Hybrid Fine-Tuning Strategy for Few-Shot Classification
COMPUTATIONAL INTELLIGENCE AND NEUROSCIENCE(2022)
摘要
Few-shot classification aims to enable the network to acquire the ability of feature extraction and label prediction for the target categories given a few numbers of labeled samples. Current few-shot classification methods focus on the pretraining stage while fine-tuning by experience or not at all. No fine-tuning or insufficient fine-tuning may get low accuracy for the given tasks, while excessive fine-tuning will lead to poor generalization for unseen samples. To solve the above problems, this study proposes a hybrid fine-tuning strategy (HFT), including a few-shot linear discriminant analysis module (FSLDA) and an adaptive fine-tuning module (AFT). FSLDA constructs the optimal linear classification function under the few-shot conditions to initialize the last fully connected layer parameters, which fully excavates the professional knowledge of the given tasks and guarantees the lower bound of the model accuracy. AFT adopts an adaptive fine-tuning termination rule to obtain the optimal training epochs to prevent the model from overfitting. AFT is also built on FSLDA and outputs the final optimum hybrid fine-tuning strategy for a given sample size and layer frozen policy. We conducted extensive experiments on mini-ImageNet and tiered-ImageNet to prove the effectiveness of our proposed method. It achieves consistent performance improvements compared to existing fine-tuning methods under different sample sizes, layer frozen policies, and few-shot classification frameworks.
更多查看译文
关键词
classification,fine-tuning,few-shot
AI 理解论文
溯源树
样例
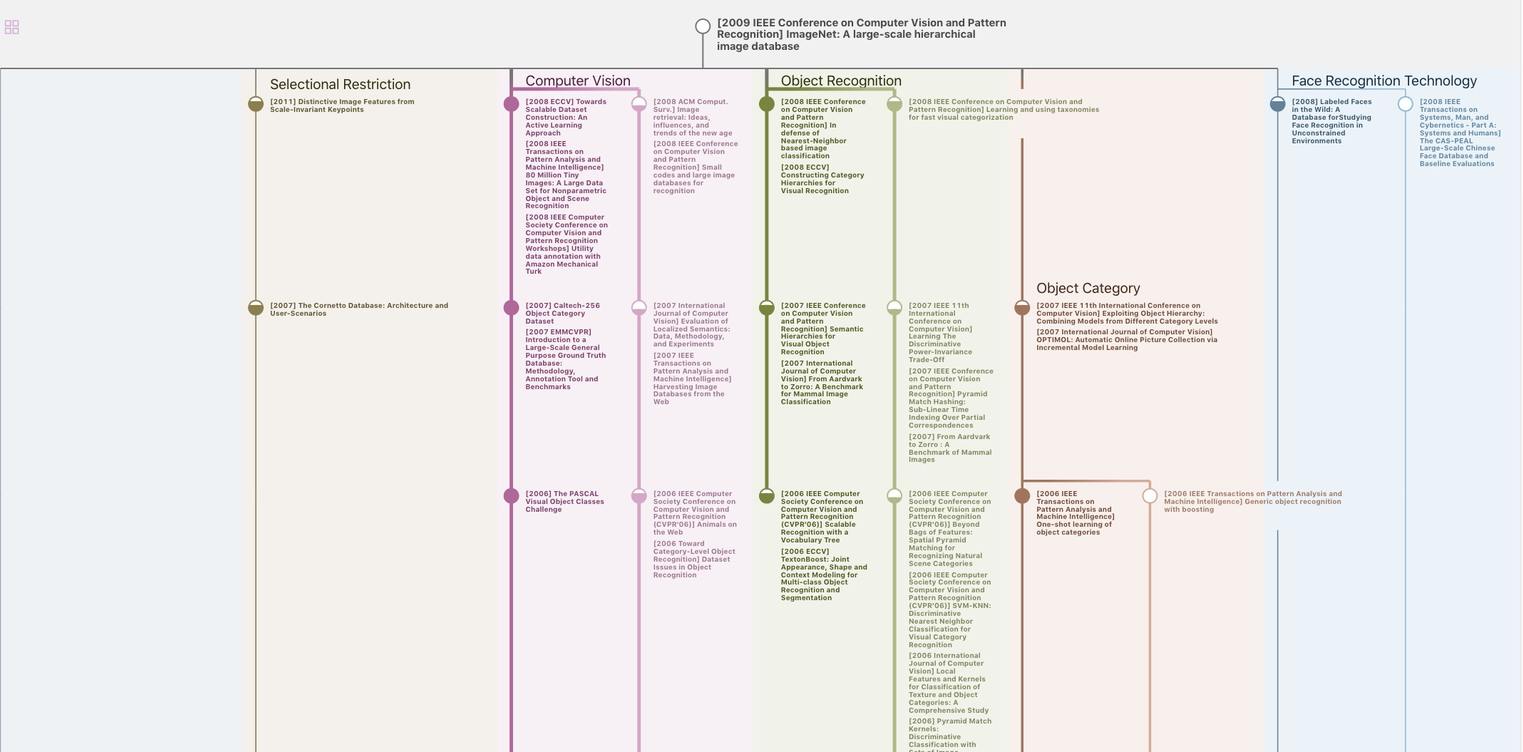
生成溯源树,研究论文发展脉络
Chat Paper
正在生成论文摘要