An improvement process of knowledge-based plan (KBP) model to standardize VMAT plan quality for prostate cancer
Journal of Medical Imaging and Radiation Sciences(2022)
Abstract
Purpose: The purpose of this study was to propose an improvement process of knowledge-based plan (KBP) models to standardize the VMAT plan quality for prostate cancer.Methods: The KBP models were updated at two times by setting each goal. First model was created from training plans at first phase (47 clinical IMRT/VMAT plans). Second model was created by training plans at second phase (50 consecutive new clinical VMAT plans) which exceeded the mean values +1SD of the dose–volume parameters of plans generated by first model as dosimetric goals. Third model was created by training plans at third phase (50 consecutive anew clinical VMAT plans) with more strict dose constraints for organs at risks (OARs) than first and second models. Each KBP model was verified for 30 validation plans (KBP1, KBP2, and KBP3) that were not used as training plans, and the dose–volume parameters were compared (D95 of target = prescribed dose of 78 Gy/39 fr). The Cook's distances of regression scatterplots of each model were also evaluated.Results: Significant differences (KBP1 vs. KBP2) were found in homogeneity index (3.90 vs. 4.44) and 95% isodose conformity index (1.22 vs. 1.20) for target, V40Gy (47.3% vs. 45.7%), V60Gy (27.9% vs. 27.1%), V70Gy (16.4% vs. 15.2%), and V78Gy (0.4% vs. 0.2%) for rectal wall, and V40Gy (43.8% vs. 41.8%) and V70Gy (21.3% vs. 20.5%) for bladder wall (p < 0.05). For KBP2 vs. KBP3, only V70Gy (15.2% vs. 15.8%) of rectal wall differed significantly. The proportions of cases with a Cook's distance of <1.0 (first, second, and third models) were 55%, 78%, and 84% for rectal wall, and 77%, 68%, and 76% for bladder wall, respectively.Conclusion: The iteratively improved KBP models could generate superior dose–volume parameters and converged regression scatterplots in the model. Our approach could be used to standardize the VMAT plan quality. Purpose: The purpose of this study was to propose an improvement process of knowledge-based plan (KBP) models to standardize the VMAT plan quality for prostate cancer. Methods: The KBP models were updated at two times by setting each goal. First model was created from training plans at first phase (47 clinical IMRT/VMAT plans). Second model was created by training plans at second phase (50 consecutive new clinical VMAT plans) which exceeded the mean values +1SD of the dose–volume parameters of plans generated by first model as dosimetric goals. Third model was created by training plans at third phase (50 consecutive anew clinical VMAT plans) with more strict dose constraints for organs at risks (OARs) than first and second models. Each KBP model was verified for 30 validation plans (KBP1, KBP2, and KBP3) that were not used as training plans, and the dose–volume parameters were compared (D95 of target = prescribed dose of 78 Gy/39 fr). The Cook's distances of regression scatterplots of each model were also evaluated. Results: Significant differences (KBP1 vs. KBP2) were found in homogeneity index (3.90 vs. 4.44) and 95% isodose conformity index (1.22 vs. 1.20) for target, V40Gy (47.3% vs. 45.7%), V60Gy (27.9% vs. 27.1%), V70Gy (16.4% vs. 15.2%), and V78Gy (0.4% vs. 0.2%) for rectal wall, and V40Gy (43.8% vs. 41.8%) and V70Gy (21.3% vs. 20.5%) for bladder wall (p < 0.05). For KBP2 vs. KBP3, only V70Gy (15.2% vs. 15.8%) of rectal wall differed significantly. The proportions of cases with a Cook's distance of <1.0 (first, second, and third models) were 55%, 78%, and 84% for rectal wall, and 77%, 68%, and 76% for bladder wall, respectively. Conclusion: The iteratively improved KBP models could generate superior dose–volume parameters and converged regression scatterplots in the model. Our approach could be used to standardize the VMAT plan quality.
