Large-scale Model Personalization via Low Rank and Sparse decomposition
arxiv(2023)
摘要
Personalization of machine learning (ML) predictions for individual users/domains/enterprises is critical for practical recommendation style systems. Standard personalization approaches involve learning a user/domain specific embedding that is fed into a fixed global model which can be limiting. On the other hand, personalizing/fine-tuning model itself for each user/domain -- a.k.a meta-learning -- has high storage/infrastructure cost. We propose a novel meta-learning style approach that models network weights as a sum of low-rank and sparse matrices. This captures common information from multiple individuals/users together in the low-rank part while sparse part captures user-specific idiosyncrasies. Furthermore, the framework is up to two orders of magnitude more scalable (in terms of storage/infrastructure cost) than user-specific finetuning of model. We then study the framework in the linear setting, where the problem reduces to that of estimating the sum of a rank-$r$ and a $k$-column sparse matrix using a small number of linear measurements. We propose an alternating minimization method with iterative hard thresholding -- AMHT-LRS -- to learn the low-rank and sparse part. For the realizable, Gaussian data setting, we show that AMHT-LRS solves the problem efficiently with nearly optimal samples. A significant challenge in personalization is ensuring privacy of each user's sensitive data. We alleviate this problem by proposing a differentially private variant of our method that also is equipped with strong generalization guarantees. Finally, on multiple standard recommendation datasets, we demonstrate that our approach allows personalized models to obtain superior performance in sparse data regime.
更多查看译文
AI 理解论文
溯源树
样例
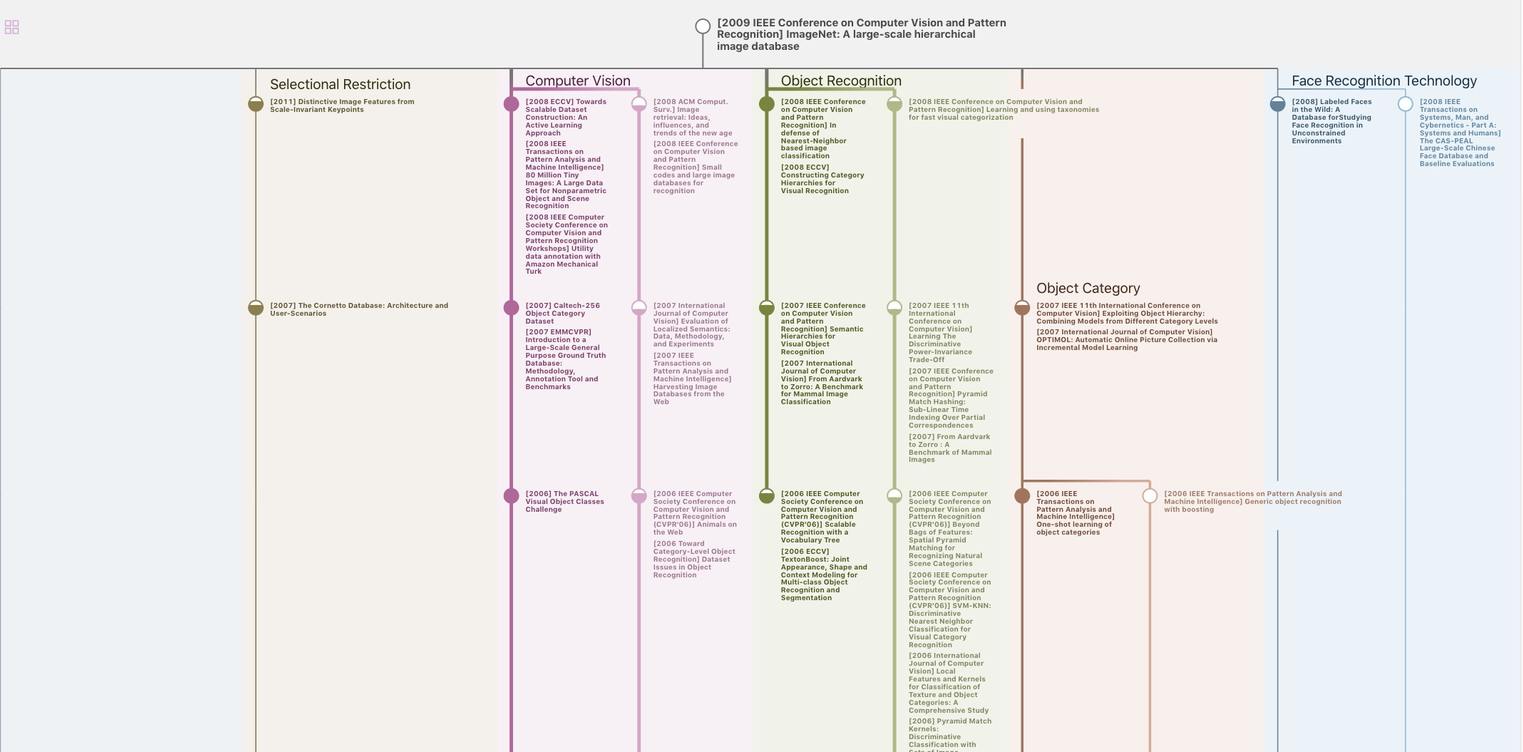
生成溯源树,研究论文发展脉络
Chat Paper
正在生成论文摘要