Depersonalized Federated Learning: Tackling Statistical Heterogeneity by Alternating Stochastic Gradient Descent
ICC 2023 - IEEE INTERNATIONAL CONFERENCE ON COMMUNICATIONS(2023)
摘要
Federated learning (FL) enables distributed clients to cooperatively train a common machine learning (ML) model for intelligent inference without raw data sharing. However, problems in practical networks, such as non-independent-and-identically-distributed (non-iid) raw data and limited network resources, lead to slow and unstable convergence of the FL training process. To address these issues, this paper proposes a new FL method that can mitigate statistical heterogeneity through the depersonalization mechanism. Specifically, we decouple the global and local optimization objectives by alternating stochastic gradient descent, thus reducing the accumulated variance in local update phases to accelerate the FL convergence. Furthermore, the proposed FL method is analyzed in detail and proved to converge at a sublinear speed under the general non-convex setting. Finally, extensive experiments are conducted on public datasets to verify the effectiveness of the proposed method with comparisons of other representative baseline methods.
更多查看译文
关键词
Federated learning,depersonalization mechanism,statistical heterogeneity,convergence analysis
AI 理解论文
溯源树
样例
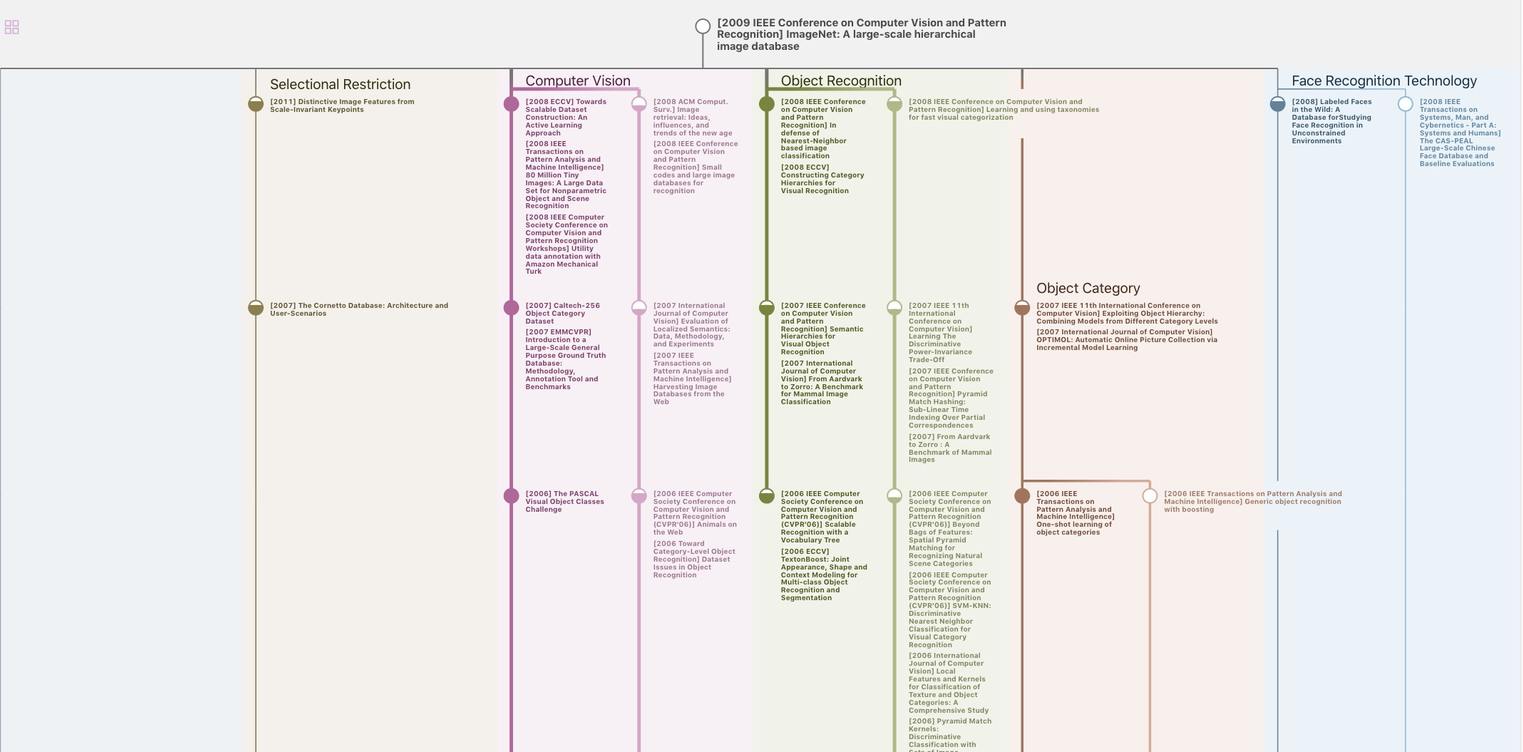
生成溯源树,研究论文发展脉络
Chat Paper
正在生成论文摘要