ST-MGAT:Spatio-temporal multi-head graph attention network for Traffic prediction
Physica A: Statistical Mechanics and its Applications(2022)
摘要
Traffic flow prediction is an important part of the intelligent transportation system, and accurate traffic flow prediction can better perform the data management and control of the urban road network. Traditional methods often ignore the interaction between traffic flow factors and the spatiotemporal dependence of traffic networks. In this paper, a spatiotemporal multi-head graph attention network (ST-MGAT) is proposed for the traffic flow prediction task. In the input layer, multiple traffic flow variables are used as input to learn the nonlinearity and complexity existing in it. In terms of modeling, the structure of linear gating units is transformed using the full volume and applied to correlation learning in the temporal dimension. The spatial dimension features are then captured using a multi-head attention map network. Ablation experiments are designed to verify the use effect of each module. At the same time, several sets of parameter experiments are designed to obtain the best model parameters. The experimental results show that compared with the baselines, the RMSE of the ST-MGAT model is decreased by almost 2.340%∼22.471%, and the MAE is decreased by almost 7.417%∼26.142%. It is proved that the ST-MGAT model has high performance on the traffic flow prediction task.
更多查看译文
关键词
Traffic engineering,Traffic flow prediction model,GAT model,GLU model,Traffic flow
AI 理解论文
溯源树
样例
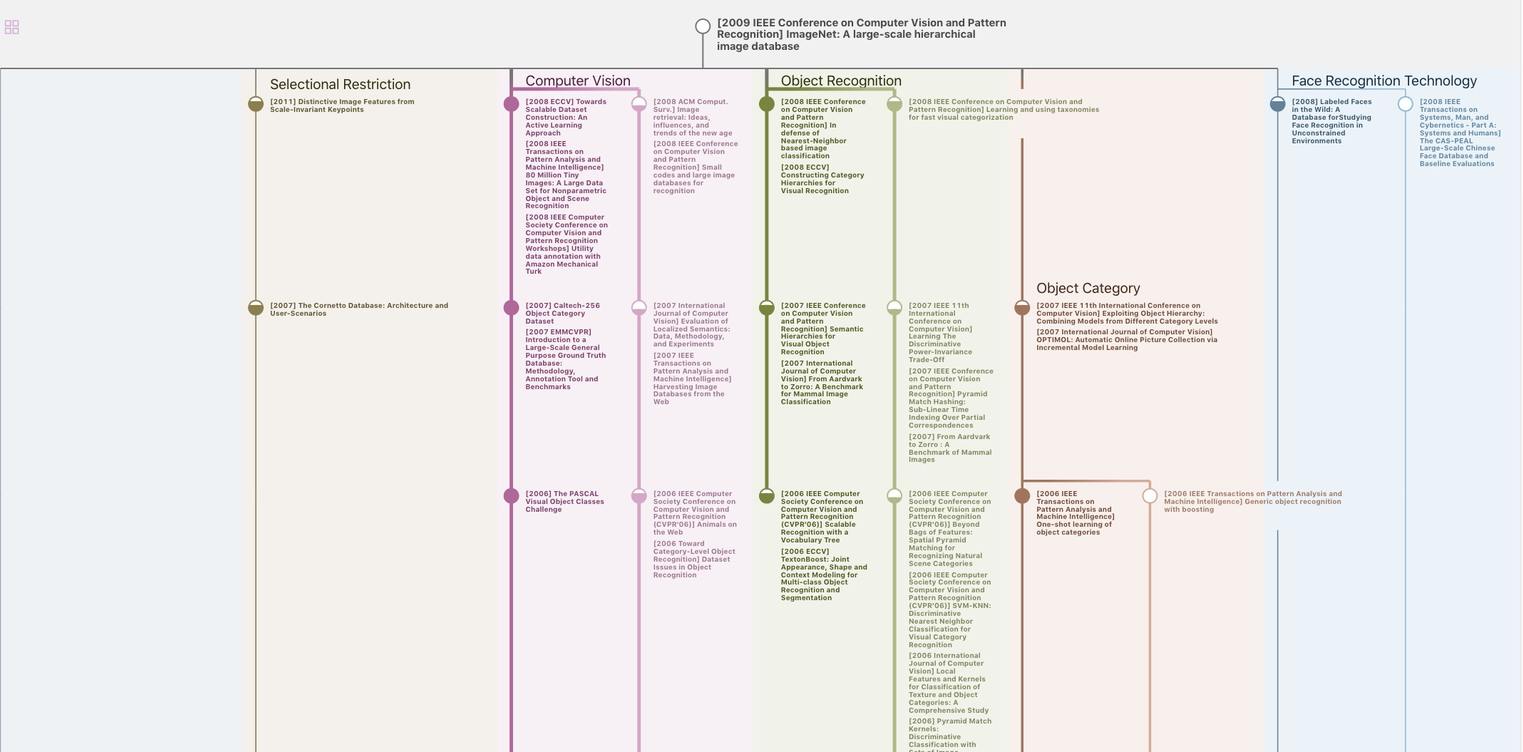
生成溯源树,研究论文发展脉络
Chat Paper
正在生成论文摘要