Development and validation of a clinical decision-support tool for prediction of weaning outcome on admission to the icu
Chest(2022)
摘要
SESSION TITLE: Encounters with Mechanical VentilationSESSION TYPE: Rapid Fire Original InvPRESENTED ON: 10/17/2022 12:15 pm - 1:15 pmPURPOSE: Predicting weaning outcomes of mechanically ventilated intensive care unit (ICU) patients is a challenging dilemma for clinicians. This study used machine learning methods to predict the risk for failure to wean from mechanical ventilation (MV) upon admission to the ICU.METHODS: Data were extracted from The Medical Information Mart for Intensive Care III (MIMIC-III) Database. Inclusion criteria included: 1) Age >=18 years and 2) ICU admission undergoing ventilation. Clinical variables were collected upon ICU admission and throughout their ICU stay. Patients were initially classified into one of four groups according to the Weaning According to a New Definition (WIND) classification which classifies patients based on their weaning outcome: Group 0 if no separation attempt (SA) is performed, Group 1 or short weaning (SA <24 hours) , Group 2 or difficult weaning (SA takes >1 day and <1 week), and Group 3 or prolonged weaning (SA is 1 week or longer). Patients were excluded if they did not experience any SA (group 0) or died within 7 days after extubation.For the analysis, patients weaned off MV in 7 days or less (WIND groups 1-2) were labelled as weaning success and patients with prolonged weaning (WIND group 3) were labelled as weaning failure. Classification models included logistic regression (LR), random forest (RF), and Extreme Gradient Boosting (XGBoost). A decision curve analysis (DCA) was plotted to evaluate the clinical utility of the model.RESULTS: Of 46520 records, 12908 met the inclusion criteria. 1569 patients did not undergo any SA and 1233 died within 7 days from extubation and were excluded from the analysis. Patients in the ‘failed weaning’ group (n=1241) had more comorbidities (acute respiratory failure, CHF, atrial fibrillation), higher severity of illness scores, and higher MV parameters than the ‘successful weaning’ group (n= 8865). The AUCs using RF was 0.920 (95% CI, ±0.007), XGBoost was 0.919 (95% CI, ±0.007), and LR of 0.687 (95% CI, ±0.016). For the XGBoost model, the top 3 predictive features for weaning failure included acute respiratory failure, history of atrial fibrillation, and spontaneous tidal volume prior to extubation. The DCA showed XGBoost with a 20% additional net benefit compared to the RF model in patients at high-risk thresholds.CONCLUSIONS: We were able to successfully predict weaning outcome in ICU patients undergoing MV. XGBoost and RF demonstrated high precision in predicting patients that were at risk of weaning failure.CLINICAL IMPLICATIONS: There are a lack of reliable tools that aid clinicians in predicting which patients are prone to weaning failure upon ICU admission. Our clinical decision support tool can identify patients at risk of weaning failure upon ICU admission to allow for implementation of appropriate early interventions and reduce patient complications. Future prospective multi-institutional studies should validate the utility of this tool.DISCLOSURES: No relevant relationships by Ameen AmanianNo relevant relationships by Zachary BoroughsNo relevant relationships by Jacob DesmanNo relevant relationships by Joseph GreensteinNo relevant relationships by Naomi KifleNo relevant relationships by Pedro Mendez-TellezNo relevant relationships by Matthew NguyenNo relevant relationships by Shreyash SonthaliaNo relevant relationships by Casey TaylorNo relevant relationships by Meihan WeiNo relevant relationships by Tony WeiNo relevant relationships by Xihan Zhao SESSION TITLE: Encounters