Deep Learning-Based Facial Appearance Simulation Driven by Surgically Planned Craniomaxillofacial Bony Movement
MEDICAL IMAGE COMPUTING AND COMPUTER ASSISTED INTERVENTION, MICCAI 2022, PT VII(2022)
摘要
Simulating facial appearance change following bony movement is a critical step in orthognathic surgical planning for patients with jaw deformities. Conventional biomechanics-based methods such as the finite-element method (FEM) are labor intensive and computationally inefficient. Deep learning-based approaches can be promising alternatives due to their high computational efficiency and strong modeling capability. However, the existing deep learning-based method ignores the physical correspondence between facial soft tissue and bony segments and thus is significantly less accurate compared to FEM. In this work, we propose an Attentive Correspondence assisted Movement Transformation network (ACMT-Net) to estimate the facial appearance by transforming the bony movement to facial soft tissue through a point-to-point attentive correspondence matrix. Experimental results on patients with jaw deformity show that our proposed method can achieve comparable facial change prediction accuracy compared with the state-of-the-art FEM-based approach with significantly improved computational efficiency.
更多查看译文
关键词
Deep learning, Surgical planning, Simulation, Correspondence assisted movement transformation, Cross point-set attention
AI 理解论文
溯源树
样例
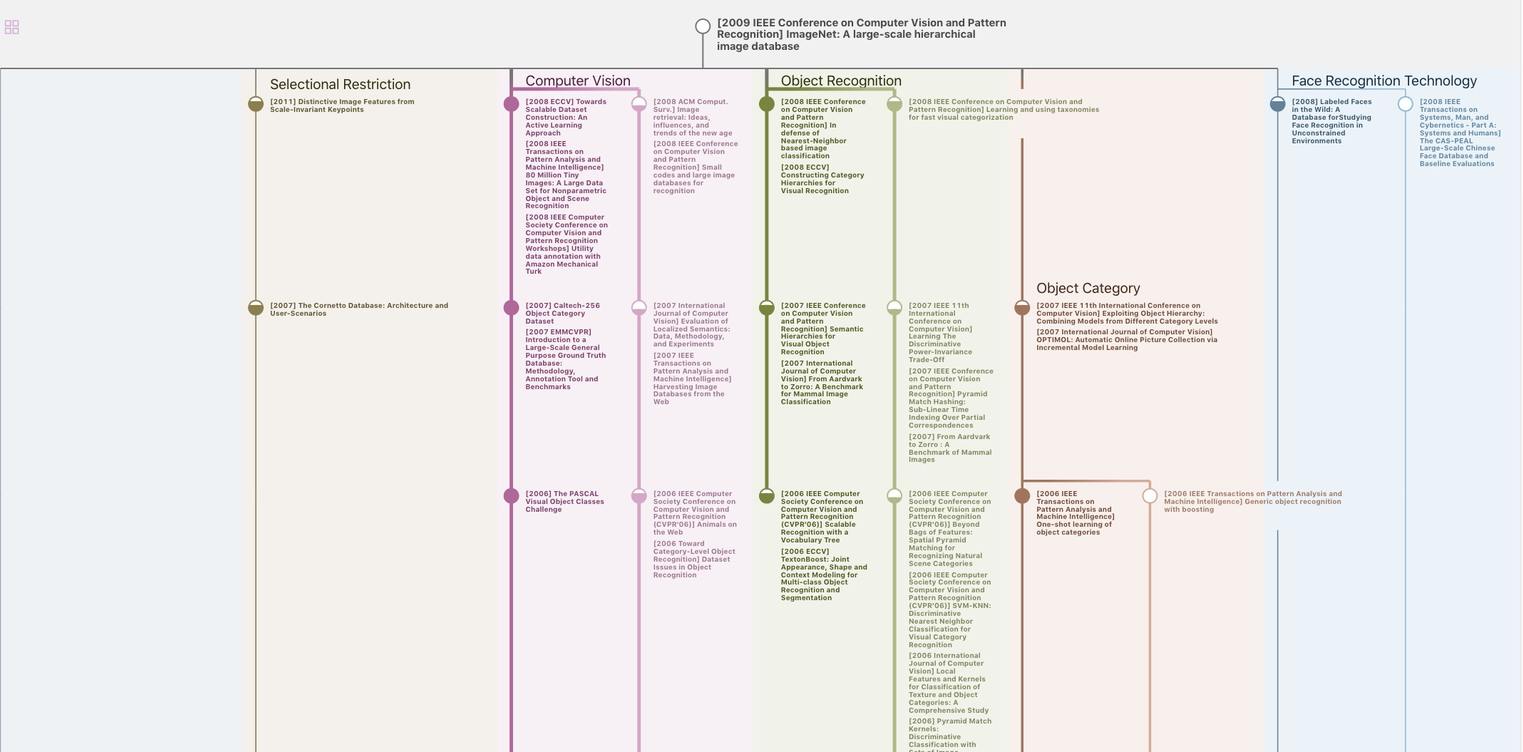
生成溯源树,研究论文发展脉络
Chat Paper
正在生成论文摘要