Large-Scale Distributed Training of Transformers for Chemical Fingerprinting
JOURNAL OF CHEMICAL INFORMATION AND MODELING(2022)
摘要
Transformer models have become a popular choice for various machine learning tasks due to their often outstanding performance. Recently, transformers have been used in chemistry for classifying reactions, reaction prediction, physiochemical property prediction, and more. These models require huge amounts of data and localized compute to train effectively. In this work, we demonstrate that these models can successfully be trained for chemical problems in a distributed manner across many computers-a more common scenario for chemistry institutions. We introduce MFBERT: Molecular Fingerprints through Bidirectional Encoder Representations from Transformers. We use distributed computing to pre-train a transformer model on one of the largest aggregate datasets in chemical literature and achieve state-of-the-art scores on a virtual screening benchmark for molecular fingerprints. We then fine-tune our model on smaller, more specific datasets to generate more targeted fingerprints and assess their quality. We utilize a Sentence-Piece tokenization model, where the whole procedure from raw molecular representation to molecular fingerprints becomes data-driven, with no explicit tokenization rules.
更多查看译文
关键词
chemical fingerprinting,transformers,large-scale
AI 理解论文
溯源树
样例
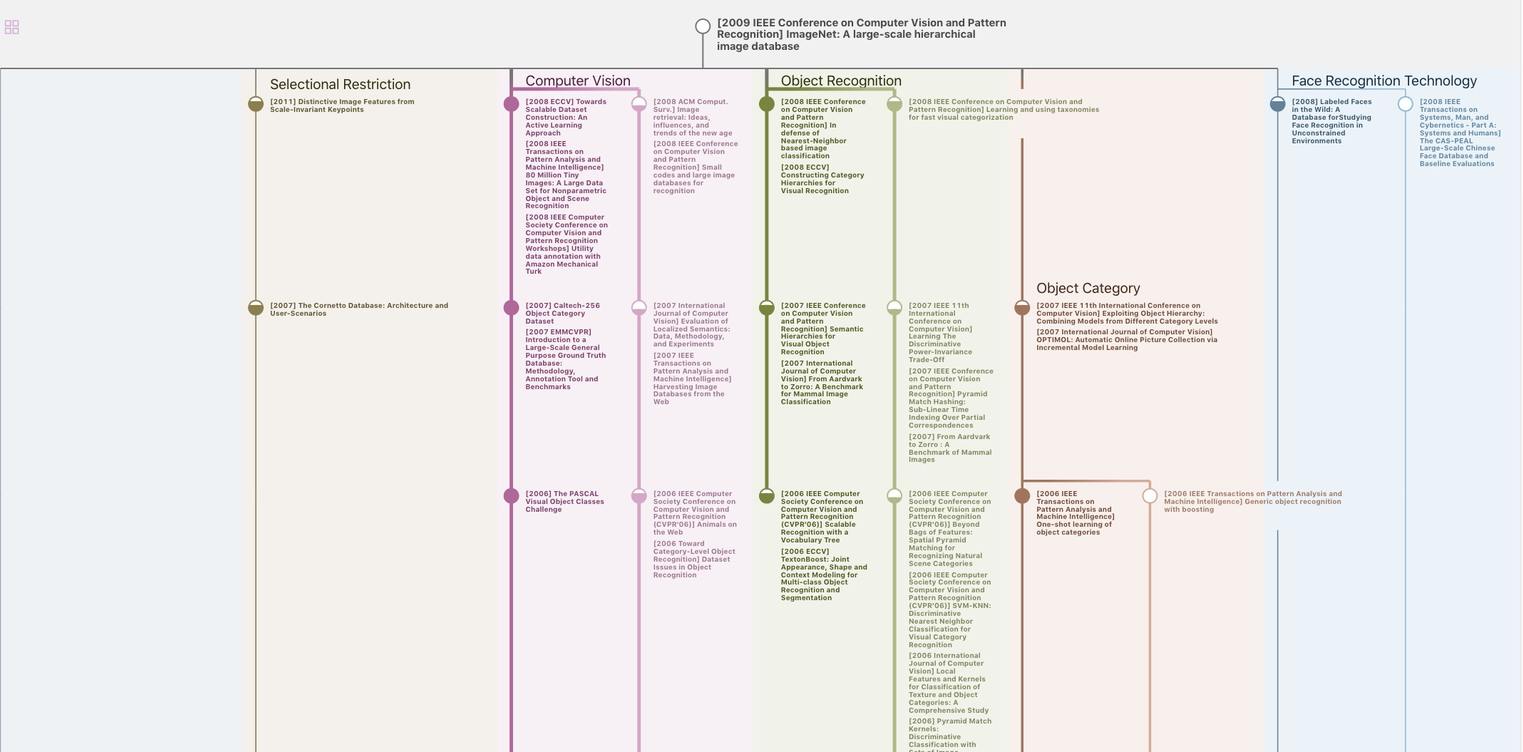
生成溯源树,研究论文发展脉络
Chat Paper
正在生成论文摘要