Year-ahead Ambient Temperature Forecasting in Pharmaceutical Transport Lanes Thermal Conditions
Procedia Computer Science(2022)
摘要
This research aims to predict year-ahead ambient temperature aggregated at monthly level per hour on airport locations using historical temperature data. In this research, an Extreme gradient boosting regression model, LSTM model and the benchmark model, a persistence model, are compared in accuracy. Models are fine-tuned on the cities with the highest variability in temperature and grid searches are implemented only for these cities (one per data source). Overall, we have seen that the LSTM model with output size 12 months x 24 hours predicts for the next year the best. The Persistence model is closely followed by the Extreme gradient boosting model, with a small deviation in the quantiles. The point predictions for each of the other models are a bit further of (with more than 3 degrees Celsius) and LSTM 365 days x 24 hours is the worst in this case. These models can be used to give an indication for the ambient temperature on lane level.
更多查看译文
关键词
Ambient temperature,Forecasting,Pharmaceutical products,Packaging,Air cargo,Tarmac,LSTM,Persistence model,Extreme gradient boosting
AI 理解论文
溯源树
样例
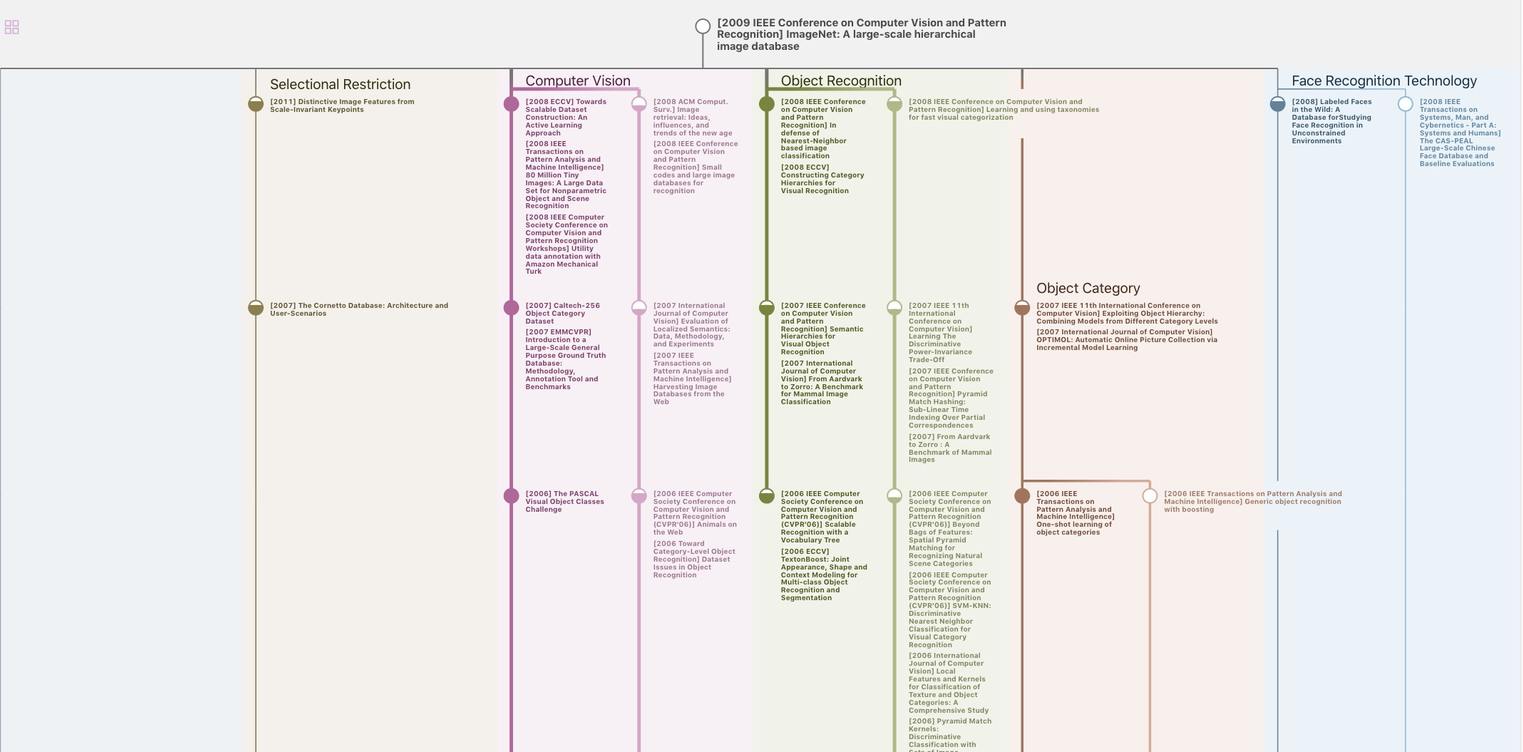
生成溯源树,研究论文发展脉络
Chat Paper
正在生成论文摘要