Multi-output regression with structurally incomplete target labels: A case study of modelling global vegetation cover.
Ecol. Informatics(2022)
摘要
Weakly-supervised learning has recently emerged in the classification context where true labels are often scarce or unreliable. However, this learning setting has not yet been extensively analyzed for regression problems, which are typical in macroecology. We further define a novel computational setting of structurally noisy and incomplete target labels, which arises, for example, when the multi-output regression task defines a distribution such that outputs must sum up to unity. We propose an algorithmic approach to reduce noise in the target labels and improve predictions. We evaluate this setting with a case study in global vegetation modelling, which in-volves building a model to predict the distribution of vegetation cover from climatic conditions based on global remote sensing data. We compare the performance of the proposed approach to several incomplete target baselines. The results indicate that the error in the targets can be reduced by our proposed partial-imputation algorithm. We conclude that handling structural incompleteness in the target labels instead of using only complete observations for training helps to better capture global associations between vegetation and climate.
更多查看译文
关键词
Multi -output regression,Compositional data,Incomplete targets,Weakly supervised learning,Vegetation cover modelling
AI 理解论文
溯源树
样例
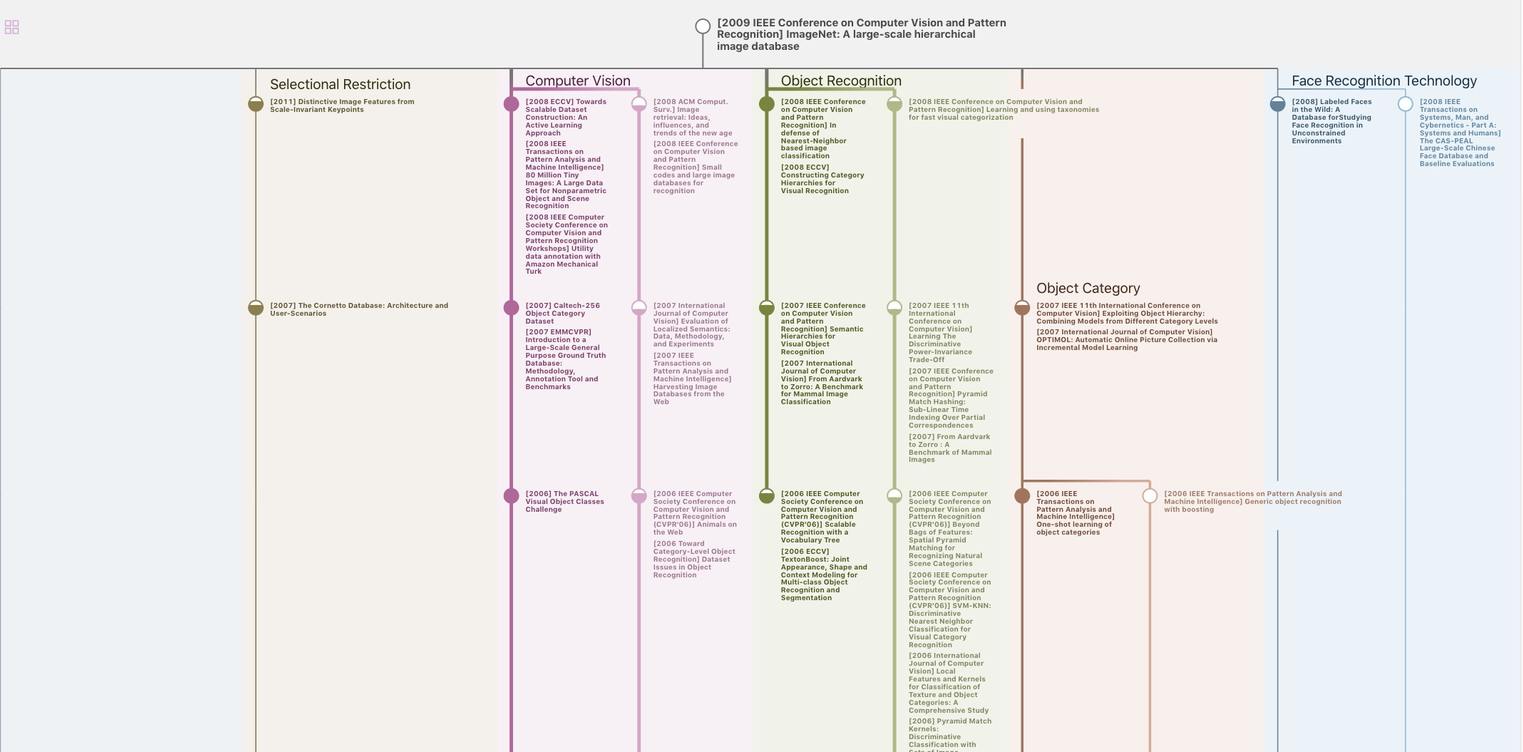
生成溯源树,研究论文发展脉络
Chat Paper
正在生成论文摘要