Automated Tumor Segmentation in Radiotherapy.
Seminars in radiation oncology(2022)
摘要
Autosegmentation of gross tumor volumes holds promise to decrease clinical demand and to provide consistency across clinicians and institutions for radiation treatment planning. Additionally, autosegmentation can enable imaging analyses such as radiomics to construct and deploy large studies with thousands of patients. Here, we review modern results that utilize deep learning approaches to segment tumors in 5 major clinical sites: brain, head and neck, thorax, abdomen, and pelvis. We focus on approaches that inch closer to clinical adoption, highlighting winning entries in international competitions, unique network architectures, and novel ways of overcoming specific challenges. We also broadly discuss the future of gross tumor volumes autosegmentation and the remaining barriers that must be overcome before widespread replacement or augmentation of manual contouring.
更多查看译文
AI 理解论文
溯源树
样例
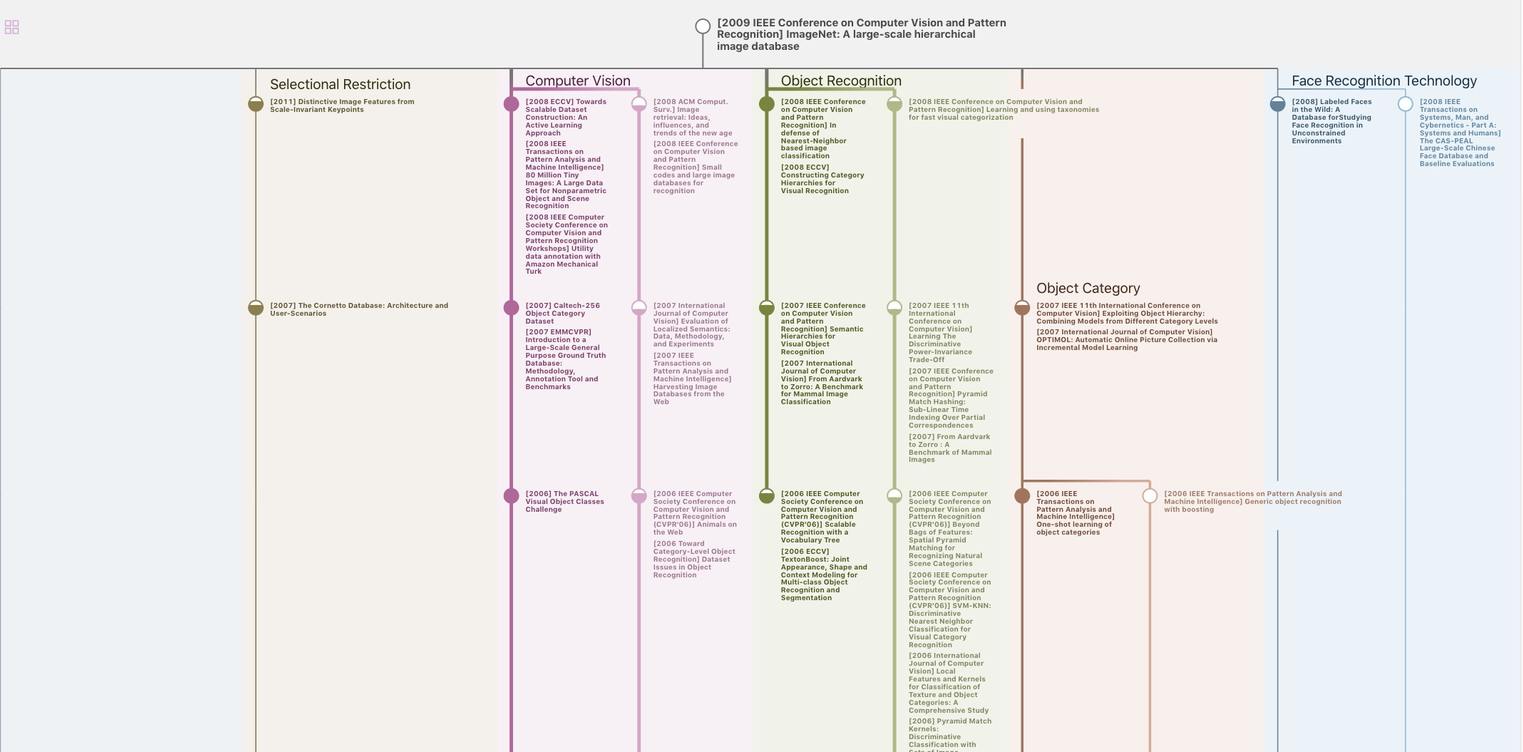
生成溯源树,研究论文发展脉络
Chat Paper
正在生成论文摘要