Deep learning based liquid level extraction from video observations of gas–liquid flows
International Journal of Multiphase Flow(2022)
摘要
The slug flow pattern is one of the most common gas–liquid flow patterns in multiphase transportation pipelines, particularly in the oil and gas industry. This flow pattern can cause severe problems for industrial processes. Hence, a detailed description of the spatial distribution of the different phases in the pipe is needed for automated process control and calibration of predictive models. In this paper, a deep-learning based image processing technique is presented that extracts the gas–liquid interface from video observations of multiphase flows in horizontal pipes. The supervised deep learning model consists of a convolutional neural network, which was trained and tested with video data from slug flow experiments. The consistency of the hand-labelled data and the predictions of the trained model have been evaluated in an inter-observer reliability test. The model was further tested with other data sets, which also included recordings of a different flow pattern. It is shown that the presented method provides accurate and reliable predictions of the gas–liquid interface for slug flow as well as for other separate flow patterns. Moreover, it is demonstrated how flow characteristics can be obtained from the results of the deep-learning based image processing technique.
更多查看译文
关键词
Multiphase flow,Gas–liquid interface,Deep learning,Image processing,Convolutional neural network,Videometry
AI 理解论文
溯源树
样例
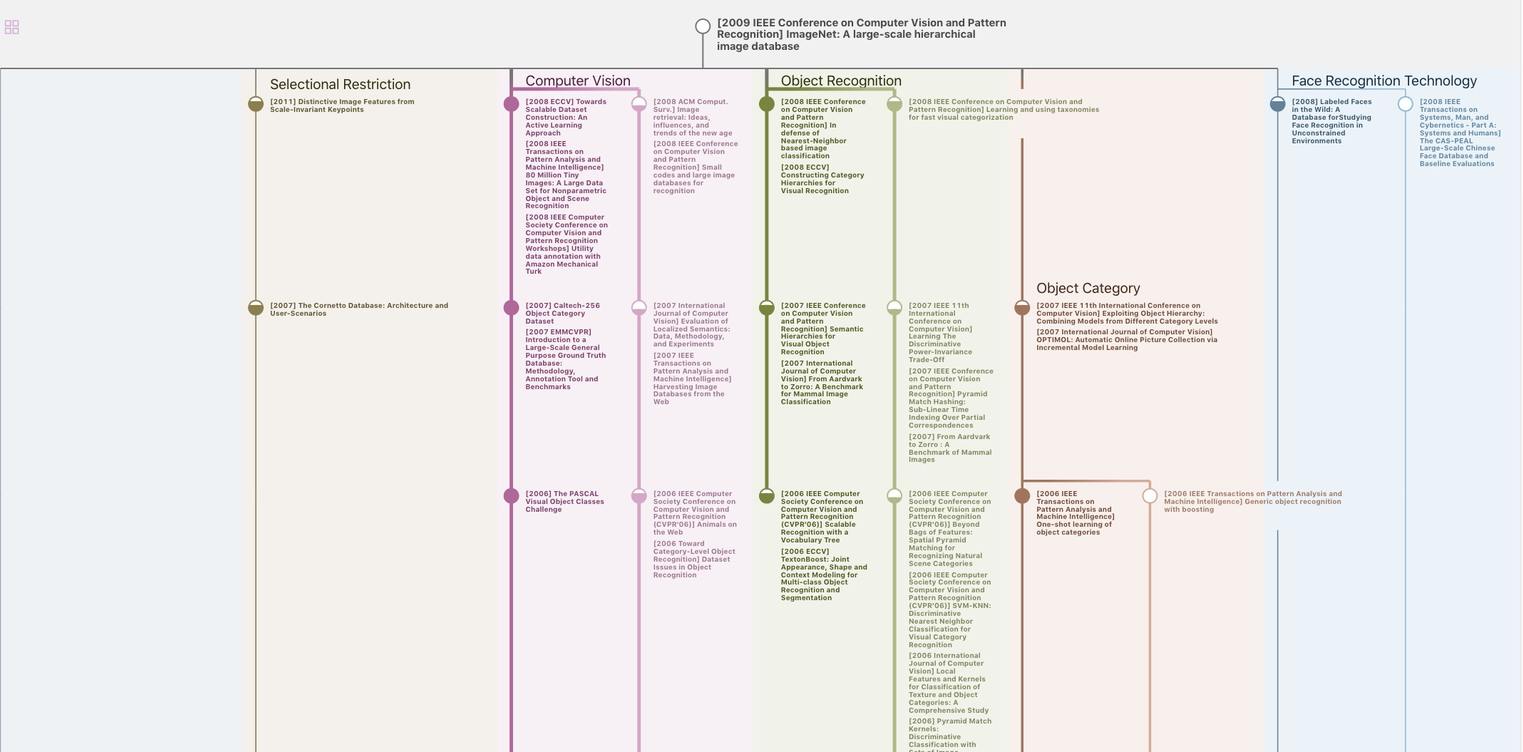
生成溯源树,研究论文发展脉络
Chat Paper
正在生成论文摘要