Gated spatial-temporal graph neural network based short-term load forecasting for wide-area multiple buses
International Journal of Electrical Power & Energy Systems(2023)
摘要
Existing short-term bus load forecasting methods mostly use temporal domain features, such as historical loads, to forecast and do not fully consider the influence of unstructured spatial-temporal coupling correlations among multiple bus loads in a wide spatial area on the forecasting results. In this paper, a wide-area multiple bus loads forecasting model is proposed. First, take the rapid computation of the maximal information coefficient (Rap-idMIC) value between bus loads as edge features, and combine the multiple node feature set to construct a similar-weighted spatial-temporal graph. The constructed similar-weighted spatial-temporal graph is not con-strained by geography and grid topology, and can directly reflect the degree of spatial-temporal coupling cor-relation between bus loads. Second, the neighboring node features of each node in the graph are extracted by the spatial convolution layer (SCL) to achieve full-domain node feature enhancement. Finally, the features extracted were formed into temporal series and input to the gated recurrent unit layer (GRUL) to achieve a wide-area multiple buses short-term load forecast. The research was carried out by selecting the measured load data (15 busbars). The corresponding symmetric mean absolute percentage error (SMAPE) and mean absolute error (MAE) are 3.19 % to 5.89 % and 1.83 megawatts (MW) to 9.8 MW, respectively. Compared with the SMAPE of other methods, the worst evaluation metrics in the test set are improved by 1.82 % to 5.94 %, and it has better robustness in the scene with abnormal load data.
更多查看译文
关键词
Wide -area multiple buses,Short-term load forecasting,Spatial -temporal graph convolutional neural,network,Similar -weighted spatial -temporal graph
AI 理解论文
溯源树
样例
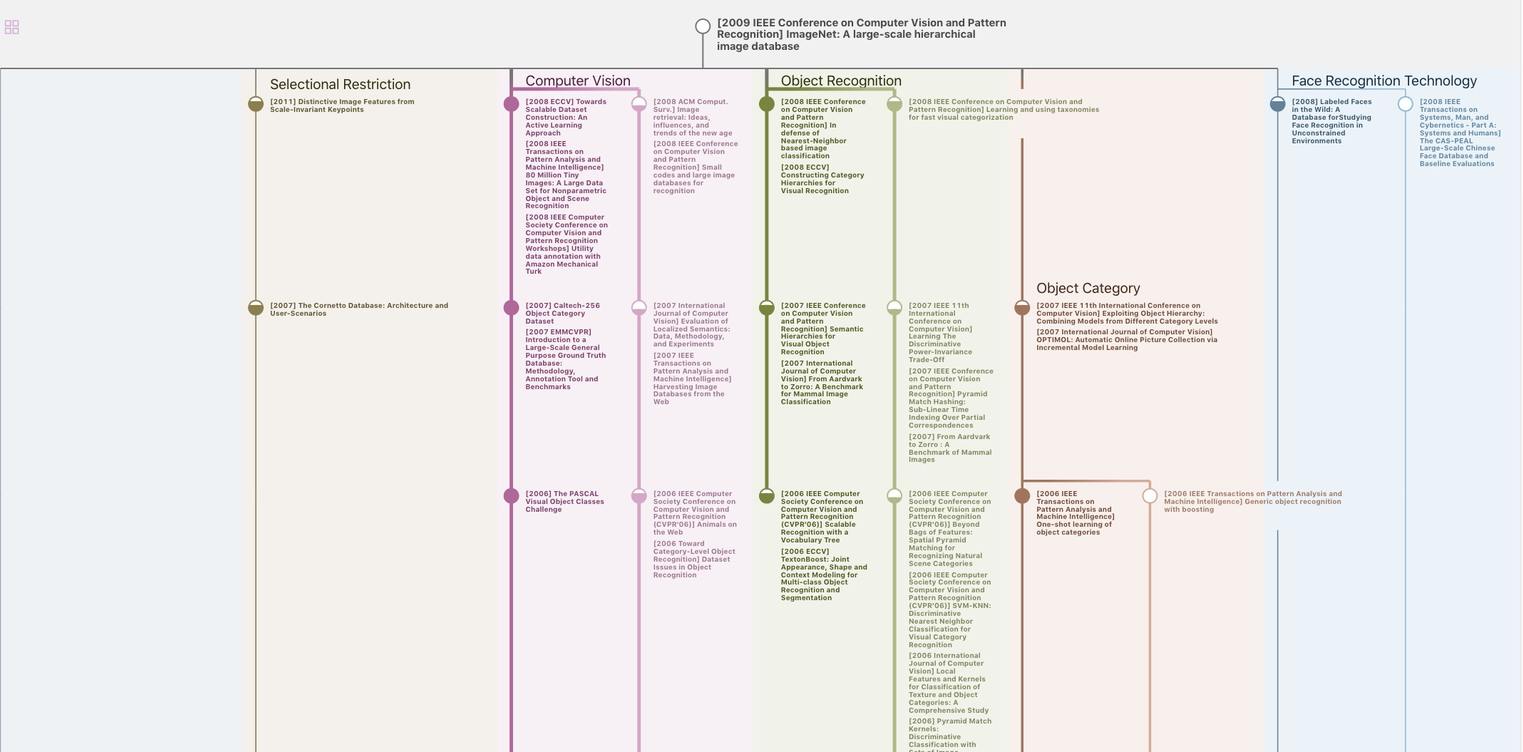
生成溯源树,研究论文发展脉络
Chat Paper
正在生成论文摘要