Real-Time Detection of Cyber-Attacks in Modern Power Grids with Uncertainty using Deep Learning
2022 International Conference on Smart Energy Systems and Technologies (SEST)(2022)
摘要
The smart grid, which is critical for developing smart cities, has a tool called state estimation (SE), which enables operators to monitor the system’s stability. While the SE result is significant for future control operations, its reliability is strongly dependent on the data integrity of the information obtained from the dispersed measuring devices. However, the dependence on communication technology renders smart grids vulnerable to advanced data integrity attacks, presenting significant concerns to the overall reliability of SE. Among these attacks, the false data-injection attack (FDIA) is gaining popularity owing to its potential to disrupt network operations without being discovered by bad data detection (BDD) methods. Existing countermeasures are limited in their ability to cope with sudden physical changes in the smart grid, such as line outages, due to their development for a certain system specifications. Therefore, the purpose of this paper is to develop an attack detection scheme to find cyber-attacks in smart grids that are influenced by contingencies. In particular, a detection framework based on long short-term memory (LSTM) is proposed to discern electrical topology change in smart grids from real-time FDIAs. Results show that the developed framework surpasses the present techniques.
更多查看译文
关键词
Cybersecurity,deep learning,smart grid,topology changes
AI 理解论文
溯源树
样例
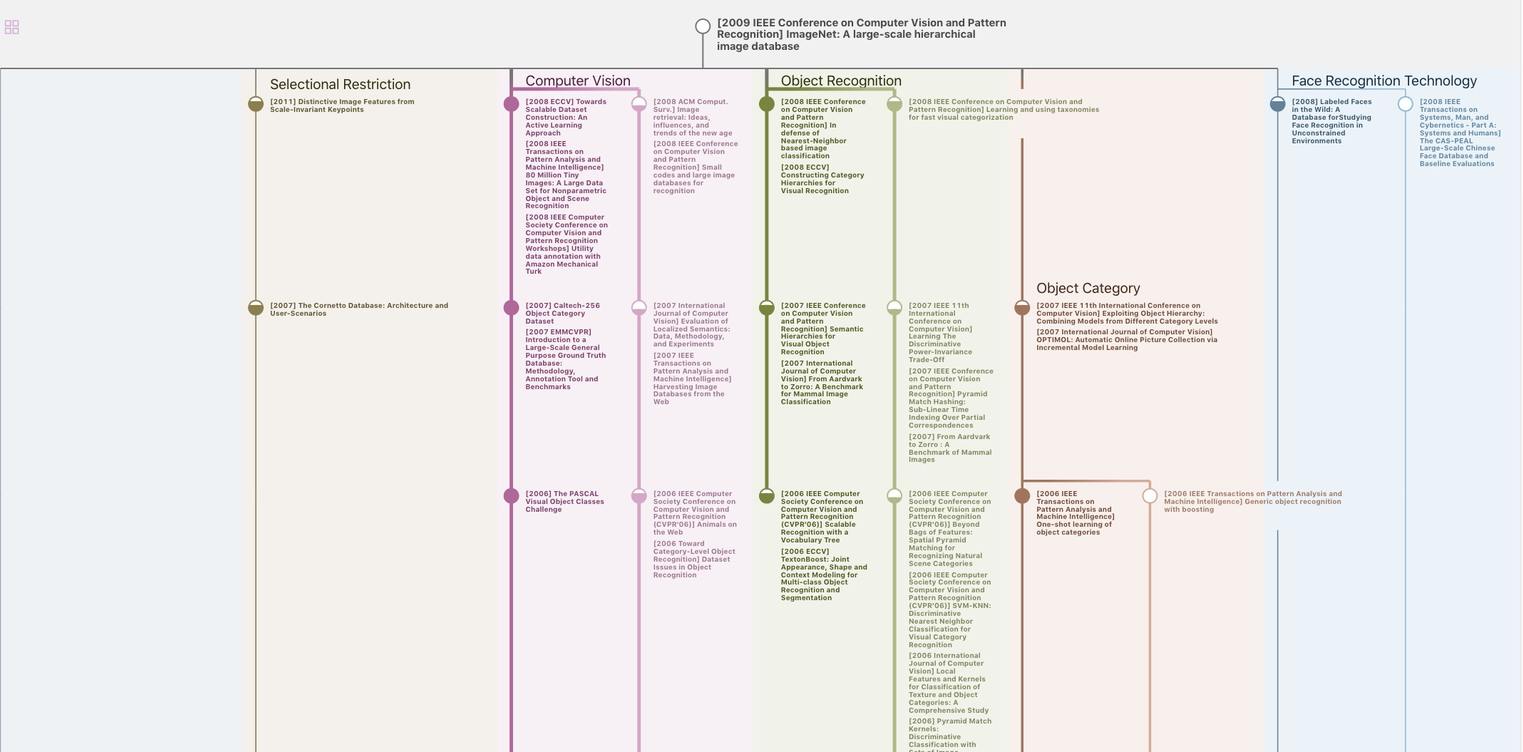
生成溯源树,研究论文发展脉络
Chat Paper
正在生成论文摘要