Recognition of GTAW weld penetration based on the lightweight model and transfer learning
Welding in the World(2022)
Abstract
Weld penetration recognition is of great significance to improve welding quality and automation level. Weld pool images can be used as the input of convolutional neural networks (CNNs) to recognize the weld penetration state, but it is difficult to design and train an efficient CNN model from scratch for real-time recognition. In the study, a flexible visual sensing welding system was developed to construct a gas tungsten arc welding (GTAW) pool image dataset. Pretrained on the ImageNet dataset, the MobileNetV2-based transfer learning model was used to fit the custom dataset and recognize weld penetration states, including lack of fusion, lack of penetration, desirable penetration, and excessive penetration. Compared with the MobileNetV2 trained from scratch and the ResNet based on transfer learning, the results show that the proposed method improves model development efficiency on small datasets, while greatly reducing the memory occupied on industrial equipment. The recognition accuracy on the validation set achieved 99.88%, and the recognition time of a single image was about 65 ms. The model was visualized by gradient-weighted class activation mapping (Grad-CAM), showing key areas the model depends on to recognize the weld penetration state. Finally, the GTAW penetration monitoring system was designed for online evaluation.
MoreTranslated text
Key words
Weld penetration recognition,Deep learning,MobileNetV2,Transfer learning,Gas tungsten arc welding
AI Read Science
Must-Reading Tree
Example
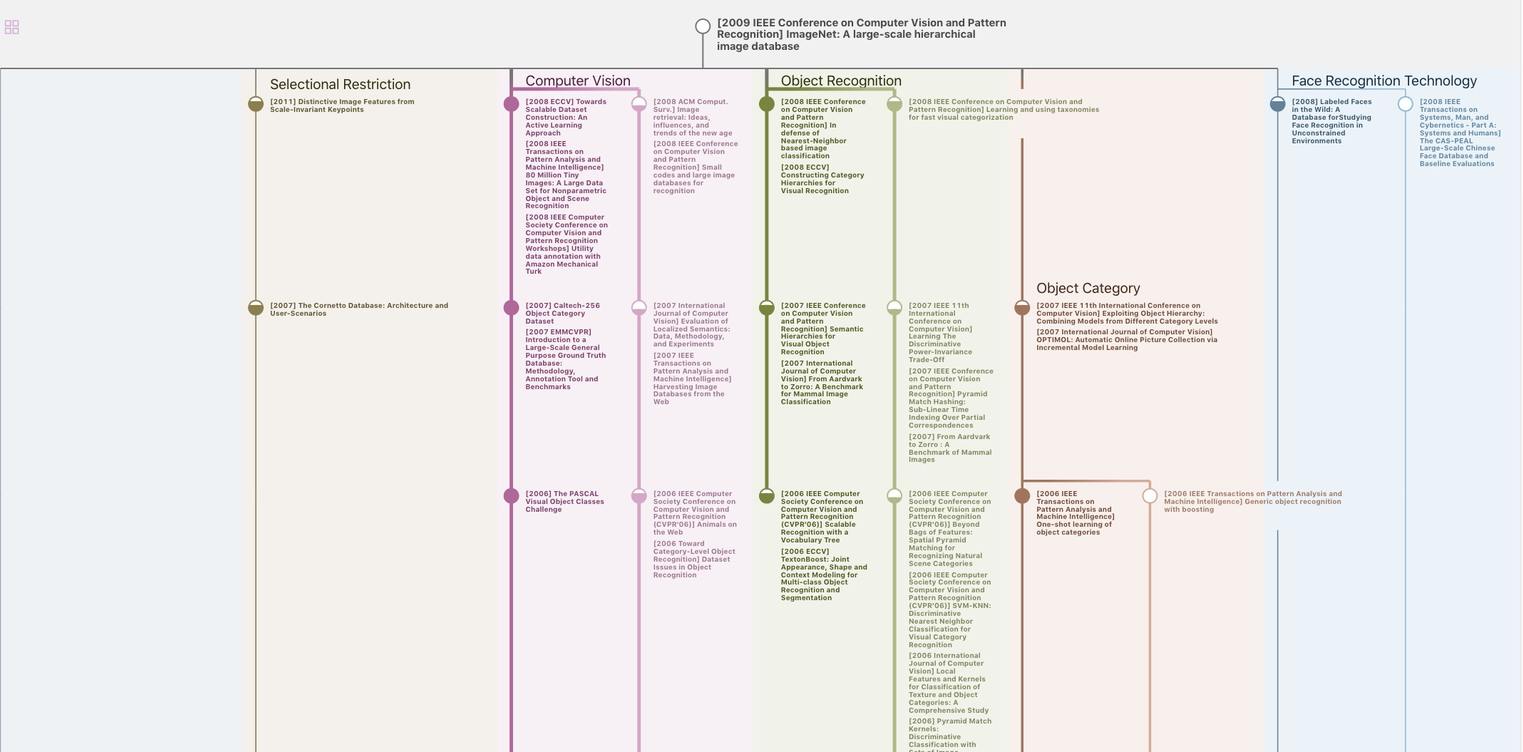
Generate MRT to find the research sequence of this paper
Chat Paper
Summary is being generated by the instructions you defined