Deep Inventory Management
arxiv(2022)
摘要
This work provides a Deep Reinforcement Learning approach to solving a periodic review inventory control system with stochastic vendor lead times, lost sales, correlated demand, and price matching. While this dynamic program has historically been considered intractable, our results show that several policy learning approaches are competitive with or outperform classical methods. In order to train these algorithms, we develop novel techniques to convert historical data into a simulator. On the theoretical side, we present learnability results on a subclass of inventory control problems, where we provide a provable reduction of the reinforcement learning problem to that of supervised learning. On the algorithmic side, we present a model-based reinforcement learning procedure (Direct Backprop) to solve the periodic review inventory control problem by constructing a differentiable simulator. Under a variety of metrics Direct Backprop outperforms model-free RL and newsvendor baselines, in both simulations and real-world deployments.
更多查看译文
关键词
inventory,management
AI 理解论文
溯源树
样例
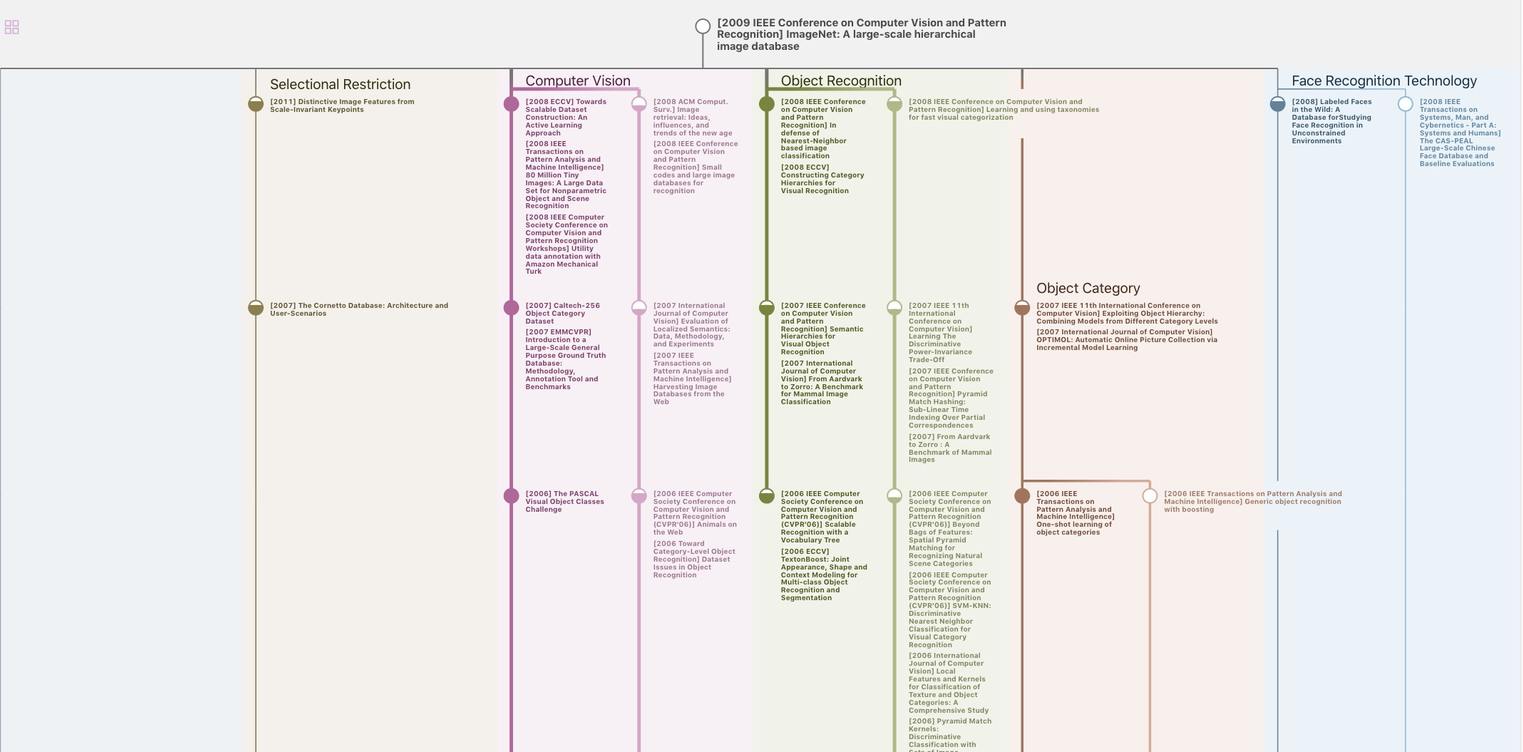
生成溯源树,研究论文发展脉络
Chat Paper
正在生成论文摘要