CUPID: A labeled dataset with Pentesting for evaluation of network intrusion detection
Journal of Systems Architecture(2022)
摘要
Reproducibility of network intrusion detection research necessitates widely available datasets that represent real-world scenarios. One of the key omissions of existing datasets used in empirical evaluations of network intrusions is the lack of human-generated traffic with accurate labels to distinguish benign and malicious behavior. Using an emulated network environment with a vulnerable web application, we collected baseline traffic, human-generated normal user traffic, automated attacks, and the attacks of ten human penetration testers of varying abilities. We preprocessed this collected data to produce a new dataset named the Colorado University Pentesting Intrusion Dataset (CUPID). The attacks span from reconnaissance activities to delivery of an exploit payload. To our knowledge, this is the first collection that provides labeled, Institutional Review Board-approved, benign and attacker data that is publicly available. The CUPID dataset can be used to train and test the limits of classification-based machine learning algorithms used for network intrusion detection systems.
更多查看译文
关键词
Network intrusion detection,Feature selection
AI 理解论文
溯源树
样例
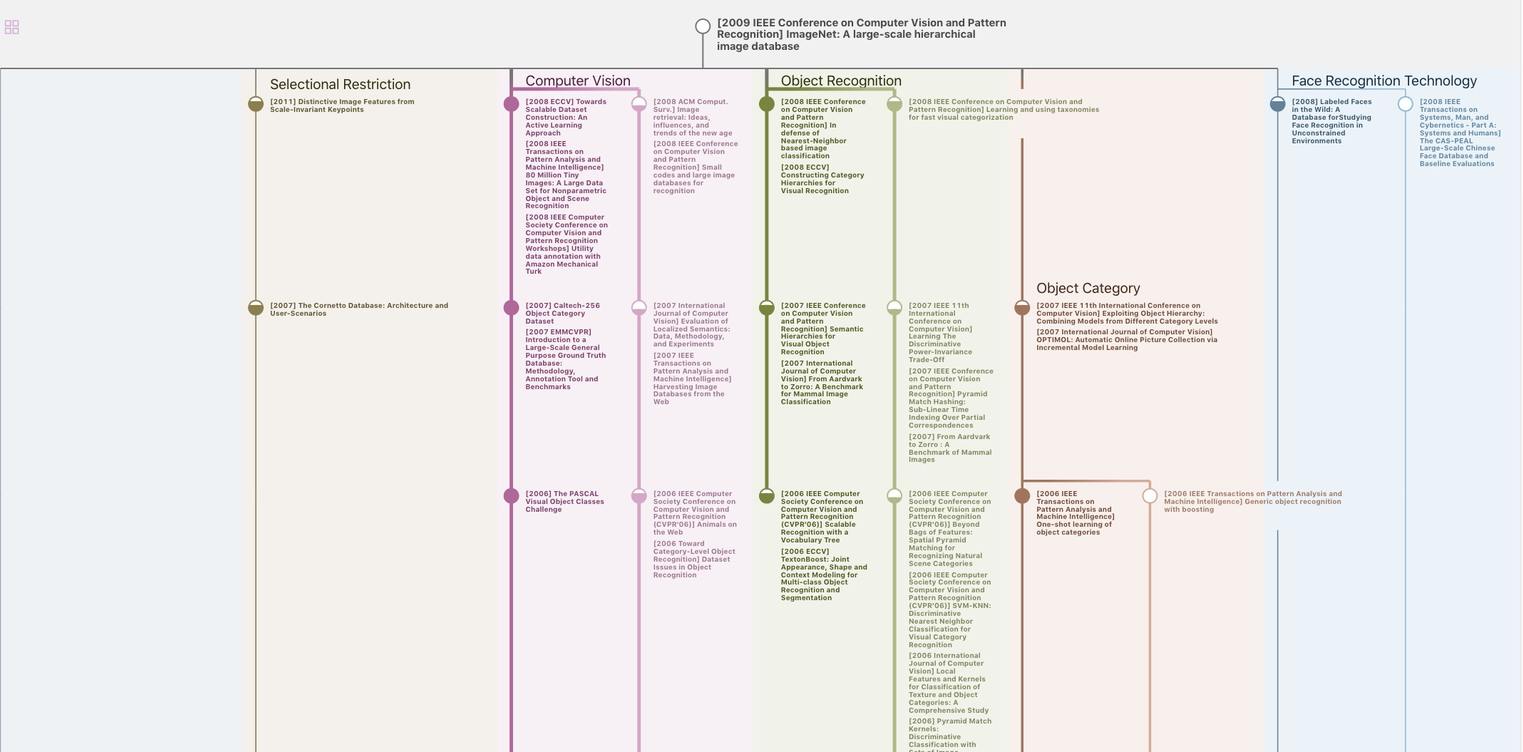
生成溯源树,研究论文发展脉络
Chat Paper
正在生成论文摘要