Mutual Learning of Single- and Multi-Channel End-to-End Neural Diarization
2022 IEEE Spoken Language Technology Workshop (SLT)(2023)
摘要
Due to the high performance of multi-channel speech processing, we can use the outputs from a multi-channel model as teacher labels when training a single-channel model with knowledge distillation. To the contrary, it is also known that single-channel speech data can benefit multi-channel models by mixing it with multi-channel speech data during training or by using it for model pretraining. This paper focuses on speaker diarization and proposes to conduct the above bi-directional knowledge transfer alternately. We first introduce an end-to-end neural diarization model that can handle both single- and multi-channel inputs. Using this model, we alternately conduct i) knowledge distillation from a multi-channel model to a single-channel model and ii) finetuning from the distilled single-channel model to a multi-channel model. Experimental results on two-speaker data show that the proposed method mutually improved single- and multi-channel speaker diarization performances.
更多查看译文
关键词
Speaker diarization,EEND,multi-channel,knowledge distillation,transfer learning,mutual learning
AI 理解论文
溯源树
样例
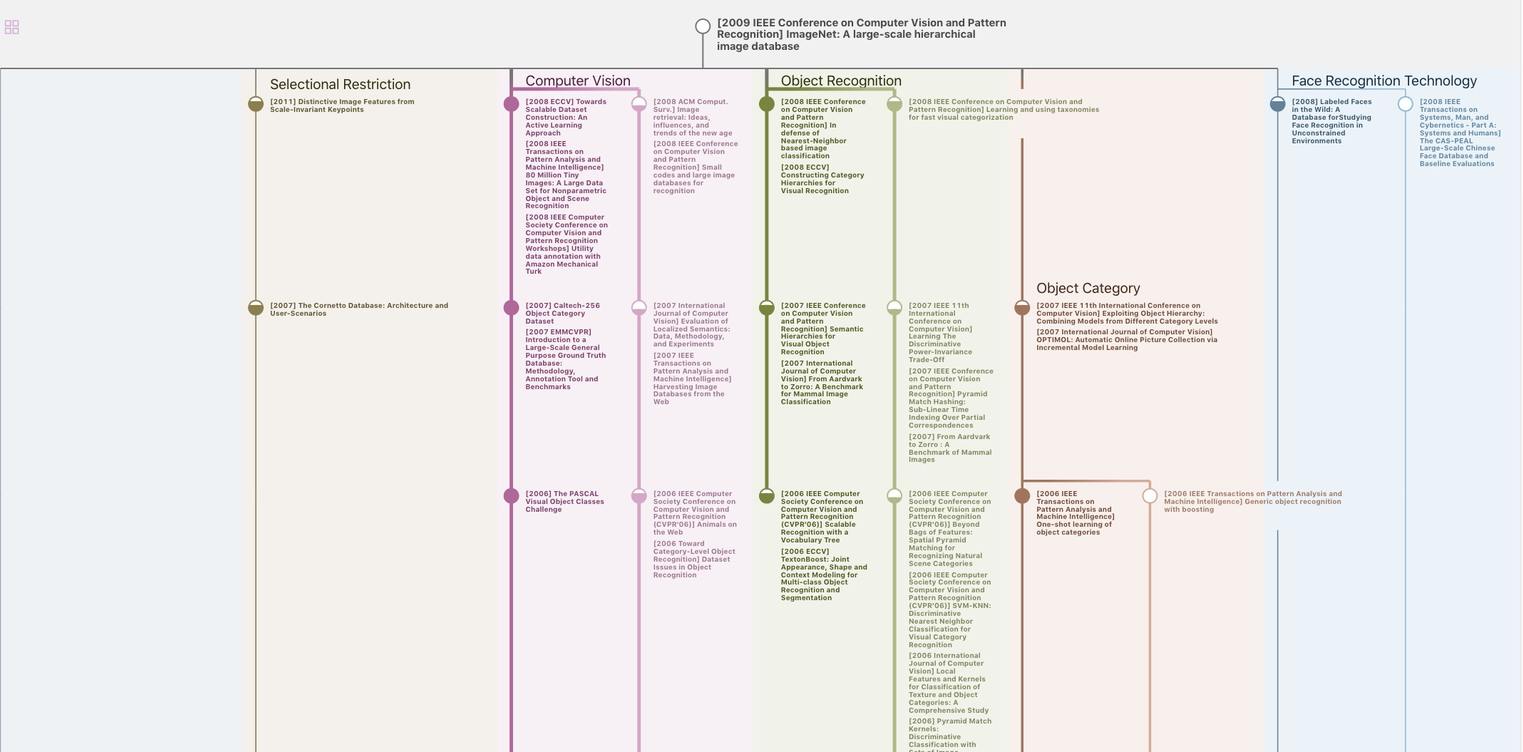
生成溯源树,研究论文发展脉络
Chat Paper
正在生成论文摘要