Polyconvex neural networks for hyperelastic constitutive models: A rectification approach
Mechanics Research Communications(2022)
摘要
A simple approach to rectify unconstrained neural networks for hyperelastic constitutive models is proposed with the aim of ensuring both mathematical well-posedness (in terms of existence theorems) and physical consistency. The surrogate involves neural networks that are made admissible by selecting a proper parameterization, following standard results in continuum mechanics, and by enforcing polyconvexity through integral representations. The relevance of the formulation is demonstrated by considering digitally synthesized and experimental datasets for isotropic and anisotropic materials, including the case of soft biological tissues.
更多查看译文
关键词
Constitutive modeling,Hyperelasticity,Neural networks,Polyconvexity,Rectification
AI 理解论文
溯源树
样例
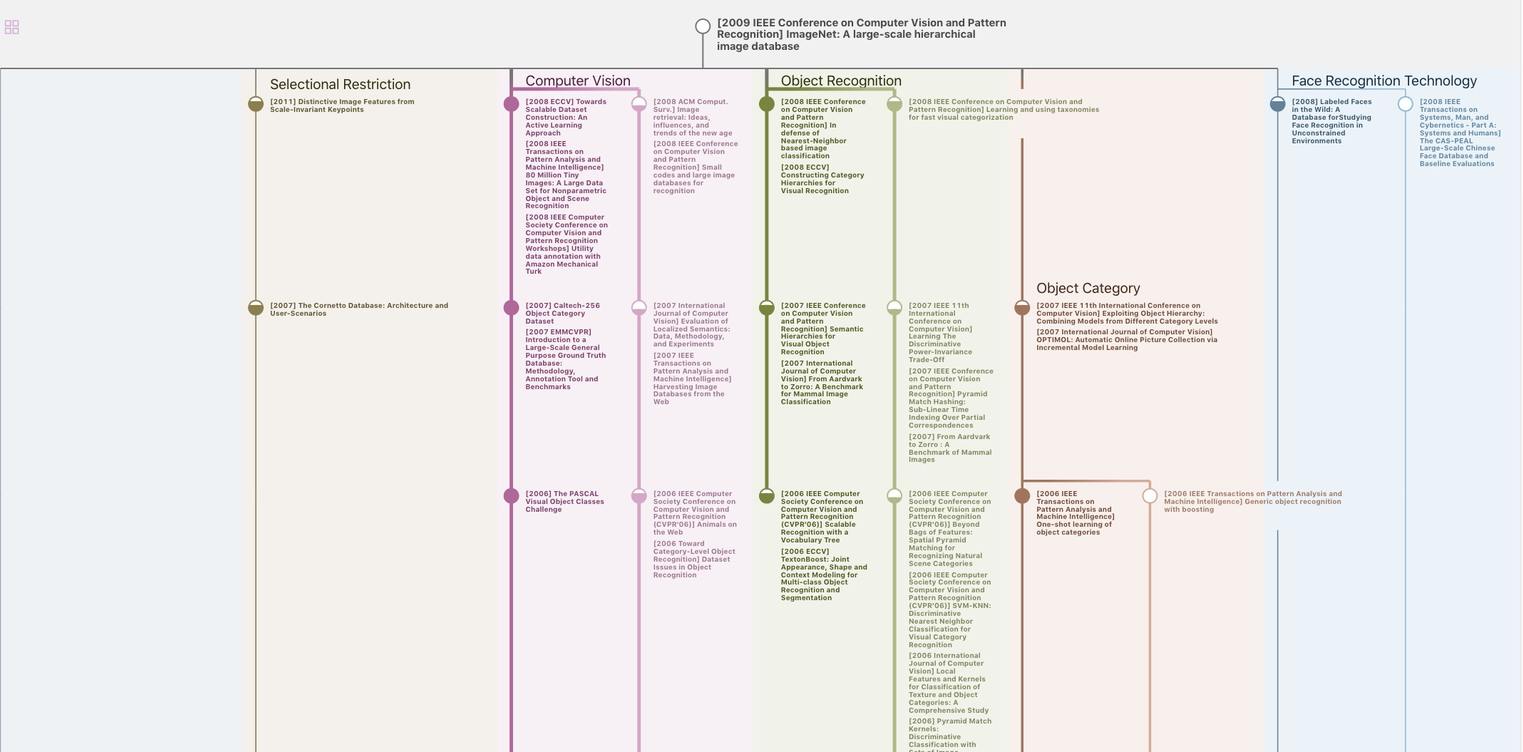
生成溯源树,研究论文发展脉络
Chat Paper
正在生成论文摘要