Multi-agent-based deep reinforcement learning for dynamic flexible job shop scheduling
Procedia CIRP(2022)
摘要
In disruption-prone manufacturing environments, flexible job shop scheduling becomes a dynamic problem. For achieving a high solution quality, operations research approaches can be applied. In contrast, due to the required fast response times, dispatching rules are the standard. In order to elaborate on both, we present a new deep reinforcement learning algorithm. It combines policy gradient algorithms with actor-critic architectures and interprets the production system as a multi-agent system. Our evaluation on benchmark instances shows that the algorithm generates better schedules than dispatching rules with the same response time. It also generalizes well when tested on different manufacturing environments.
更多查看译文
关键词
production planning,production control,artificial intelligence,machine learning,cyber production management
AI 理解论文
溯源树
样例
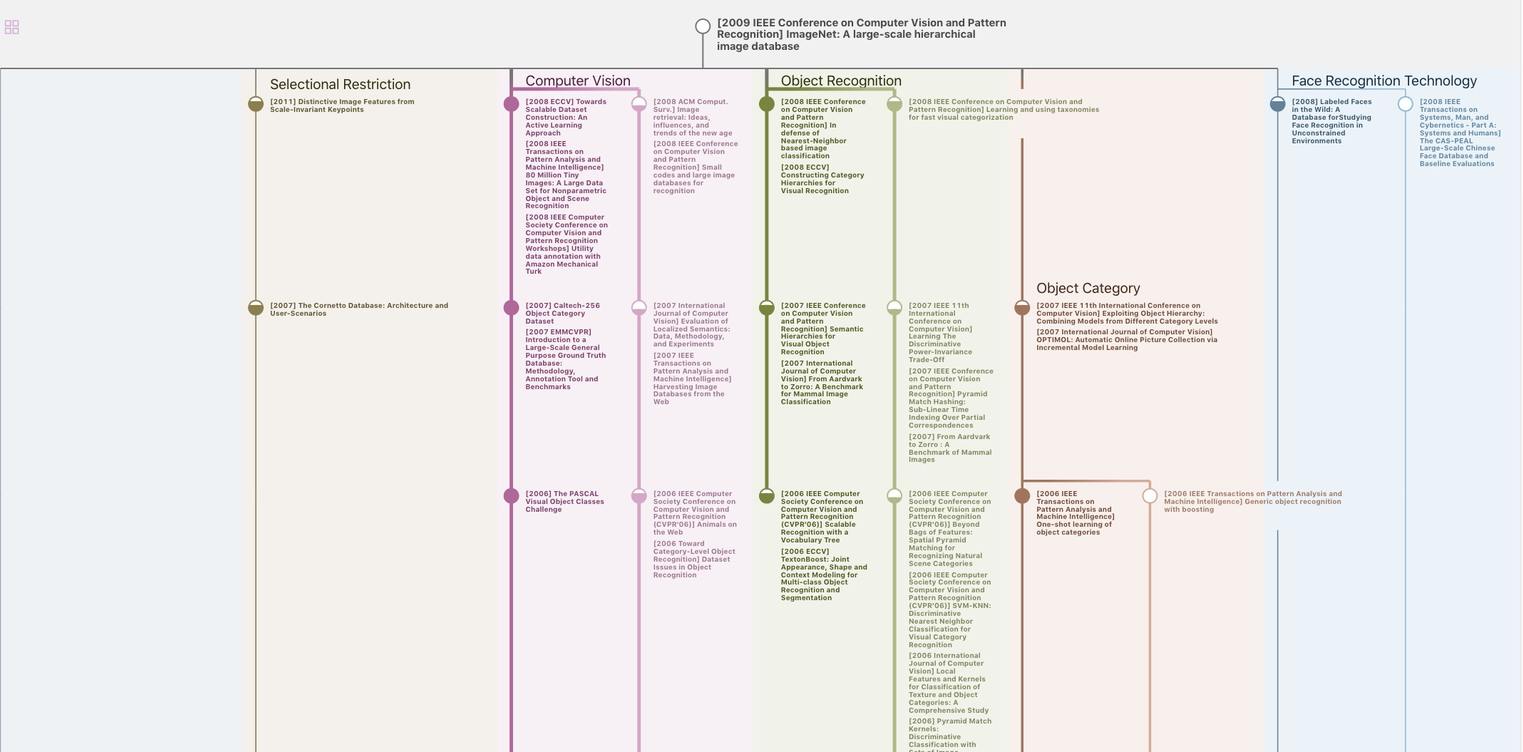
生成溯源树,研究论文发展脉络
Chat Paper
正在生成论文摘要