SkinSensPred as a Promising in Silico Tool for Integrated Testing Strategy on Skin Sensitization
INTERNATIONAL JOURNAL OF ENVIRONMENTAL RESEARCH AND PUBLIC HEALTH(2022)
Abstract
Skin sensitization is an important regulatory endpoint associated with allergic contact dermatitis. Recently, several adverse outcome pathway (AOP)-based alternative methods were developed to replace animal testing for evaluating skin sensitizers. The AOP-based assays were further integrated as a two-out-of-three method with good predictivity. However, the acquisition of experimental data is resource-intensive. In contrast, an integrated testing strategy (ITS) capable of maximizing the usage of laboratory data from AOP-based and in silico methods was developed as defined approaches (DAs) to both hazard and potency assessment. There are currently two in silico models, namely Derek Nexus and OECD QSAR Toolbox, evaluated in the OECD Testing Guideline No. 497. Since more advanced machine learning algorithms have been proposed for skin sensitization prediction, it is therefore desirable to evaluate their performance under the ITS framework. This study evaluated the performance of a new ITS DA (ITS-SkinSensPred) adopting a transfer learning-based SkinSensPred model. Results showed that the ITS-SkinSensPred has similar or slightly better performance compared to the other ITS models. SkinSensPred-based ITS is expected to be a promising method for assessing skin sensitization.
MoreTranslated text
Key words
adverse outcome pathway,skin sensitization,machine learning,SkinSensPred,3R
AI Read Science
Must-Reading Tree
Example
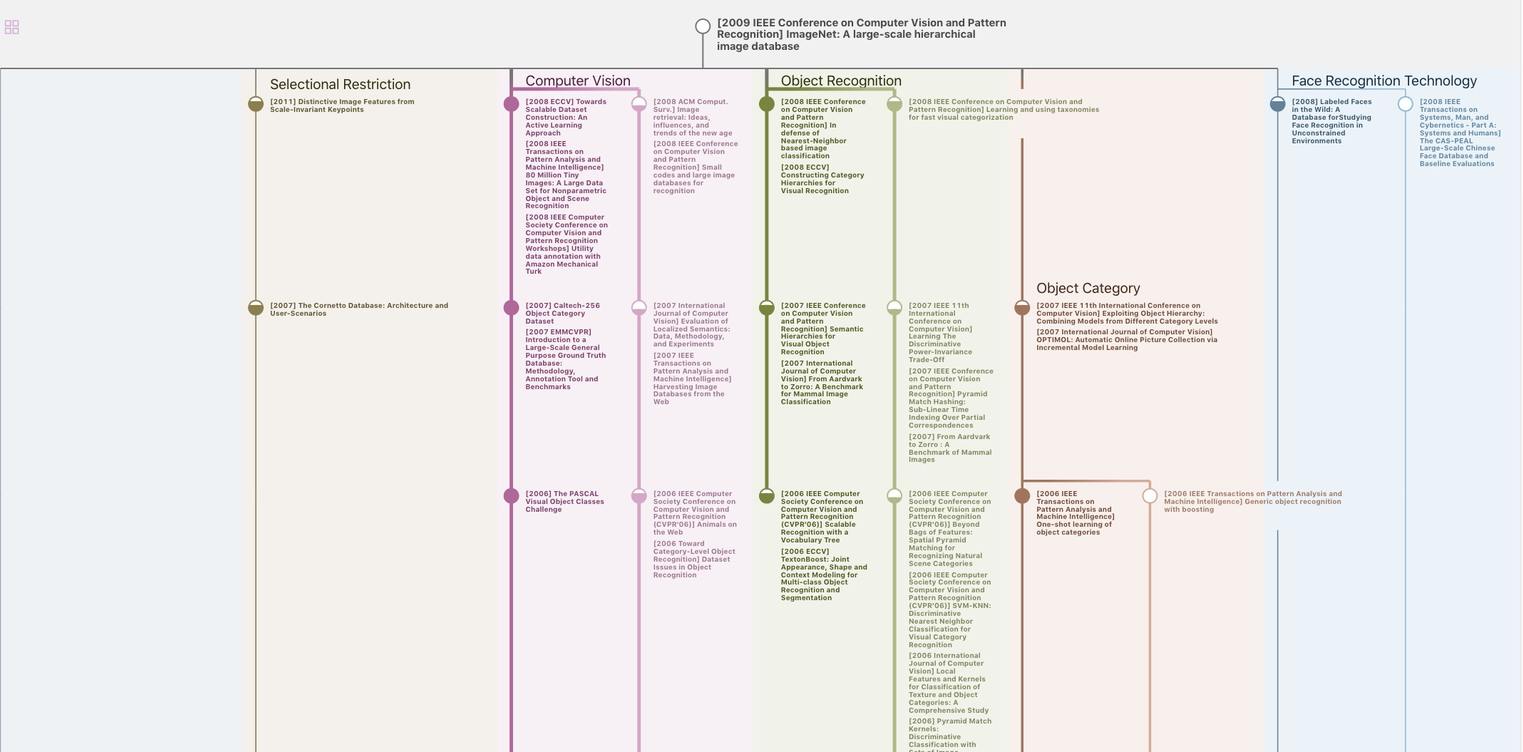
Generate MRT to find the research sequence of this paper
Chat Paper
Summary is being generated by the instructions you defined