Image enhancement of ultra-low dose CBCT images using a deep generative model
Oral Surgery, Oral Medicine, Oral Pathology and Oral Radiology(2022)
摘要
Objective The aim of this study was to improve the image quality of CBCT images, obtained with an ultra-low dose (ULD) protocol, using a deep generative model called pix2pix image-to-image translation technique. Study Design CBCT images of a dry skull and mandible were obtained using preset standard and ULD imaging protocols. We combined the scans into a dataset with 1,412 ULD scans and normal-dose DICOM image pairs (2,824 scans in total), and randomly selected 10% (140) image pairs as the test set. We trained a pix2pix deep generative model by first converting the DICOM images to JPG images and then feeding the training set image pairs to the network. We tested the performance of the trained model on the previously unseen test set ULD images and compared the synthesized images with the corresponding normal dose and ULD images. A preliminary blind study on the ULD, standard, and synthesized images by an oral and maxillofacial radiologist was done and image quality and edge definition were assessed. Results Preliminary results indicated that in the three datasets used for review, all synthesized images were rated as having higher image quality and better edge definition compared to ULD images. Analysis also indicated that synthesized images were equivalent to standard images in image quality and edge definition. Conclusion CBCT usage is expected to grow globally by 9.7%. With the expected growth, reducing radiation dose and improving image quality is of paramount importance to reduce the radiation burden on the population. The current study focused on image enhancement of CBCT images acquired with the ULD protocol. Initial analysis indicated a marked improvement in image quality of the synthesized images when compared to ULD images. With further research and successful implementation, we have an opportunity to reduce radiation risks to patients while improving image quality. Statement of Ethical Review Ethical Review or exemption was not warranted for this study. The aim of this study was to improve the image quality of CBCT images, obtained with an ultra-low dose (ULD) protocol, using a deep generative model called pix2pix image-to-image translation technique. CBCT images of a dry skull and mandible were obtained using preset standard and ULD imaging protocols. We combined the scans into a dataset with 1,412 ULD scans and normal-dose DICOM image pairs (2,824 scans in total), and randomly selected 10% (140) image pairs as the test set. We trained a pix2pix deep generative model by first converting the DICOM images to JPG images and then feeding the training set image pairs to the network. We tested the performance of the trained model on the previously unseen test set ULD images and compared the synthesized images with the corresponding normal dose and ULD images. A preliminary blind study on the ULD, standard, and synthesized images by an oral and maxillofacial radiologist was done and image quality and edge definition were assessed. Preliminary results indicated that in the three datasets used for review, all synthesized images were rated as having higher image quality and better edge definition compared to ULD images. Analysis also indicated that synthesized images were equivalent to standard images in image quality and edge definition. CBCT usage is expected to grow globally by 9.7%. With the expected growth, reducing radiation dose and improving image quality is of paramount importance to reduce the radiation burden on the population. The current study focused on image enhancement of CBCT images acquired with the ULD protocol. Initial analysis indicated a marked improvement in image quality of the synthesized images when compared to ULD images. With further research and successful implementation, we have an opportunity to reduce radiation risks to patients while improving image quality.
更多查看译文
关键词
image enhancement,images,ultra-low
AI 理解论文
溯源树
样例
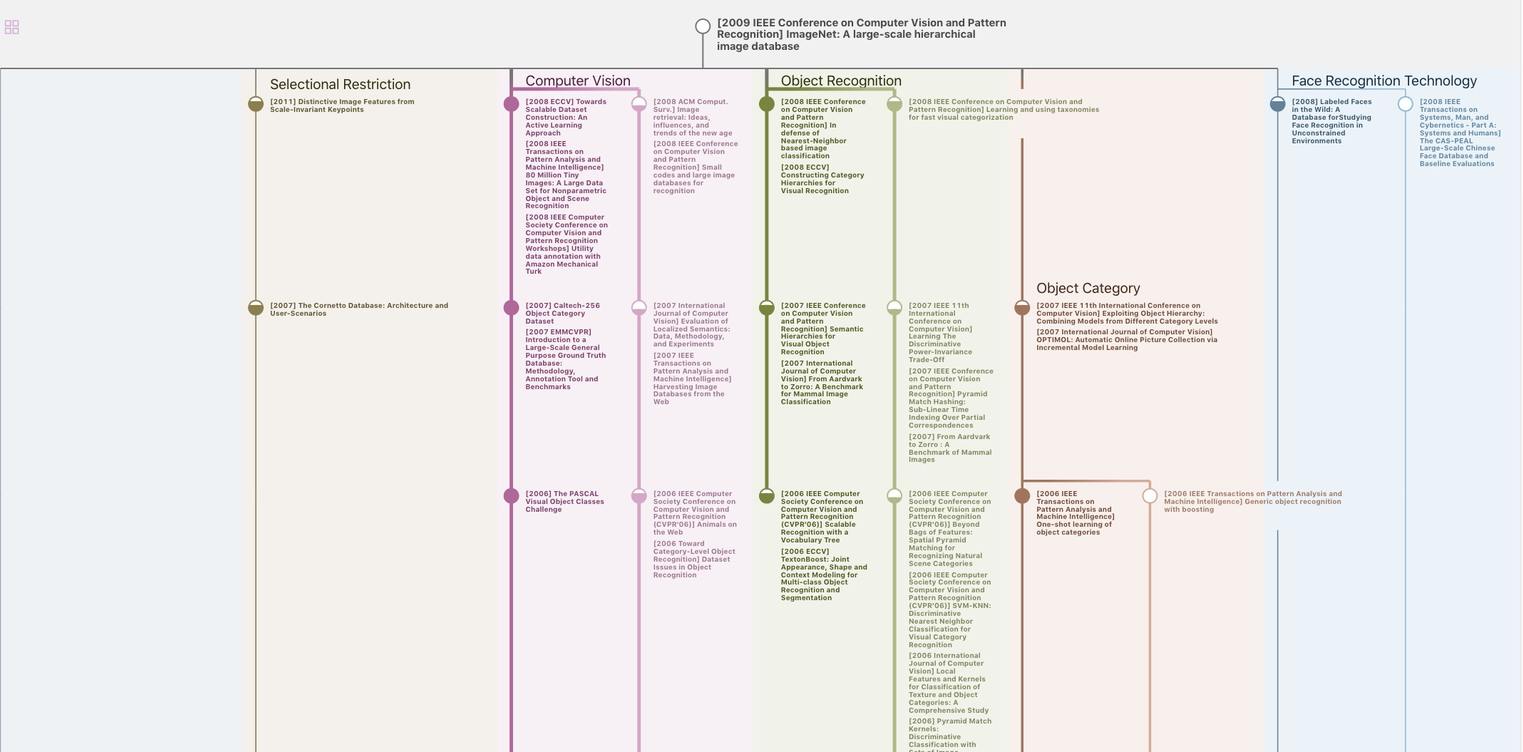
生成溯源树,研究论文发展脉络
Chat Paper
正在生成论文摘要