A Multi-Stage Automated Online Network Data Stream Analytics Framework for IIoT Systems
IEEE Transactions on Industrial Informatics(2023)
Abstract
Industry 5.0 aims at maximizing the collaboration between humans and machines. Machines are capable of automating repetitive jobs, while humans handle creative tasks. As a critical component of Industrial Internet of Things (IIoT) systems for service delivery, network data stream analytics often encounter concept drift issues due to dynamic IIoT environments, causing performance degradation and automation difficulties. In this article, we propose a novel multistage automated network analytics framework for concept drift adaptation in IIoT systems, consisting of dynamic data preprocessing, the proposed drift-based dynamic feature selection method, dynamic model learning and selection, and the proposed window-based weighted probability averaging ensemble model. It is a complete automated data stream analytics framework that enables automatic, effective, and efficient data analytics for IIoT systems in Industry 5.0. Experimental results on two public IoT datasets demonstrate that the proposed framework outperforms state-of-the-art methods for IIoT data stream analytics.
MoreTranslated text
Key words
Automated data analytics,concept drift,data streams,ensemble learning,Internet of Things (IoT),online machine learning
AI Read Science
Must-Reading Tree
Example
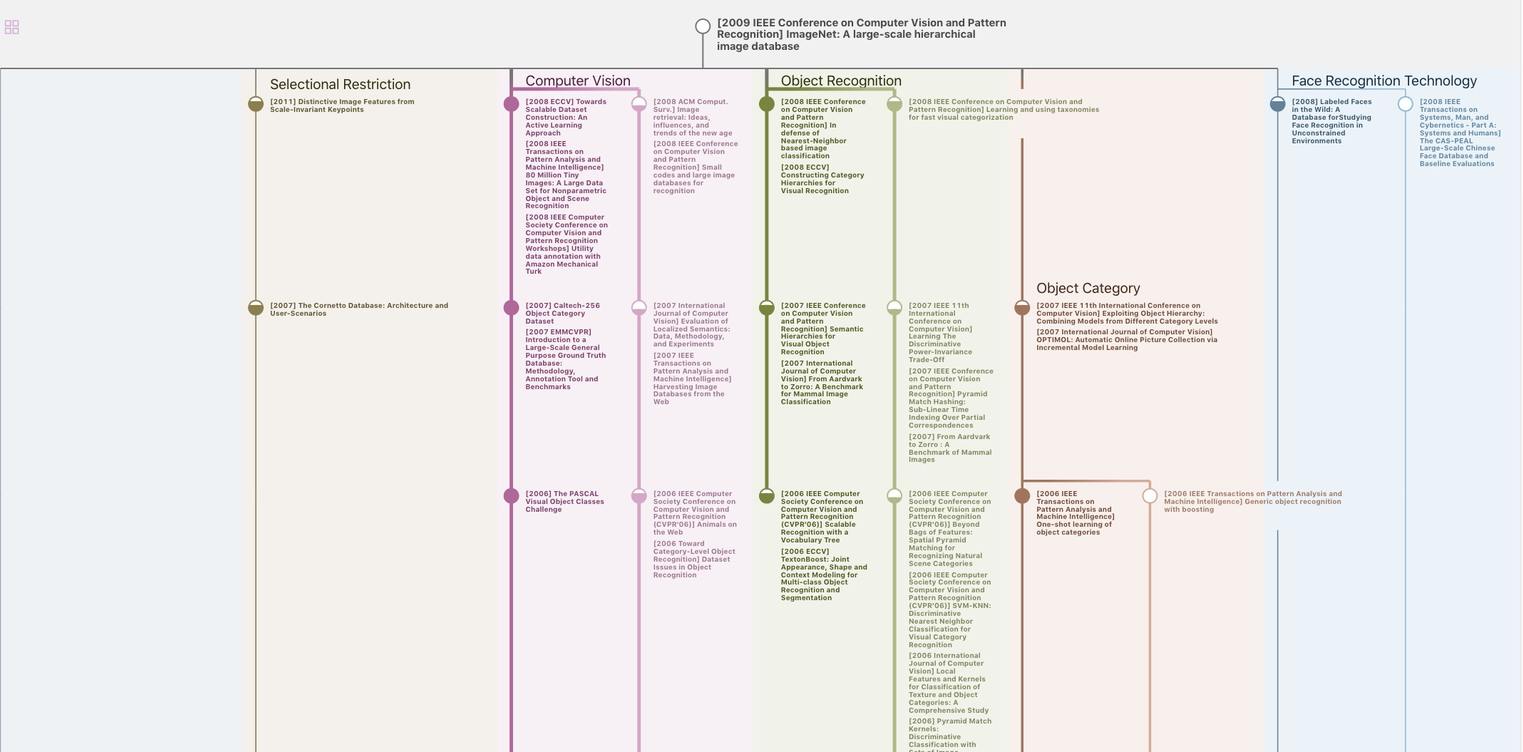
Generate MRT to find the research sequence of this paper
Chat Paper
Summary is being generated by the instructions you defined