with Mechanical Ventilation SESSION TYPE: Rapid Fire Original Inv PRESENTED ON: 10/17/2022 12:15 pm - 1:15 pm PURPOSE: Predicting weaning outcomes of mechanically ventilated intensive care unit (ICU) patients is a challenging dilemma for clinicians. This study used machine learning methods to predict the risk for failure to wean from mechanical ventilation (MV) upon admission to the ICU. METHODS: Data were extracted from The Medical Information Mart for Intensive Care III (MIMIC-III) Database. Inclusion criteria included: 1) Age >=18 years and 2) ICU admission undergoing ventilation. Clinical variables were collected upon ICU admission and throughout their ICU stay. Patients were initially classified into one of four groups according to the Weaning According to a New Definition (WIND) classification which classifies patients based on their weaning outcome: Group 0 if no separation attempt (SA) is performed, Group 1 or short weaning (SA <24 hours) , Group 2 or difficult weaning (SA takes >1 day and <1 week), and Group 3 or prolonged weaning (SA is 1 week or longer). Patients were excluded if they did not experience any SA (group 0) or died within 7 days after extubation. For the analysis, patients weaned off MV in 7 days or less (WIND groups 1-2) were labelled as weaning success and patients with prolonged weaning (WIND group 3) were labelled as weaning failure. Classification models included logistic regression (LR), random forest (RF), and Extreme Gradient Boosting (XGBoost). A decision curve analysis (DCA) was plotted to evaluate the clinical utility of the model. RESULTS: Of 46520 records, 12908 met the inclusion criteria. 1569 patients did not undergo any SA and 1233 died within 7 days from extubation and were excluded from the analysis. Patients in the ‘failed weaning’ group (n=1241) had more comorbidities (acute respiratory failure, CHF, atrial fibrillation), higher severity of illness scores, and higher MV parameters than the ‘successful weaning’ group (n= 8865). The AUCs using RF was 0.920 (95% CI, ±0.007), XGBoost was 0.919 (95% CI, ±0.007), and LR of 0.687 (95% CI, ±0.016). For the XGBoost model, the top 3 predictive features for weaning failure included acute respiratory failure, history of atrial fibrillation, and spontaneous tidal volume prior to extubation. The DCA showed XGBoost with a 20% additional net benefit compared to the RF model in patients at high-risk thresholds. CONCLUSIONS: We were able to successfully predict weaning outcome in ICU patients undergoing MV. XGBoost and RF demonstrated high precision in predicting patients that were at risk of weaning failure. CLINICAL IMPLICATIONS: There are a lack of reliable tools that aid clinicians in predicting which patients are prone to weaning failure upon ICU admission. Our clinical decision support tool can identify patients at risk of weaning failure upon ICU admission to allow for implementation of appropriate early interventions and reduce patient complications. Future prospective multi-institutional studies should validate the utility of this tool. DISCLOSURES: No relevant relationships by Ameen Amanian No relevant relationships by Zachary Boroughs No relevant relationships by Jacob Desman No relevant relationships by Joseph Greenstein No relevant relationships by Naomi Kifle No relevant relationships by Pedro Mendez-Tellez No relevant relationships by Matthew Nguyen No relevant relationships by Shreyash Sonthalia No relevant relationships by Casey Taylor No relevant relationships by Meihan Wei No relevant relationships by Tony Wei No relevant relationships by Xihan Zhao
更多查看译文
关键词
weaning outcome validation admission,icu,decision-support
AI 理解论文
溯源树
样例
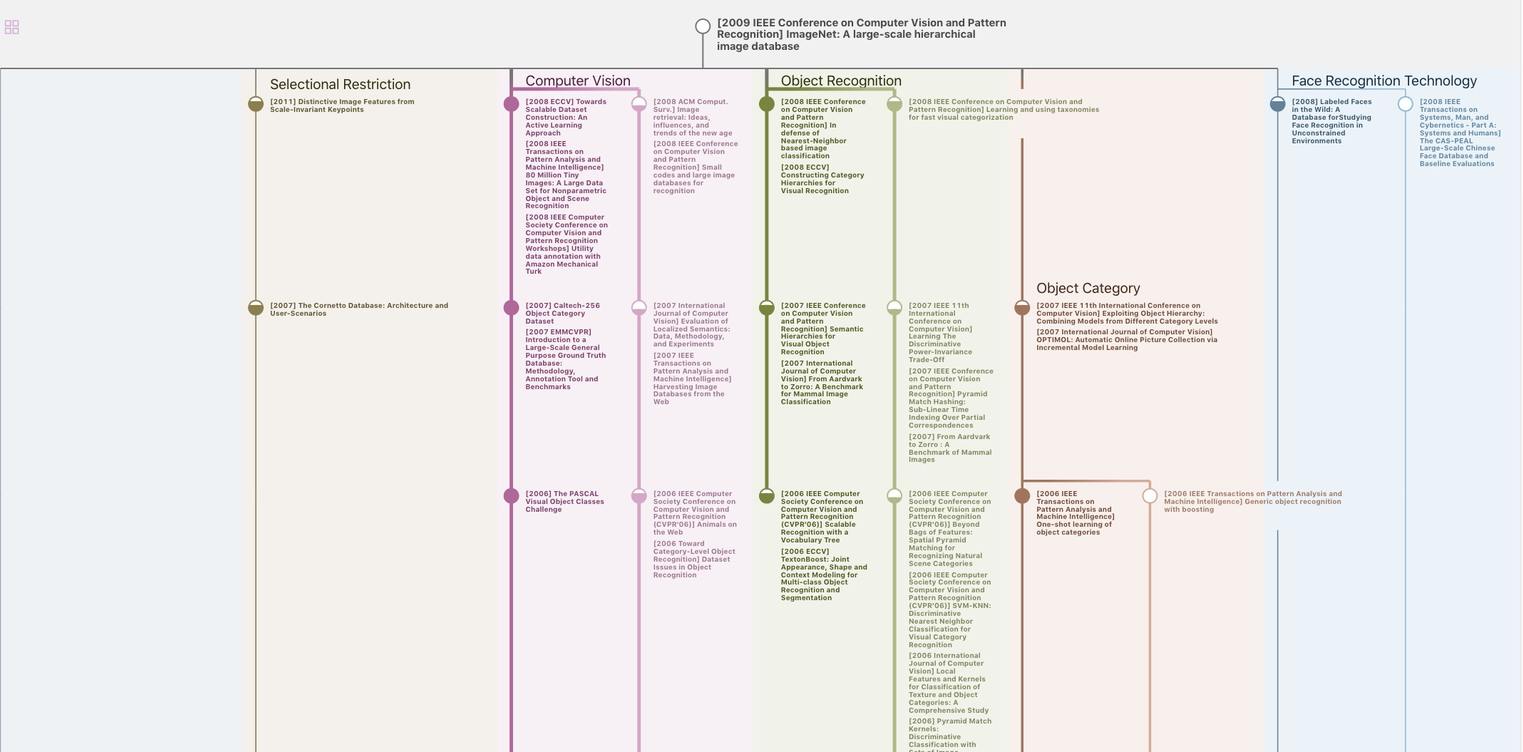
生成溯源树,研究论文发展脉络
Chat Paper
正在生成论文摘要