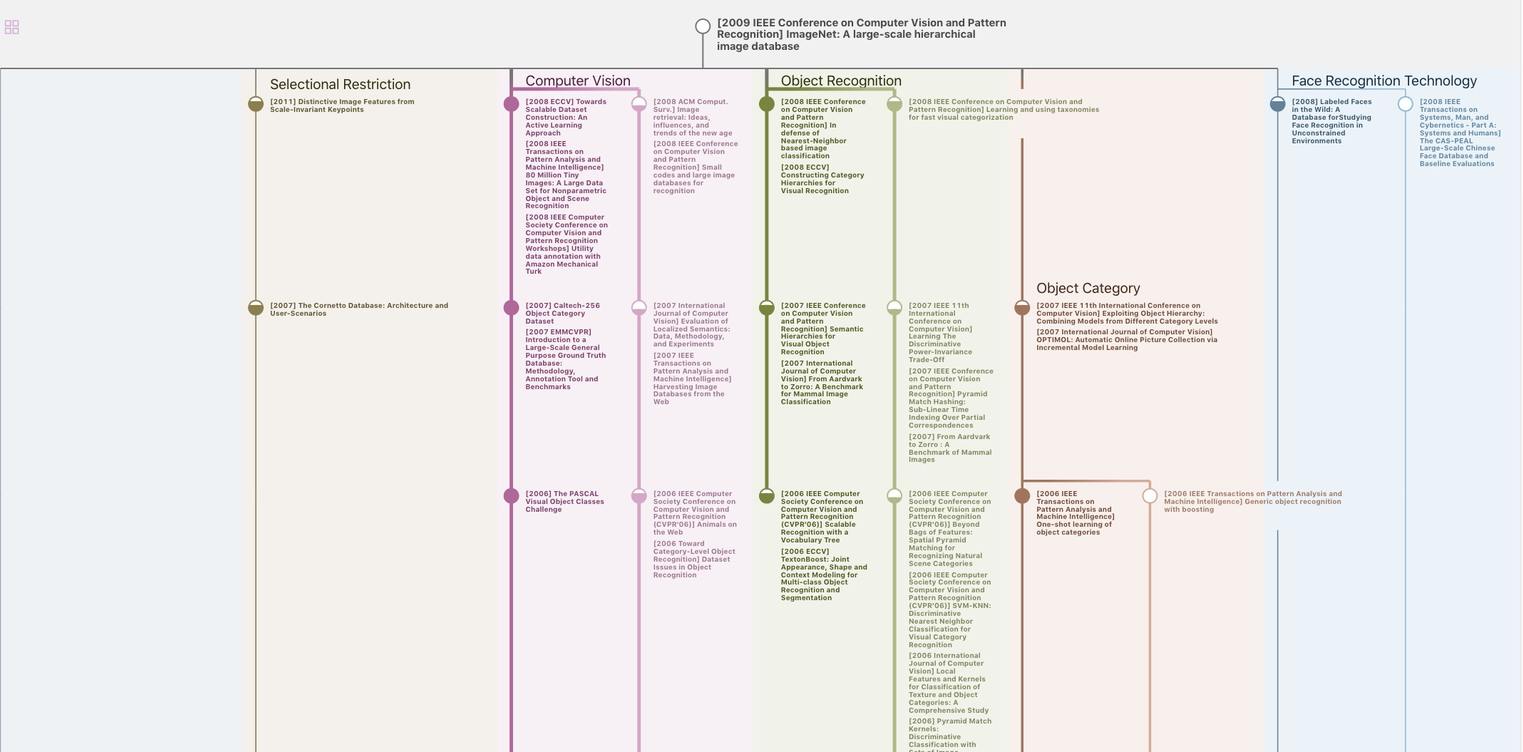
生成溯源树,研究论文发展脉络
Chat Paper
正在生成论文摘要