Cross-domain fault diagnosis of bearing using improved semi-supervised meta-learning towards interference of out-of-distribution samples
Knowledge-Based Systems(2022)
摘要
The study of cross-domain semi-supervised fault diagnosis of bearings using meta-learning technique has important practical significance. However, existing methods fail to consider the interference problem caused by unlabelled out-of-distribution samples and are difficult to flexibly adapt to different diagnostic scenarios. For this purpose, a new method called improved semi-supervised meta-learning (ISSML) model is proposed in this study. First, a label allocation strategy is designed to fully utilise the information of unlabelled samples and effectively suppress the interference of out-of-distribution samples. Next, a scalable distance metric function is defined to flexibly evaluate the similarity between fault samples and efficiently extract the generic characteristics of the overall diagnostic task space. The proposed method is used to analyse experimental bearing datasets, and its superiority is demonstrated through several cross-domain few-shot scenarios and different interferences of out-of-distribution samples.
更多查看译文
关键词
Cross-domain bearing fault diagnosis,Out-of-distribution samples,Label allocation strategy,Scalable distance metric function,Meta-learning
AI 理解论文
溯源树
样例
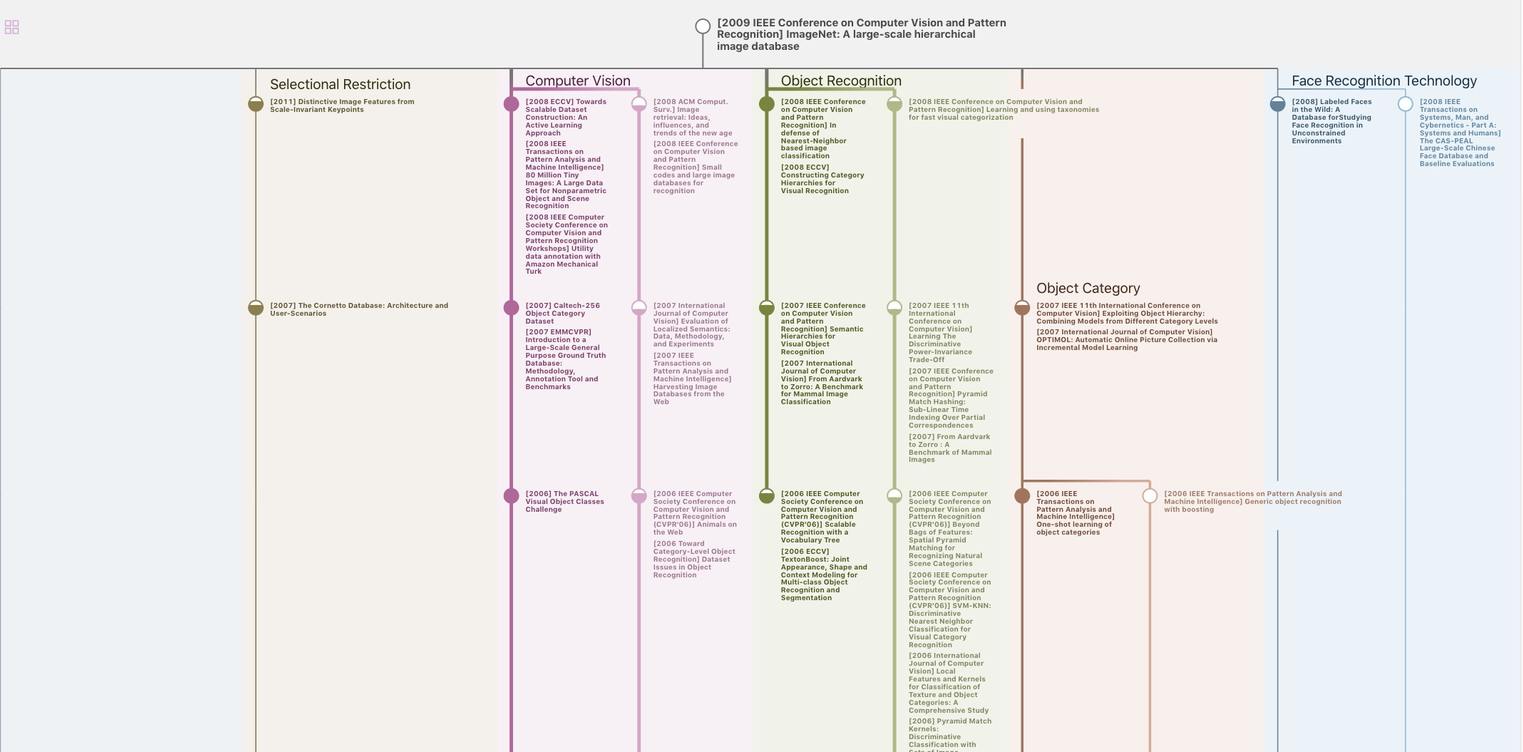
生成溯源树,研究论文发展脉络
Chat Paper
正在生成论文摘要