MoreTranslated text
Key words
vmat plan quality,prostate cancer,kbp,knowledge-based
AI Read Science
Must-Reading Tree
Example
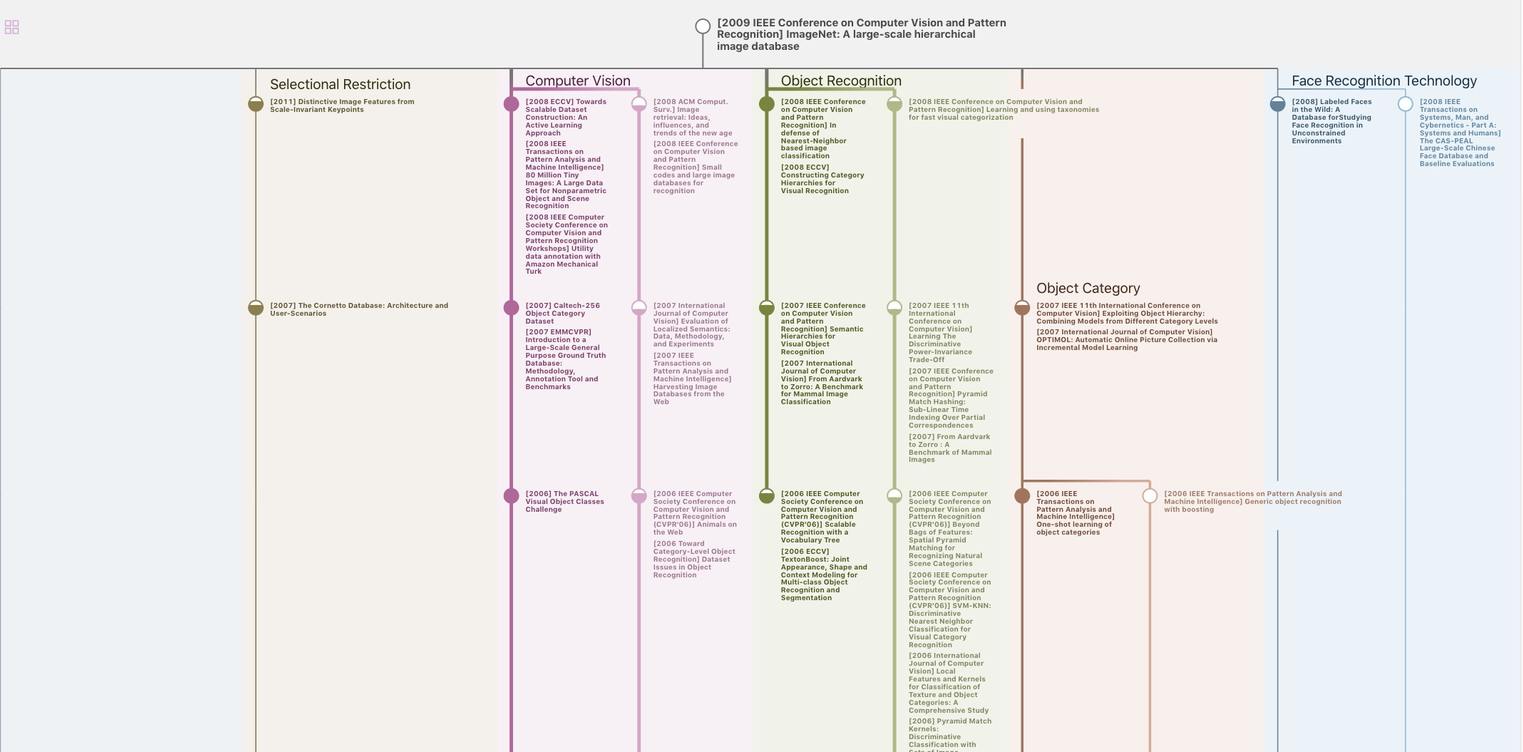
Generate MRT to find the research sequence of this paper
Chat Paper
Summary is being generated by the instructions you defined