A data-driven framework to manage uncertainty due to limited transferability in urban growth models
Computers, Environment and Urban Systems(2022)
Abstract
The processes of urban growth vary in space and time. There is a lack of model transferability, which means that models estimated for a particular study area and period are not necessarily applicable for other periods and areas. This problem is often addressed through scenario analysis, where scenarios reflect different plausible model realisations based typically on expert consultation. This study proposes a novel framework for data-driven scenario development which, consists of three components - (i) multi-area, multi-period calibration, (ii) growth mode clustering, and (iii) cross-application. The framework finds clusters of parameters, referred to as growth modes: within the clusters, parameters represent similar spatial development trajectories; between the clusters, parameters represent substantially different spatial development trajectories. The framework is tested with a stochastic dynamic urban growth model across European functional urban areas over multiple time periods, estimated using a Bayesian method on an open global urban settlement dataset covering the period 1975–2014.
MoreTranslated text
Key words
Transferability,Scenario development,Uncertainty,Non-stationarity,Urban growth modes,Cellular automata land-use change models
AI Read Science
Must-Reading Tree
Example
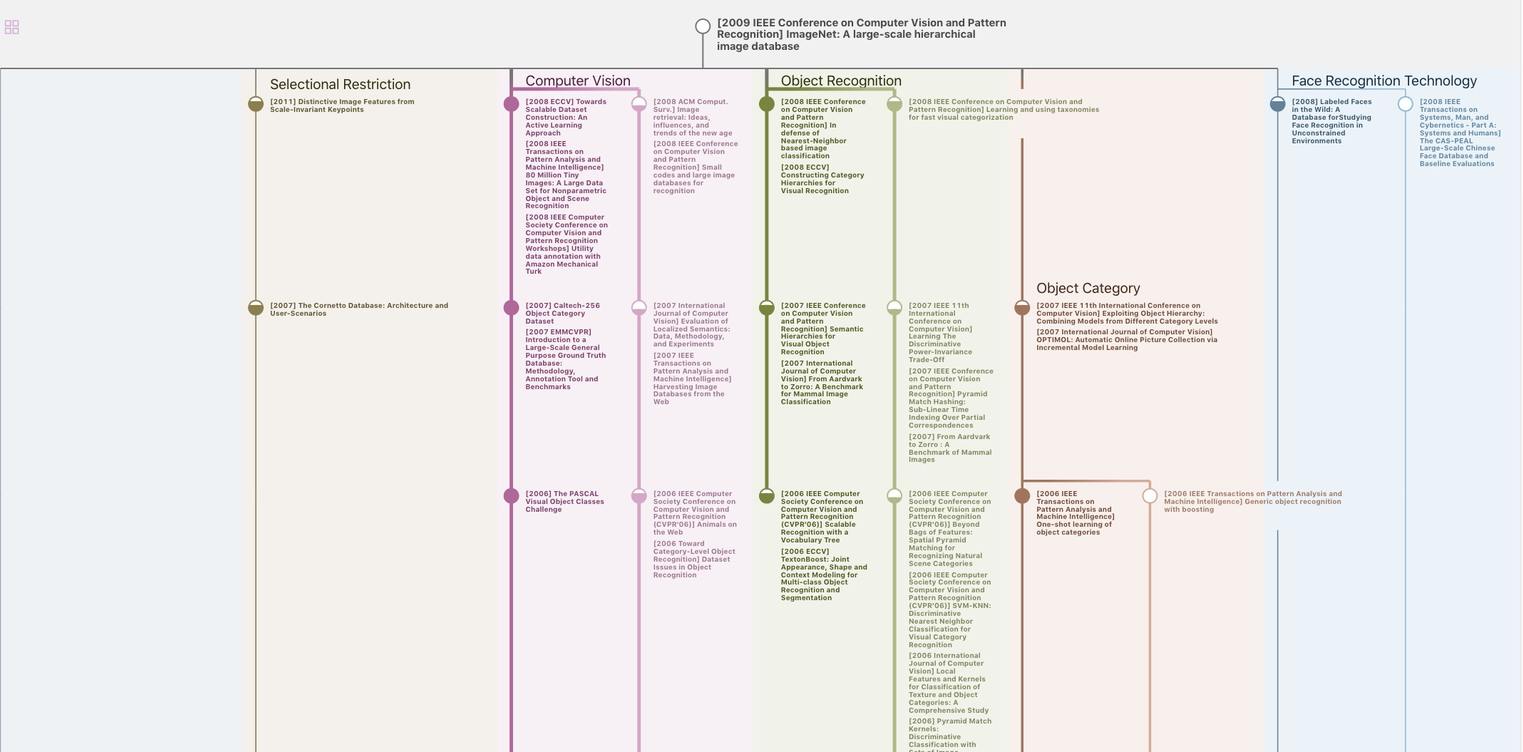
Generate MRT to find the research sequence of this paper
Chat Paper
Summary is being generated by the instructions you defined