Short-Term Online Forecasting for Passenger Origin–Destination (OD) Flows of Urban Rail Transit: A Graph–Temporal Fused Deep Learning Method
Mathematics(2022)
摘要
Predicting short-term passenger flow accurately is of great significance for daily management and for a timely emergency response of rail transit networks. In this paper, we propose an attention-based Graph–Temporal Fused Neural Network (GTFNN) that can make online predictions of origin–destination (OD) flows in a large-scale urban transit network. In order to solve the key issue of the passenger hysteresis in online flow forecasting, the proposed GTFNN takes finished OD flow and a series of features, which are known or observable, as the input and performs multi-step prediction. The model is constructed from capturing both spatial and temporal characteristics. For learning spatial characteristics, a multi-layer graph neural network is proposed based on hidden relationships in the rail transit network. Then, we embedded the graph convolution into a Gated Recurrent Unit to learn spatial–temporal features. For learning temporal characteristics, a sequence-to-sequence structure embedded with the attention mechanism is proposed to enhance its ability to capture both local and global dependencies. Experiments based on real-world data collected from Chongqing’s rail transit system show that the metrics of GTFNN are better than other methods, e.g., the SMAPE (Symmetric Mean Absolute Percentage Error) score is about 14.16%, with a range from 5% to 20% higher compared to other methods.
更多查看译文
关键词
urban rail system, short-term prediction, passenger origin-destination (OD), graph neural networks, graph-temporal fused, sequence to sequence
AI 理解论文
溯源树
样例
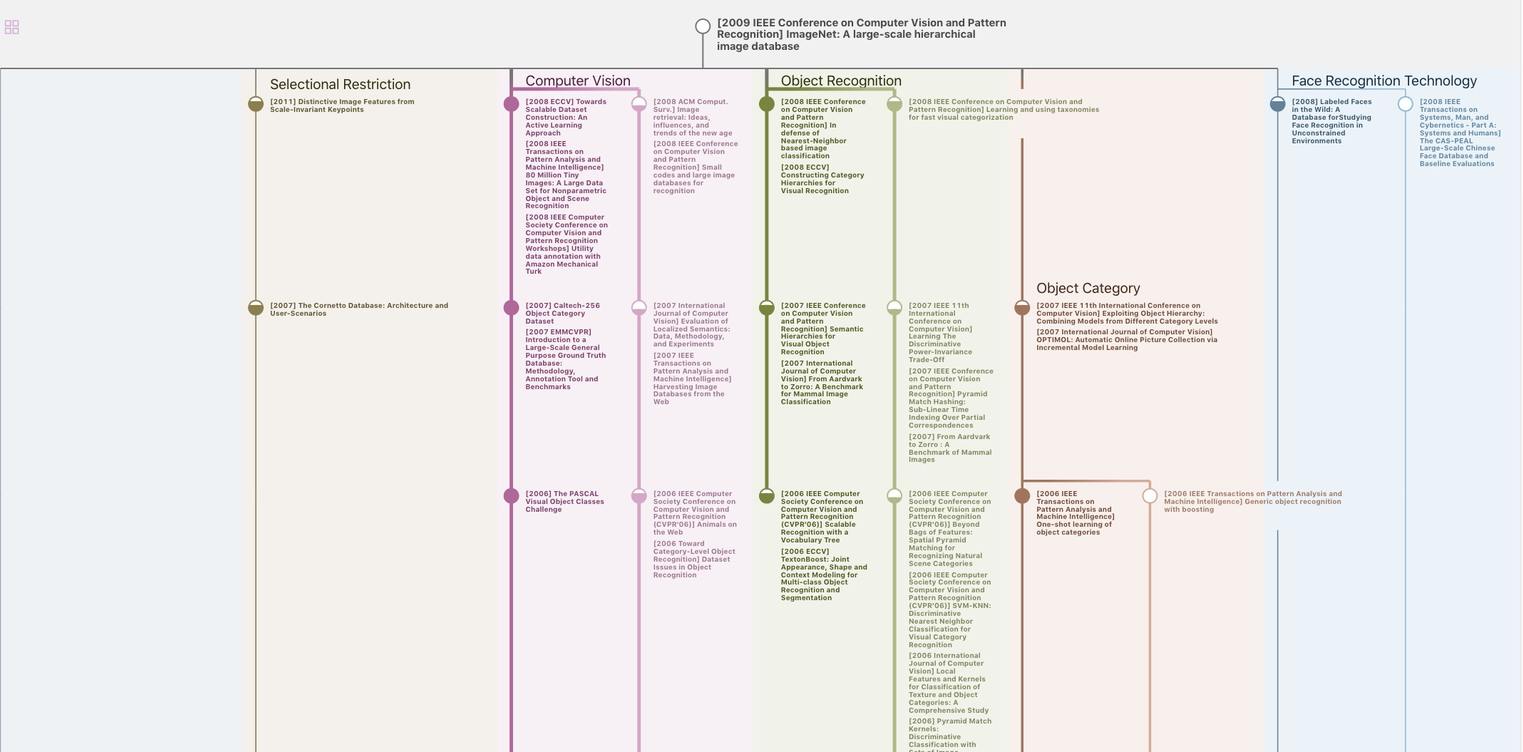
生成溯源树,研究论文发展脉络
Chat Paper
正在生成论文摘要