Confidence based class weight and embedding discrepancy constraint network for partial domain adaptation
Journal of Visual Communication and Image Representation(2022)
Abstract
Partial domain adaptation (PDA) is a special domain adaptation task where the label space of the target domain is a subset of the source domain. In this work, we present a novel adversarial PDA method named Confidence Based Class Weight and Embedding Discrepancy Constraint Network (CEN). Specifically, we design a robust weighting scheme that takes sample confidence and class information into account. It can automatically distinguish outlier samples in the source domain and reduce their importance. Besides, we consider the relationship between feature norm and domain shift. We limit the expectation of the feature norms of both domains to an adaptive value. By this means, we can align the feature distributions and help the deep model learn domain-invariant representations. Comprehensive experiments on three domain adaptation datasets Office-31, Office-home, and Visda2017 show that our approach surpasses state-of-the-art methods on various PDA tasks.
MoreTranslated text
Key words
Partial domain adaptation,Deep transfer learning,Adversarial alignment,Classification learning
AI Read Science
Must-Reading Tree
Example
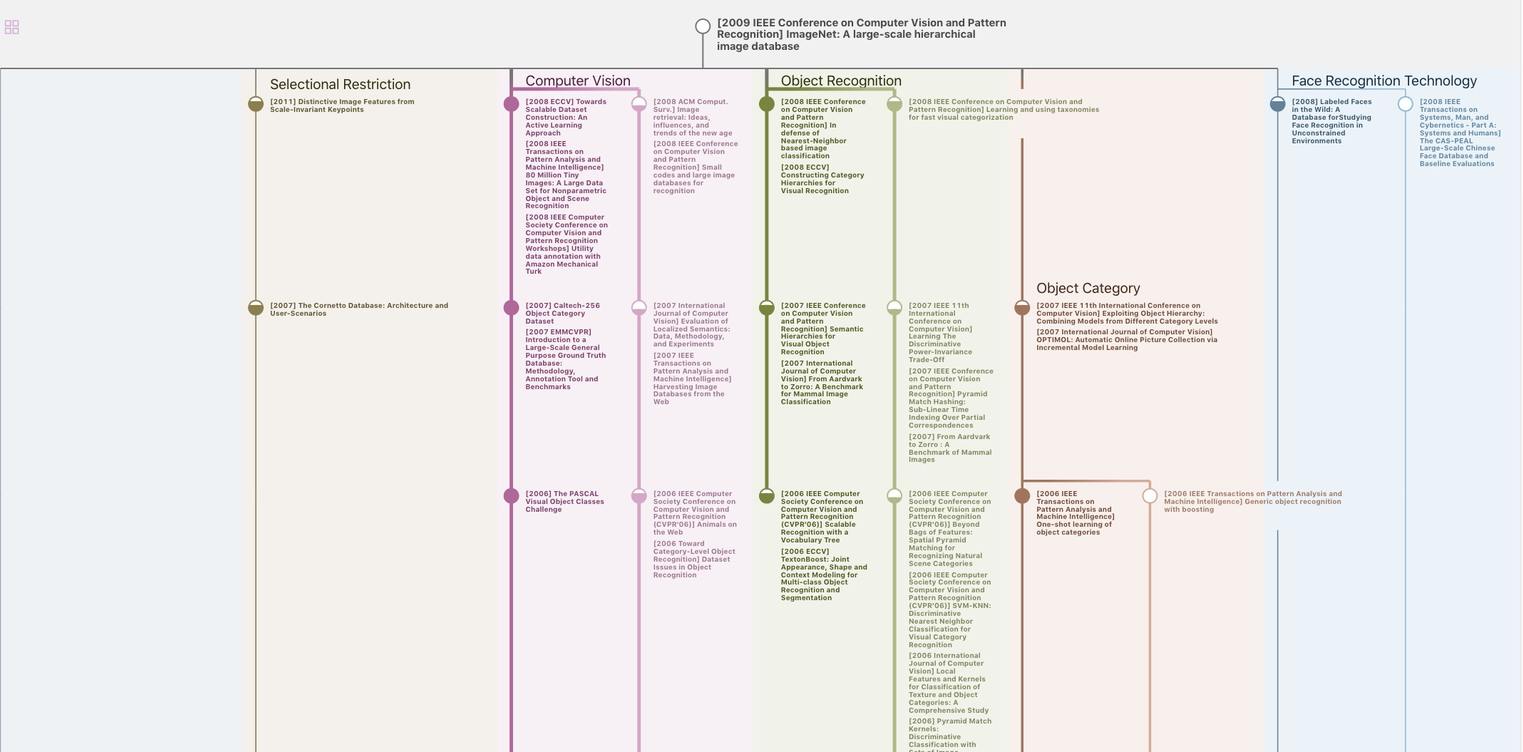
Generate MRT to find the research sequence of this paper
Chat Paper
Summary is being generated by the instructions you defined