Robust correlation filter tracking based on response map analysis network
Signal Processing: Image Communication(2022)
摘要
Conventional discriminative-correlation-filter-based (DCF-based) visual tracking methods always update model at a fixed frequency and learning rate. Without evaluating the tracking confidence scores, the response map generated by filter is the only evidence for locating. Thus, most of the existing DCF-based methods suffer from the model contamination caused by drastic appearance variations, which leads to tracking drift even failure. And excessively frequent update will increase the computational redundancy and risk of over-fitting. In addition, these methods cannot recover target from heavy occlusion neither. Based on the observation that the shape of response maps reflects the matching degree between filter and target, we design and train a small-scale binary network named as response map analysis network (RAN) to evaluate the confidence scores of filters. Further, we propose to learn multiple filters to exploit different kinds of features, and adaptively adjust the update parameters according to the corresponding confidence scores. Moreover, we build a simple occlusion event model to detect heavy occlusion and recover target. Extensive experimental results validate the effectiveness of RAN and demonstrate that the proposed tracker performs favorably against other state-of-the-art (SOTA) DCF-based trackers in terms of precision, overlap rate and efficiency.
更多查看译文
关键词
Visual tracking,Discriminative correlation filter,Tracking confidence,Neuron network
AI 理解论文
溯源树
样例
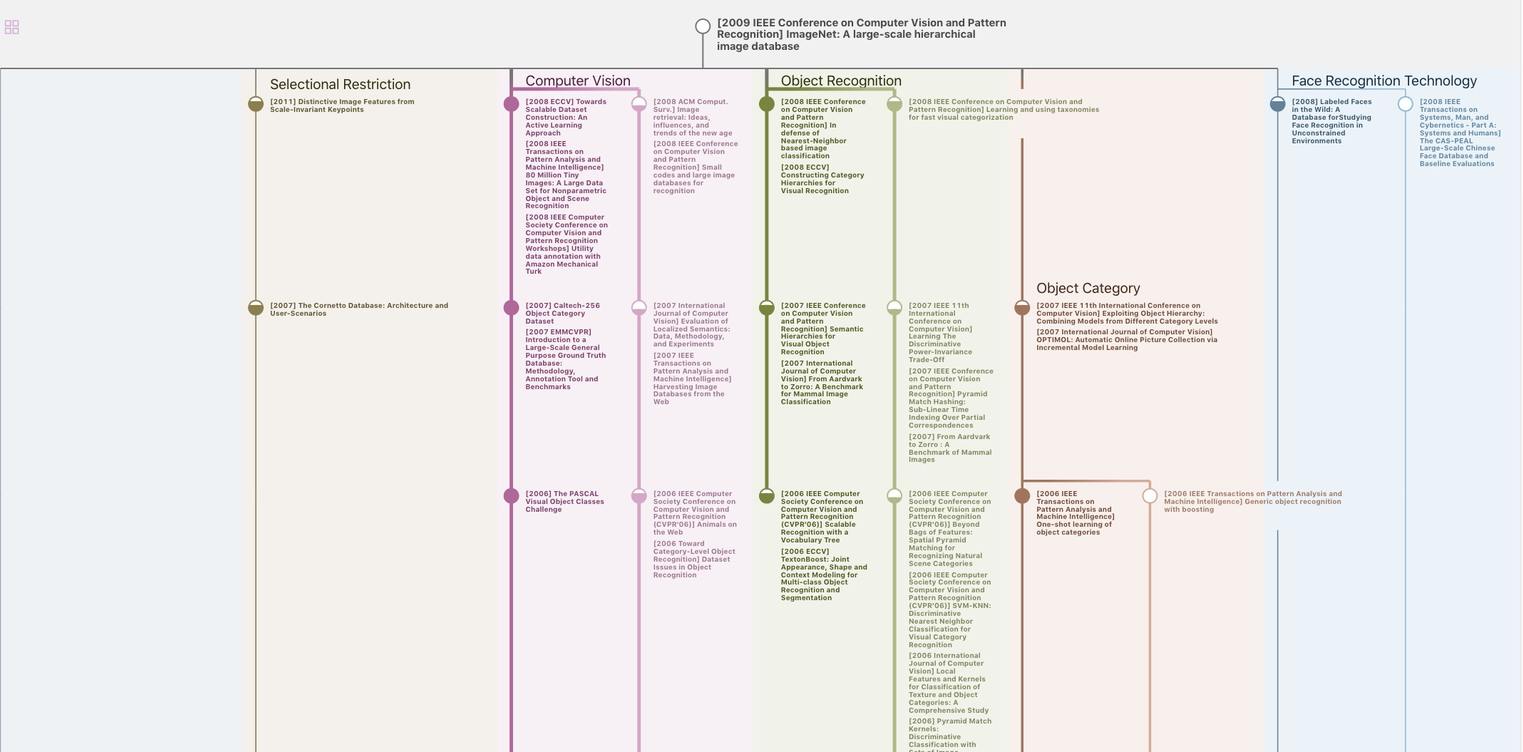
生成溯源树,研究论文发展脉络
Chat Paper
正在生成论文摘要