Measuring the Impact of Data Augmentation Techniques in Lung Radiograph Classification Using a Fractional Factorial Design: A Covid-19 Case Study
2022 IEEE COLOMBIAN CONFERENCE ON APPLICATIONS OF COMPUTATIONAL INTELLIGENCE (COLCACI 2022)(2022)
Abstract
Convolutional neural networks (CNNs) have become dominant in various computer vision tasks, obtaining state-of-the-art results in medical image analysis. Nevertheless, CNNs require large datasets to achieve high performance, which might not always be available in medical settings. Hence, different data augmentation strategies have been proposed to synthetically increase the size and diversity of a dataset. According to the state of the art, the relationship between data augmentation operations and the classification accuracy of a neural network has not been fully explored. In this work, the effect that basic augmentation techniques have in the detection of COVID-19 on chest X-Ray images is analyzed using a 2 (7−1) fractional factorial experimental design. The experimental results show that zoom in and height shift operations have a significant positive effect on the accuracy, while horizontal flip operation hinders the performance. Moreover, by applying a cube plot analysis, the data augmentation operations and values that maximize the accuracy of the CNN are found. A 97% accuracy, 93% precision, and 97.7% recall scores are attained on a publicly available COVID-19 dataset using these data augmentation operations.
MoreTranslated text
Key words
Medical Image Classification,COVID-19 Detection,Convolutional Neural Networks,Image Data Augmentation,Design of Experiments,Fractional Factorial Design
AI Read Science
Must-Reading Tree
Example
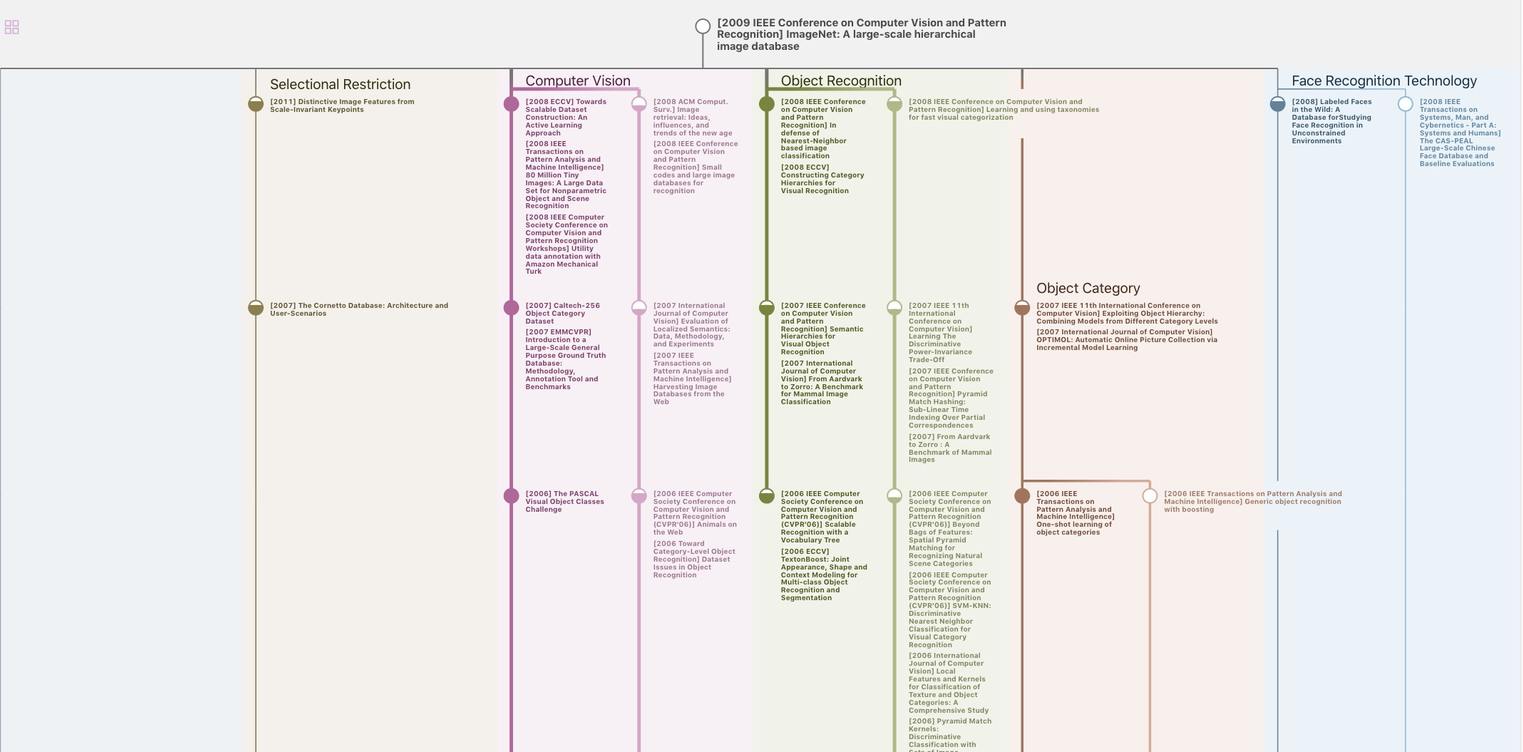
Generate MRT to find the research sequence of this paper
Chat Paper
Summary is being generated by the instructions you defined