Deep learning based missing data recovery of non-stationary wind velocity
Journal of Wind Engineering and Industrial Aerodynamics(2022)
摘要
Wireless sensor technology and wireless sensor network are the developing trend of structural health monitoring (SHM) system in the future. Due to working principles, environment and other various factors, random data missing may occur easily during data transmission by mobile wireless sensors. The randomness and fluctuation of non-stationary wind velocity is strong and complex, affected by turbulence effect. Regarding random missing of non-stationary wind velocity in data transmission, a missing data recovery algorithm based on secondary decomposition of signals, convolutional neural network (CNN) and Kriging based sequence interpolation (KSI) is proposed in this paper. Before data transmission, the non-stationary wind velocity is decomposed by wavelet transform (WT) and empirical wavelet transform (EWT) to obtain a set of sub-signals with uniform frequency. Then low-frequency sub-signals are supplemented by cubic spline interpolation (CSI). Due to the randomness and complexity of high-frequency sub-signals, CNN is used to establish the recovery model to achieve preliminary recovery results. Generally, there exists lots of extreme points in high-frequency sub-signals. The recovery error may be large due to strong randomness of extreme points. Therefore, KSI based extreme value estimation is proposed for high-frequency sub-signals to estimate extreme curves, which are subsequently used to correct the preliminary recovery results. Numerical examples of field monitoring downburst data testify the superiority of the proposed algorithm. In addition, structural dynamic analysis fully verifies the effectiveness of extreme value correction.
更多查看译文
关键词
Missing data recovery,Secondary decomposition,KSI based Extreme value estimation,Extreme value correction
AI 理解论文
溯源树
样例
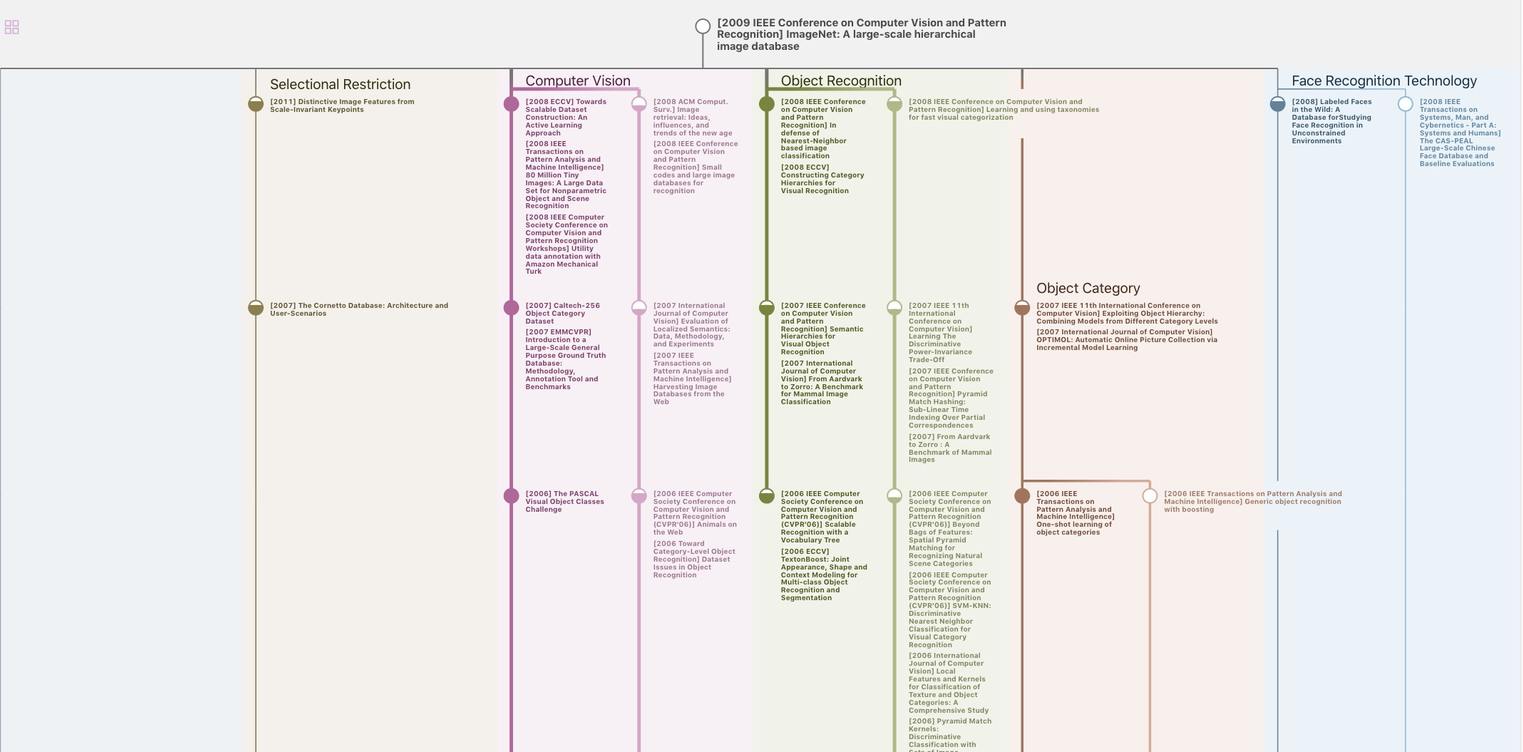
生成溯源树,研究论文发展脉络
Chat Paper
正在生成论文摘要