A motor bearing fault diagnosis method based on multi-source data and one-dimensional lightweight convolution neural network.
J. Syst. Control. Eng.(2023)
摘要
Deep learning is widely adopted in the field of fault diagnosis because of its powerful feature representation capabilities. The existing diagnosis methods are always proposed based on the data only from a single source and are susceptible to interference. Due to the need to convertthe data into two dimensions, data preprocessing also requires a lot of time. To address these problems, a new fault diagnosis algorithm based on multi-source data and one-dimensional lightweight convolutional neural network is presented. In particular, original data can be exploited directly without conversion to two dimensions. To enhance data fusion, attention module and channel shuffle module are added. Besides, to improve the utilization of data, a modified [Formula: see text] criterion is proposed to remove outliers in multi-source data. The performance of the proposed method in multi-source data fusion is verified in bearing failure experiments. Compared with the existing diagnosis methods (one-dimensional convolutional neural network, ResNet, ShuffleNet, MobileNet, EfficientNet and the two recurrence methods), multi-source data and one-dimensional lightweight convolutional neural network does a better job of balancing the efficiency and robustness.
更多查看译文
关键词
Motor fault diagnosis,multi-source data fusion,deep learning,lightweight network,1D CNN
AI 理解论文
溯源树
样例
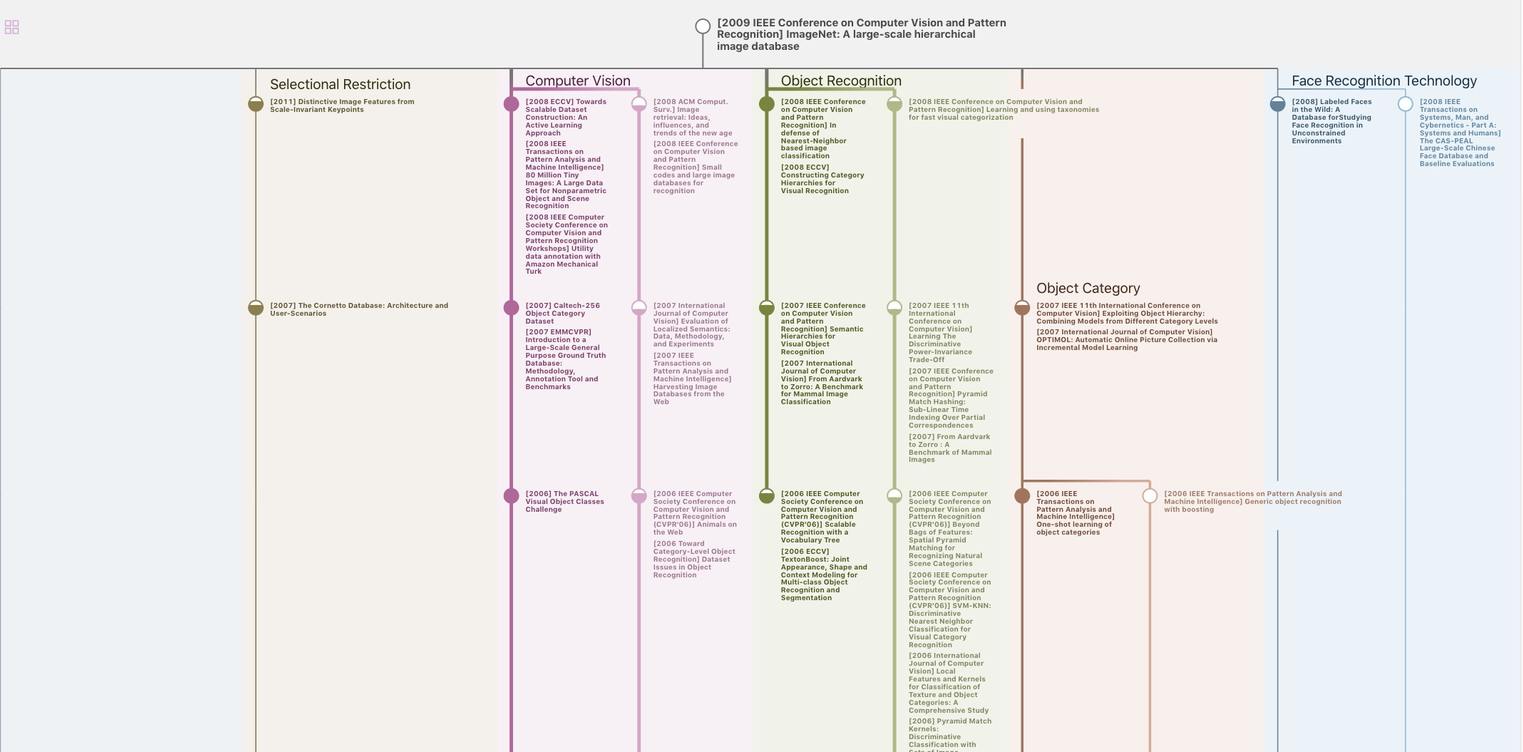
生成溯源树,研究论文发展脉络
Chat Paper
正在生成论文摘要