Factors affecting clinical outcomes after massive rotator cuff repair using a data mining technique
Journal of Shoulder and Elbow Surgery(2022)
摘要
A data mining technique using decision tree analysis is a method of discovery of new knowledge and patterns from big data. This study aimed to clarify by decision tree analysis the interaction among preoperative and postoperative prognostic factors that affect patient satisfaction after massive rotator cuff repairs (RCR). The study evaluated 1367 shoulders (right 956, left 411) with massive rotator cuff tears. Exclusion criteria were fracture, dislocation, re-tear, nerve paralysis, and insufficient follow-up. These patients were divided into two groups: satisfaction group and dissatisfaction group. We compared these groups with eight preoperative factors and two surgical factors using a decision tree analysis. Our results revealed three prognostic factors of patient satisfaction after massive RCR: tendon covering rate, preoperative ROM of flexion, and MMT. In clinical practice, decision tree analysis can be useful for understanding the interactions among predictors of patient satisfaction after massive RCR.
更多查看译文
关键词
massive rotator cuff repair,clinical outcomes
AI 理解论文
溯源树
样例
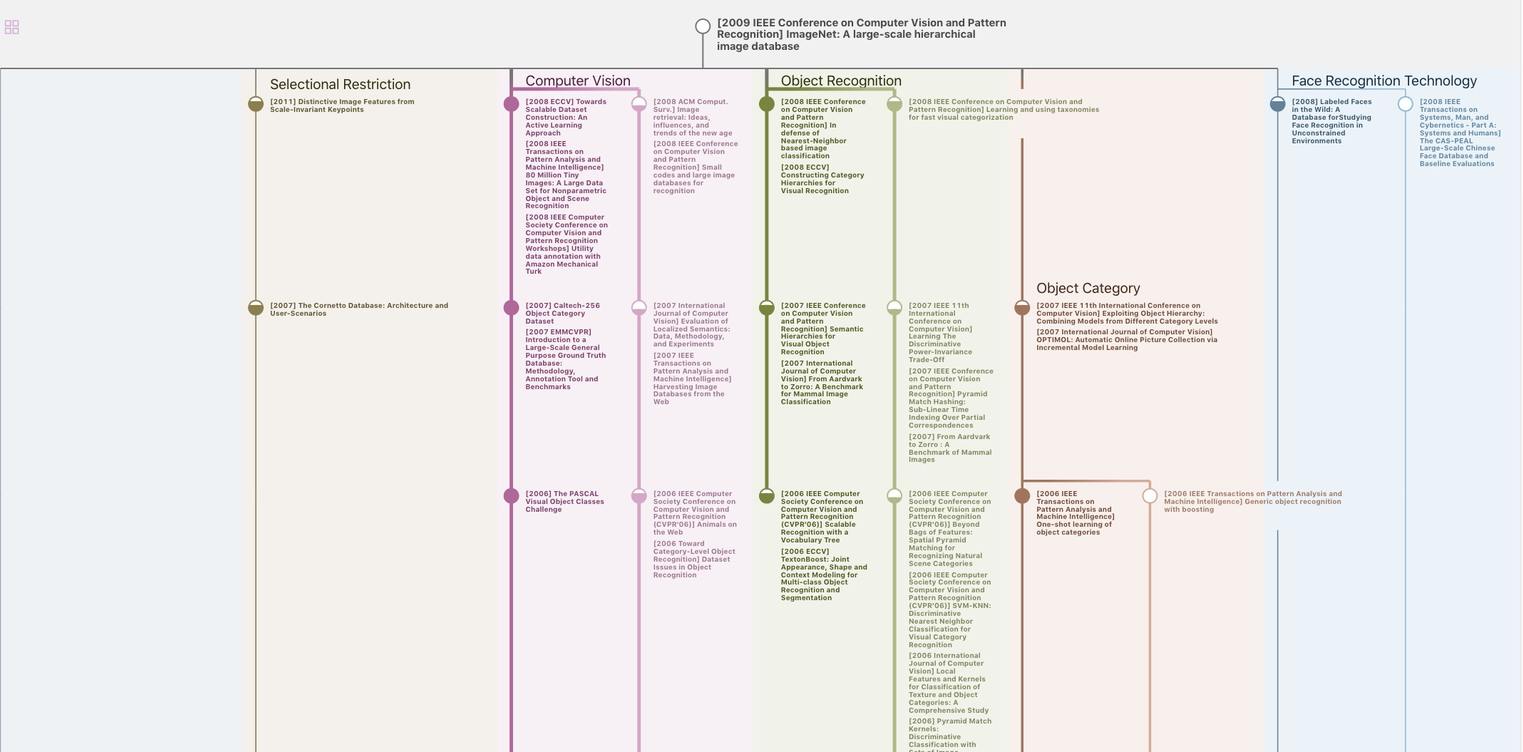
生成溯源树,研究论文发展脉络
Chat Paper
正在生成论文摘要